DeepMind, A.I. firm behind AlphaGo, has just conquered another classic game
The A.I. achieved the benchmark as all three of the game's races.
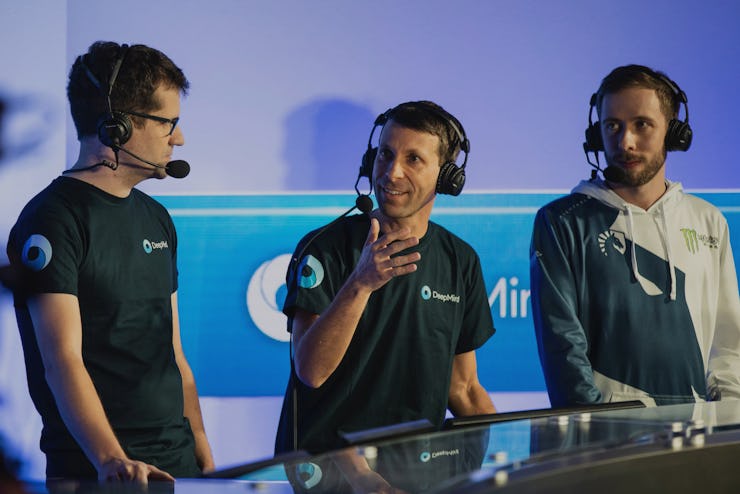
Humans are good at creating games, but in recent years artificial intelligence has shown that it might be much better at winning them. New research has demonstrated this once again, this time in the realm of an online strategy game called Starcraft II, in which an A.I. successfully beat 99.8 percent of human opponents it faced in the online game to become a Grandmaster.
The research was published Wednesday in the journal Nature and describes an approach in which researchers trained an A.I. called AlphaStar to successfully compete anonymously on a public European server for the online strategy game Starcraft II. The system was created in part by DeepMind, the London-based company owned by Google parent company Alphabet, which has previously designed A.I.s that could beat humans at the game Go as well as A.I. designed to drive genomic research and improve healthcare systems.
In this case, not only did AlphaStar beat the human opponents it played against in these quick games, but it did so as all three of the game’s races — Protoss, Terran, and Zerg. Despite each race having slightly different gameplay, AlphaStar was able to achieve Grandmaster status in all three. The system was trained using a mix of neural networks, reinforcement learning, multi-agent learning, and imitation learning.
“”
If an A.I. beating humans at a game of our own creation sounds familiar, that’s because such successes have become common benchmarks against which to judge the problem-solving abilities and flexibility of an artificial intelligence. A.I.s have previously beaten humans at games like chess, Go and even the gameshow Jeopardy.
But what separates those achievements from that of AlphaStar’s in Starcraft II is that the game, released in 2010, is a real-time strategy game with tens of thousands of possible moves (10^26 or nearly an octillion, to be exact) and a partially obscured playing field that can only be fully seen through scouting. The combination of these constraints has helped Starcraft II quickly rise to renown as a particularly challenging test for A.I.s. The game even has its own A.I. leaderboard.
For the AlphaStar team, besting human players took a combination of tried-and-true approaches as well as new innovations, namely a new way in which the A.I. learned self-play, a form of reinforcement learning. Self-play, in a nutshell, is a process in which an A.I. competes against iterations of itself in order to test and improve its approach to gameplay. However, the authors write, a self-play approach to learning can suffer from a shortcoming in which the A.I. forgets what it has learned from previous iterations of the play and is continually chasing its own tail to keep up with repeated moves.
In a blog post about the research, the authors give the example of rock-paper-scissors to illustrate the pitfall.
“Forgetting can create a cycle of an agent “chasing its tail”, and never converging or making real progress. For example, in the game rock-paper-scissors, an agent may currently prefer to play rock over other options. As self-play progresses, a new agent will then choose to switch to paper, as it wins against rock. Later, the agent will switch to scissors, and eventually back to rock, creating a cycle. Fictitious selfplay - playing against a mixture of all previous strategies - is one solution to cope with this challenge.”
Pictured above, AlphaStar holding its own in a round of *Starcraft II*.
In the case of AlphaStar and Starcraft II, this fictitious gameplay was achieved by mimicking a multiplayer gaming tactic: creating a league of players. A key feature of this approach which led to more robust gameplay, write the authors, is that designated “exploiter” agents in the league would identify and exploit weaknesses in the A.I.’s gameplay, forcing it to continually innovate its approach.
“Using this training method, the current League learns all its complex StarCraft II strategy in an end-to-end-fashion – as opposed to the earlier incarnation of our work, which stitched together agents produced by a variety of methods and algorithms,” write the authors in a blog post.
Using this approach to training, and real-world constraints (such as lag and limited action speed) as approved by a professional Starcraft II player, AlphaStar was able to best 99.8 percent of its human opponents and become Grandmaster in all three races.
In their paper, the authors write that this achievement has the potential to go far beyond just gaming and could be generalized and applied to other situations that require real-time, dynamic choices to be made with limited information, such as self-driving cars or personal assistants. These skills learned through AlphaStar would add to DeepMind’s already growing inventory of real-world applications for A.I., which currently includes skills such as weather prediction.
Together they prove once again that playing video games, at least in the pursuit of research, is far from a waste of time.
Read the abstract here:
Many real-world applications require artificial agents to compete and coordinate with other agents in complex environments. As a stepping stone to this goal, the domain of StarCraft has emerged by consensus as an important challenge for artificial intelligence research, owing to its iconic and enduring status among the most difficult professional esports and its relevance to the real world in terms of its raw complexity and multiagent challenges. Over the course of a decade and numerous competitions 1–3, the best results have been made possible by hand-crafting major elements of the system, simplifying important aspects of the game, or using superhuman capabilities 4. Even with these modifications, no previous system has come close to rivalling the skill of top players in the full game. We chose to address the challenge of StarCraft using general purpose learning methods that are in principle applicable to other complex domains: a multi-agent reinforcement learning algorithm that uses data from both human and agent games within a diverse league of continually adapting strategies and counterstrategies, each represented by deep neural networks5,6. We evaluated our agent, AlphaStar, in the full game of StarCraft II, through a series of online games against human players. AlphaStar was rated at Grandmaster level for all three StarCraft races and above 99.8% of officially ranked human players.