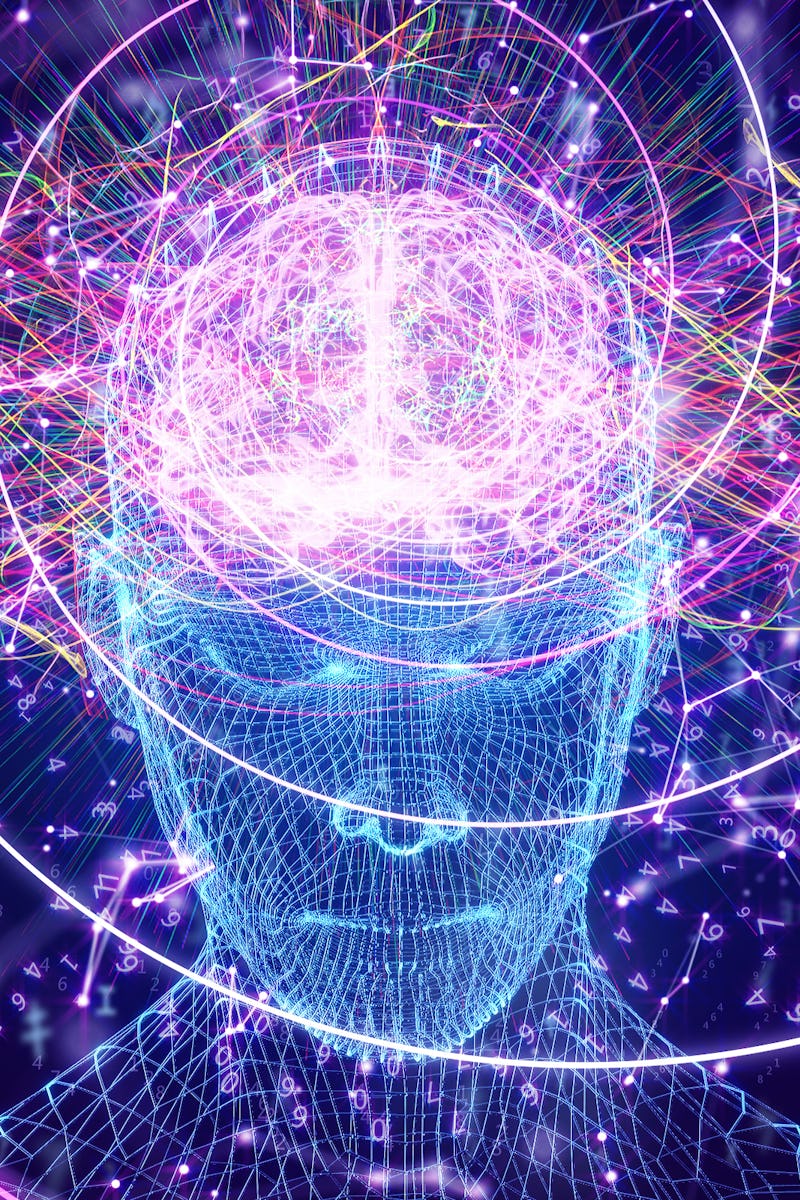
Shutterstock
"[Machines cannot...] be kind, resourceful, beautiful, friendly, have initiative, have a sense of humour, tell right from wrong, make mistakes, fall in love, enjoy strawberries and cream, make someone fall in love with it, learn from experience, use words properly, be the subject of its own thought, have as much diversity of behaviour as a man, do something really new."
Alan Turing, "Computing Machinery and Intelligence"
Scientists design "quantum brain" to revolutionize computing
Physicists have designed a new approach to machine learning that uses a quantum brain instead of algorithms to embed intelligence.
Computers have come a long way since Alan Turing postulated their limitations in his now-famous 1950 paper "Computing Machinery and Intelligence."
They may not be falling in love (though, having someone fall in love with them is up for debate), or enjoying a bowl of strawberries and fresh cream, but the limitations of these machines are slowly crumbling. In the age of machine learning, another one has bitten the dust: The ability to learn from experience.
Typically, this kind of intelligence is achieved through a leap-frog-like system of multiple computers and machine-learning algorithms, but in a new paper published Monday in the journal Nature Nanotechnology, a team of physicists propose a different method. They designed a computer capable of embedding basic intelligence into its hardware — by taking advantage of atoms' quantum spins.
Why it matters — This so-called "quantum brain" is an example of neuromorphic computing — computing systems designed to mimic the biological structures of the brain. Ultimately, this technology could enable robots embedded with a tiny piece of computing hardware in their "brains" to make decisions on their own.
The big idea — Googling your favorite restaurant's hours may seem like an energy-free computation, but in the grand scheme these calculations add up to a substantial carbon footprint— and the magnitude of these computations is only growing.
Alexander Khajetoorians is first author of this new study and professor of scanning probe microscopy at Radboud University. He explains in a statement that, to meet computation's growing requirements and lower its energy-footprint, scientists need to seriously rethink how machines store and process information.
"This requires not only improvements to technology, but also fundamental research in game changing approaches," Khajetoorians says.
To tackle this, Khajetoorians and colleagues follow a streamlined approach to essentially embed a software "brain" into physical hardware instead.
"Our new idea of building a 'quantum brain' based on the quantum properties of materials could be the basis for a future solution for applications in artificial intelligence," Khajetoorians explains.
The future of artificial intelligence may be super-efficient, quantum brains.
What they did — If asked to picture a quantum brain, you might imagine a steampunk, metal version of our own brains — maybe with a few extra wires sticking out for good measure. But in reality, these kinds of neuromorphic technologies mimic the internal structure of our brains, including neurons and synapses, instead of their outer, aesthetic attributes.
Our brains make computations using signals sent via brains cells called neurons, translating incoming information into action. In their hardware version, the researchers mimicked this biological construction using cobalt atoms on a superconducting surface made from black phosphorus.
These atoms have quantum properties in the form of unique spin states. The researchers set out to see if they could embed information within these atoms' spin states and simulate 'neuron firing' between them with tiny, applied voltages.
Instead of tangible information, like how tall is the Empire State building, these neuromorphic neurons were instead tasked with remembering binary information, 0s and 1s, which represent different probability distributions for the system.
"The material adapted its reaction based on the external stimuli that it received. It learned by itself."
What they discovered — With this formulation, the researchers were able to closely model real neurons' behavior by applying voltages to their network of atoms. They also observed these atoms demonstrate self-adaptive behavior based on what they remembered "seeing."
"When stimulating the material over a longer period of time with a certain voltage, we were very surprised to see that the synapses actually changed," Khajetoorians says. "The material adapted its reaction based on the external stimuli that it received. It learned by itself."
What's next — Quantum brains are not coming to a robot near you anytime soon. The authors explain in their paper there are still a lot of unknowns to resolve before a system like this is anywhere close to its debut. One outstanding question is what — exactly — is going on within the machine to facilitate these life-like responses.
Looking into the future, Khajetoorians is excited for the possibilities ahead for this machine and the evolution of artificial intelligence.
"We are at a state where we can start to relate fundamental physics to concepts in biology, like memory and learning," he says.
"If we could eventually construct a real machine from this material, we would be able to build self-learning computing devices that are more energy efficient and smaller than today's computers... It is a very exciting time."
Abstract: The quest to implement machine learning algorithms in hardware has focused on combining various materials, each mimicking a computational primitive, to create device functionality. Ultimately, these piecewise approaches limit functionality and efficiency, while complicating scaling and on-chip learning, necessitating new approaches linking physical phenomena to machine learning models. Here, we create an atomic spin system that emulates a Boltzmann machine directly in the orbital dynamics of one well-defined material system. Utilizing the concept of orbital memory based on individual cobalt atoms on black phosphorus, we fabricate the prerequisite tuneable multi-well energy landscape by gating patterned atomic ensembles using scanning tunnelling microscopy. Exploiting the anisotropic behaviour of black phosphorus, we realize plasticity with multi-valued and interlinking synapses that lead to tuneable probability distributions. Furthermore, we observe an autonomous reorganization of the synaptic weights in response to external electrical stimuli, which evolves at a different time scale compared to neural dynamics. This self-adaptive architecture paves the way for autonomous learning directly in atomic-scale machine learning hardware.
This article was originally published on