Climate change threatens crops. A.I. could help save them.
A new kind of A.I. could help us increase agricultural yields.
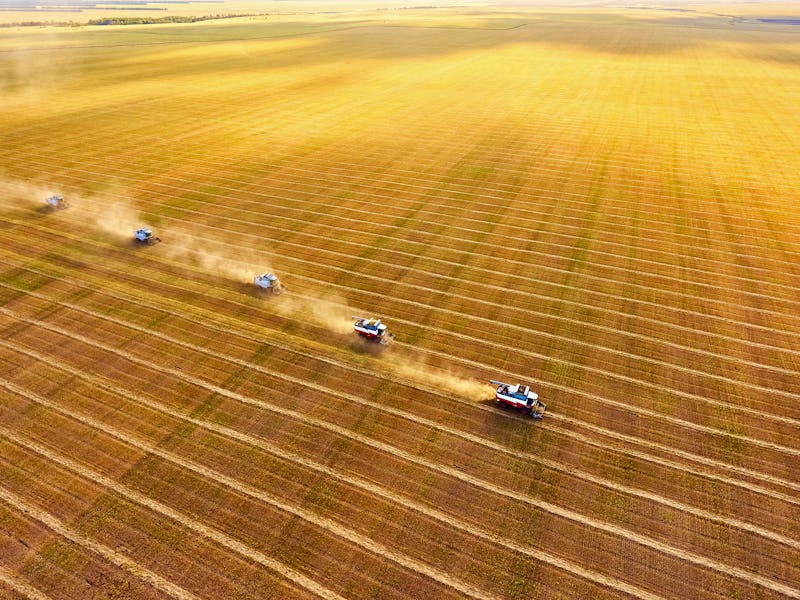
Many of today's farms don't look like the farms of the past. Robots and drones are increasingly being used to help grow, harvest and process the food we eat, and artificial intelligence can help farmers gain insight into how they can maximize their productivity. A new study that was published on Thursday describes an artificial intelligence system that could help farmers monitor what they're growing and increase yields in new ways.
The study, which was published in the journal Computers and Electronics in Agriculture, was done by a research group at the University of Illinois. It describes what they call a Convolutional Neural Network (CNN) that can be fed data on soil, elevation, nitrogen application rate and seed rate to predict what a farmer's fields will yield and help them increase their yields.
Nicolas Martin, an assistant professor of crop sciences and co-author of the study, said in a statement that this is different from how A.I. has been used in agriculture in the past.
"We're trying to change how people run agronomic research. Instead of establishing a small field plot, running statistics, and publishing the means, what we're trying to do involves the farmer far more directly. We are running experiments with farmers' machinery in their own fields," Martin said. "We can detect site-specific responses to different inputs. And we can see whether there's a response in different parts of the field."
Martin explained that the CNN—not to be confused with the news network—uses deep learning to analyze topographic variables, soil electroconductivity, nitrogen and seed rate treatments to predict yield. The A.I. system was created using data from the Data Intensive Farm Management project, which applies seeds and nitrogen fertilizer to over 200 fields in the Midwest, Argentina, Brazil and South Africa.
Using machine learning, the researchers were able to put together the different pieces of data that were fed into this system and identify patterns. These patterns can then be analyzed to learn which combination of factors would produce a certain yield.
"We don't really know what is causing differences in yield responses to inputs across a field. Sometimes people have an idea that a certain spot should respond really strongly to nitrogen and it doesn't, or vice versa. The CNN can pick up on hidden patterns that may be causing a response," Martin said. "And when we compared several methods, we found out that the CNN was working very well to explain yield variation."
See also: Researchers Unearth a New Tool for Fighting Climate Change: Better Soil
With this kind of A.I., farmers could feed data they collect in their fields into it and determine what they might need to change to increase their yield. If they discover nitrogen levels are too low, for example, they could add more fertilizer to their fields.
Considering climate change is going to not only reduce crop yields by altering temperatures and precipitation patterns but also change what people grow in many areas, being able to use A.I. to analyze your fields and figure out how to produce the most food you can will be very useful. The skills that have been passed down for generations might not always work when the climate isn't the same as it was years ago and you need to adapt to it.
Abstract
Predicting crop yield response to management and environmental variables is a crucial step towards nutrient management optimization. With the increase in the amount of data generated by agricultural machinery, more sophisticated models are necessary to get full advantage of such data. In this work, we propose a Convolutional Neural Network (CNN) to capture relevant spatial structures of different attributes and combine them to model yield response to nutrient and seed rate management. Nine on-farm experiments on corn fields are used to construct a suitable dataset to train and test the CNN model. Four architectures combining input attributes at different stages in the network are evaluated and compared to the most commonly used predictive models. Results show a reduction in the test dataset RMSE up to 68% when compared to multiple linear regression and up to 29% when compared to a random forest. We also demonstrate that higher variability associated with the spatial structure of the data takes the most advantage of this framework.