In London, a huge, powerful computer is creating new chemistry
Computers will help us truly understand atoms.
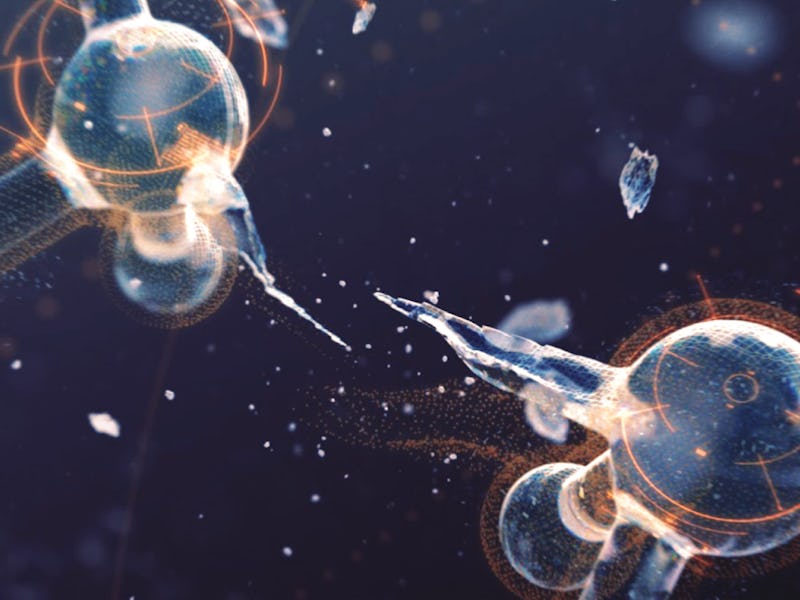
Whirling around inside our desks and under our fingernails are trillions of electrons orbiting around their atomic suns and creating all matter as we know it.
This subatomic ballet has been well known to physicists and chemists alike for over a hundred years, but being able to predict or mimic this science to create chemicals of our own has proven to be a much more difficult task. So why not design computers to do it for us?
At least, that’s the idea of Google DeepMind scientists who, in a new paper published Thursday in the journal Science, propose a neural network that can more accurately predict and recreate these electron patterns than ever before.
“Solving some of the major challenges of the 21st Century, such as producing clean electricity or developing high-temperature superconductors, will require us to design new materials with specific properties,” the authors write in a blog post about the work.
“To do this on a computer requires the simulation of electrons... we propose DM21, a neural network achieving state of the art accuracy on large parts of chemistry.”
The history — English physicist J.J. Thomson is credited with first discovering the electron in 1897 through experimentation with cathode-ray tubes — vacuum tubes full of electrons. Yet even though Thomson had discovered the electron, he and other scientists of the time had little to no understanding of how this particle actually moved about an atom.
Thomson believed that electrons were evenly distributed around a nucleus — like fruit in a Christmas pudding.
In 1904, Thomson proposed the so-called “plum pudding” model of the atom — where electrons were evenly distributed like pieces of fruit in a cake. Physicists have since discarded this idea for Erwin Schrödinger’s “electron-cloud” model, which uses quantum mechanics to predict which energy levels electrons are most likely to be found. This work earned Schrödinger a Nobel Prize in physics in 1933.
However, having a way to predict where electrons might be is not the same as tracking them directly. This is a question that Pierre Hohenberg and Walter Kohn would tackle thirty years after Schrödinger’s Nobel by proposing that instead of tracking individual electrons, we could instead use probability to calculate electron density and, through that, all their atomic interactions.
Thus, the “Density Functional Theory” (DFT) was born, for which Kohn won a Nobel Prize in chemistry in 1998.
This theory is the crux of what their neural network has innovated, the study’s first author and research scientist at Google DeepMind, James Kirkpatrick, tells Inverse.
What’s new — While DFT has been a huge step forward in calculating electron interactions, Kirkpatrick and colleagues write that it’s also plagued by estimation errors.
“Over the years, researchers have proposed many approximations to the exact functional with varying levels of accuracy,” the authors write in a blog post. “Despite their popularity, all of these approximations suffer from systematic errors because they fail to capture certain crucial mathematical properties of the exact functional.”
The neural network is designed to take in electron density maps and output interaction energies.
Some of these errors can even bend — or break — the laws of atomic physics as we know it, such as breaking spin symmetry.
The solution that Kirkpatrick and colleagues propose is a neural network that can pick up on behavior patterns in electron movement better human minds alone. Using these improved calculations could help scientists create brand new chemistry.
What they did — Instead of having DeepMind sit in on quantum chemistry lectures, Kirkpatrick says they trained the network using the charge density of a molecule as input and outputting its energy.
“In simple terms, it is trained by showing it a number of examples of charge densities whose energies we know, either from precise calculations or from known mathematical constraints on the exact functional,” Kirkpatrick says. “Training then consists of adapting the network weights to reproduce this mapping on the dataset.”
The team report in their paper that the network has already been able to accurately model complex systems like hydrogen chains, charged DNA base pairs, and orbital transition states.
What’s next — To bring this future more quickly to fruition, the team will make their source code open-access. The team writes that this work will bring quantum chemistry into the modern age of computer simulations and create everything from batteries to medicine in a brand new way.
“For years, computer simulations have played a central role in modern engineering, making it possible to provide reliable answers to questions like ‘will this bridge stay up?’ to ‘will this rocket make it into space?’” the team writes.
“As technology increasingly turns to the quantum scale to explore questions about materials, medicines, and catalysts, including those we’ve never seen or even imagined, deep learning shows promise to accurately simulate matter at this quantum mechanical.”
Abstract: Density functional theory describes matter at the quantum level, but all popular approximations suffer from systematic errors that arise from the violation of mathematical properties of the exact functional. We overcame this fundamental limitation by training a neural network on molecular data and on fictitious systems with fractional charge and spin. The resulting functional, DM21 (DeepMind 21), correctly describes typical examples of artificial charge delocalization and strong correlation and performs better than traditional functionals on thorough benchmarks for main-group atoms and molecules. DM21 accurately models complex systems such as hydrogen chains, charged DNA base pairs, and diradical transition states. More crucially for the field, because our methodology relies on data and constraints, which are continually improving, it represents a viable pathway toward the exact universal functional.