‘Super-human’ A.I. could solve one of Tesla's biggest problems
Electric vehicles have a serious accessibility issue.
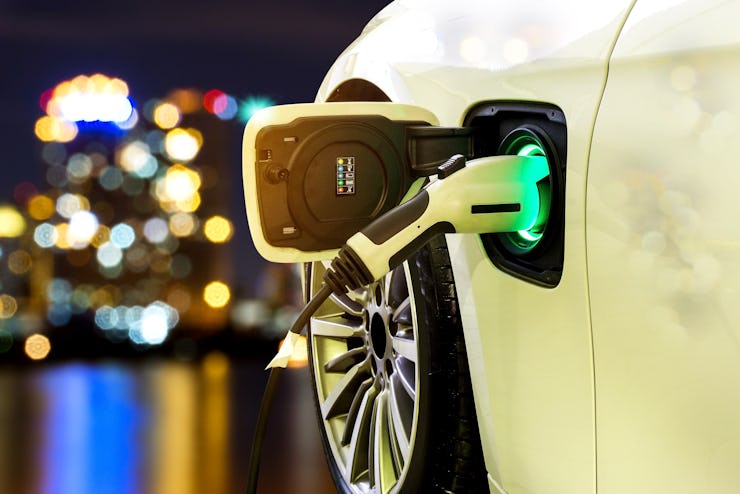
Electric vehicles are hailed as one of the easiest — and most desirable — ways to curb carbon emissions, fast. But these cars' roll out onto the roads suffers from a challenging logistics issue. Unlike the ubiquity of the gas station as a fixture in even the most deserted landscapes in America, these vehicles simply can't find anywhere to charge.
Government rebates on green vehicles like Tesla's Model 3 and supposedly better charging infrastructure have helped popularize these vehicles in recent years (not to mention the star power of the people who lead these companies). The design value of these cars has also made leaps and bounds — there is no denying the Rivian SUV or the Tesla Model Y aren't attractive. But a new study pours a little cold water on the hype: There may be positive anecdotes of their utility, but there is no good way to assess how all this injected capital translates to real-world gains.
By designing an algorithm able to quickly and accurately parse more than 3 million EV charging station reviews, the researchers behind the study reveal hidden insights into the charging problem which could help build future infrastructure and ultimately alter one of the United States' most enduring love affairs — the desire to pump the gas and hit the open road.
One of the defining landmarks in the United States, gas stations may one day be replaced by charging stations.
Why it matters — EV charging stations are fundamental infrastructure to bring Tesla and other electric cars to the masses, but they are also a major industry in their own right. The market in EV charging stations is estimated to reach over $27 billion in value by 2027. Aside from the money at play, using A.I. to better understand users' reactions to current infrastructure can also have a more profound affect on who drives electric cars in the first place. Currently the preserve of the wealthy, this study could help electric car companies better reach underserved areas, and create a seamless service ready to rival traditional gas stations.
The research was published Friday in the journal Patterns.
Here's the background — When it comes to checking in on how government projects to roll out EVs are going, there are typically two main approaches: surveys and simulations. These can be pricey, and they also suffer from inherent limits and biases, such as only capturing certain demographics, or geographic areas in their scope.
Using a new A.I. algorithm, researchers hope to tap into over 3 million user insights about EV charging infrastructure.
But thanks to third-party apps designed to rate charging stations throughout the country, there are over 3 million reviews of EV charging stations. Much like a Yelp for EV, these reviews are filled with the inner feelings, secret joys, and significant woes of EV users.
Omar Asensio is author on the study and assistant professor in the School of Public Policy at the Georgia Institute of Technology. He says the problem with these reviews is there is no good way to parse their data in a useful way.
"We're spending billions of both public and private dollars on electric vehicle infrastructure," Asensio says in a statement accompanying the research.
"But we really don't have a good understanding of how well these investments are serving the public and public interest."
Part of the problem is there are so many different topics these reviews could be addressing and having human beings sort through and categorize each one would be incredibly tedious. Instead, Asensio and colleagues set out to see if an A.I. could do it better. A deep learning algorithm to be exact.
What they did — The insights the team were most interested in fell into eight different categories:
- Functionality
- Range anxiety
- Availability
- Cost
- User interactions
- Location
- Service Time
- Dealership
The A.I. also had to be trained to recognize a variety of review presentations — from paragraphs, to three words, or even just emojis.
With this breakdown in mind, the researchers then trained the A.I. on expert-curated samples of these reviews, and then they unleashed the A.I. on the entire library.
"Opportunities abound."
What they discovered — Compared to human experts, the researchers found that their A.I. was accurate 91 percent of the time at correctly identifying the eight key topics in the EV charging station reviews. In some instances, the A.I. did a better job than the humans — it was able to correctly label review data which the human experts had missed.
The researchers say their method may be evidence of "super-human classification."
"It's no longer 'can the A.I. do as good as human?'" Asensio says. "In some cases, the A.I. exceeded the performance of human experts."
The A.I. also identified several trends in the data — including a number of underserved regions throughout the U.S.
The South and Northeast tended to have more availability issues in large metropolitan areas, but the A.I. noticed EV users in the Midwest, West, and Hawaii tended to run into availability problems in so-called "micropolitan" cities, with under 50,000 residents. The authors say their data could help highlight underserved states in need of further infrastructure.
What's next — For the time being, the study is only a proof-of-concept, but the researchers write they're excited for what the data mean for not only better understanding how Americans' attitudes towards EV infrastructure is changing. The method could also help parse other multi-faceted data sets related to infrastructure.
"Further applications of methods that we propose, particularly those that integrate artificial intelligence with real-time data and spatial analysis, can greatly enhance new ways of thinking about infrastructure management as well as economic and policy analysis," write the authors in the study.
"Other opportunities abound."
Abstract: The transportation sector is a major contributor to greenhouse gas (GHG) emissions and is a driver of adverse health effects globally. Increasingly, government policies have promoted the adoption of electric vehicles (EVs) as a solution to mitigate GHG emissions. However, government analysts have failed to fully utilize consumer data in decisions related to charging infrastructure. This is because a large share of EV data is unstructured text, which presents challenges for data discovery. In this article, we deploy advances in transformer-based deep learning to discover topics of attention in a nationally representative sample of user reviews. We report classification accuracies greater than 91% (F1 scores of 0.83), outperforming previously leading algorithms in this domain. We describe applications of these deep learning models for public policy analysis and large-scale implementation. This capability can boost intelligence for the EV charging market, which is expected to grow to US $27.6 billion by 2027.