Your voice assistant might be racist for one troubling reason — study
“Not everyone can take advantage of these powerful new tools.”
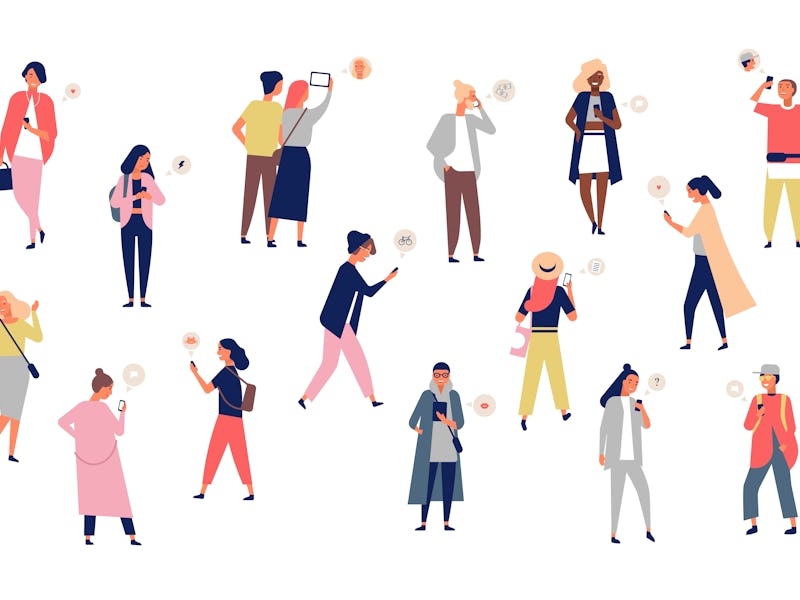
Not only might your smart assistant be listening to you, it is also probably racist for this one disturbing reason.
Automated speech recognition systems, like the ones operating voice technologies like Amazon Transcribe and Apple's Siri, understand black people less than they understand white people.
On average, even state-of-the-art automated speech recognition systems made nearly twice as many mistakes for black speakers compared to white speakers in every case, according to a new study published Monday in the journal Proceedings of the National Academy of Sciences.
Biased listeners everywhere
Automated speech recognition systems have all sorts of applications, including powering virtual assistants like your Google Home, transcription services, hands-free computing for people who have hearing problems or motor impairments, and more.
They are powered by machine-learning algorithms that take what you say to them and convert it into text commands that vary according to the text and acoustic data they’ve been trained on. These systems are now near-ubiquitous in technology, and as deep learning improves, so do their abilities..
"The direction of these findings is likely unsurprising."
But no matter the pace of advancements, they also seem to be inherently racially biased. This is hardly news: Understanding accents is a problem in court transcriptions, as the Marshall Project reports. And Google Home and Amazon Echo have a hard time understanding accents, according to reporting by the Washington Post.
“The direction of these findings is likely unsurprising; our study is the latest in a long line of similar research,” Allison Koenecke, a graduate student at Stanford University and author on the new study, tells Inverse.
“To us, the more surprising finding was the magnitude and consistency of disparities found across ASR providers.”
There is an easy fix, however: Diversify the data you train these systems on, and the systems may become more open to diversity themselves.
“Our paper suggests that ASR providers should use more diverse data to train their models,” Koenecke says. “By incorporating more diverse training data in ASR acoustic models, we would expect a decrease in the racial gap.”
Who is listening?
In the new study, Koenecke and colleagues from Stanford University and Georgetown University looked at how well speech recognition systems powered by Amazon, Apple, Google, IBM, and Microsoft transcribed interviews with black and white people.
They fed the systems 19.8 hours of audio made up of 2,141 different snippets from interviews with 42 white speakers and 2,141 different snippets from interviews with 73 black speakers. It is the largest study of its kind to date.
Approximately 44 percent of snippets were of male speakers, and the average age was around 45 years old. The interviews came from two datasets: For black individuals who speak African American Vernacular English, they came from the Corpus of Regional African American Language, and for white speakers, they were interviews with people in Sacramento and Humboldt County, collected by Voices of California.
The researchers then compared how many mistakes the machines made for each voice by computing average word error rates.
They also hand-coded a random sample of black speakers to compare the specific features and characteristics of their use of African American Vernacular English.
The average WER across ASR services is 0.35 for audio snippets of black speakers, as opposed to 0.19 for snippets of white speakers. The maximum SE among the 10 WER values displayed (across black and white speakers and across ASR services) is 0.005.
“For every hundred words, the ASR systems made about 19 errors for white speakers compared to 35 errors for black speakers, or roughly twice as many errors for black speakers than for whites,” Koenecke says.
Overall, the average error rate was 0.35 per word for black speakers, but only 0.19 for white speakers, despite variation in transcript quality across all systems.
Apple was the most biased, according to the data, with error rates of respectively 0.45 versus 0.23 between black speakers and white speakers.
The systems seem to perform particularly poorly for black men (error rate 0.41), compared to black women (error rate 0.30). But average error rates for white men and women were closer skewed.
Speaking while black
“Our findings are important because the reach of ASR systems goes far beyond, for example, your phone,” Koenecke says.
For example, doctors use speech-to-text software to create medical records about their patients, online educators rely on automated closed-captioning of their videos to reach hard-of-hearing audiences, and people with physical impairments depend on this assistive technology to control their computers, Koenecke says.
“Critically, our work shows that not everyone can take advantage of these powerful new tools,” she says.
The fault in these systems may actively harm African American communities, for example when such automatic systems are used by employers to evaluate candidates, or when criminal justice agencies transcribe courtroom proceedings.
"We hope that big tech companies can invest in collecting data ethically."
Although Koenecke doesn’t know exactly what data Amazon, Apple and Google are feeding their machine learning, they speculate that it’s probably mostly white Standard English speakers, as this is historically true of the data used for voice recognition.
“We hope that big tech companies can invest in collecting data ethically, and also be cognizant of the positive feedback loop arising from consumer product usage,” Koenecke says.
"We believe our work can serve as a baseline for ASR service providers as they aim to decrease the racial disparities."
She explains that if only white users are understood by their phone’s voice assistant, that’s the only data the phone will collect, and that skews the model on which future voice assistants are developed. It also cuts out entire sections of the population.
Everyone involved in the process of developing these systems, from the makers of speech recognition systems, to academic speech recognition researchers, and government sponsors of speech research, need to invest resources into making this an inclusive system, she says.
Data shouldn’t also just be collected on African American Vernacular English speech, but also other non-standard varieties of English.
“We believe our work can serve as a baseline for ASR service providers as they aim to decrease the racial disparities in their products in the coming years,” Koenecke says.
“We call on developers of speech recognition tools to regularly assess and publicly report their progress along this dimension.”
Abstract: Automated speech recognition (ASR) systems, which use sophisticated machine-learning algorithms to convert spoken language to text, have become increasingly widespread, powering popular virtual assistants, facilitating automated closed captioning, and enabling digital dictation platforms for health care. Over the last several years, the quality of these systems has dramatically improved, due both to advances in deep learning and to the collection of large-scale datasets used to train the systems. There is concern, however, that these tools do not work equally well for all subgroups of the population. Here, we examine the ability of five state-of-the-art ASR systems—developed by Amazon, Apple, Google, IBM, and Microsoft—to transcribe structured interviews conducted with 42 white speakers and 73 black speakers. In total, this corpus spans five US cities and consists of 19.8 h of audio matched on the age and gender of the speaker. We found that all five ASR systems exhibited substantial racial disparities, with an average word error rate (WER) of 0.35 for black speakers compared with 0.19 for white speakers. We trace these disparities to the underlying acoustic models used by the ASR systems as the race gap was equally large on a subset of identical phrases spoken by black and white individuals in our corpus. We conclude by proposing strategies—such as using more diverse training datasets that include African American Vernacular English—to reduce these performance differences and ensure speech recognition technology is inclusive.