Brain-like computer chips are a totally wild concept. And they might just be the future of AI
Scientists can use nanomaterials to mimic the human brain's structure.
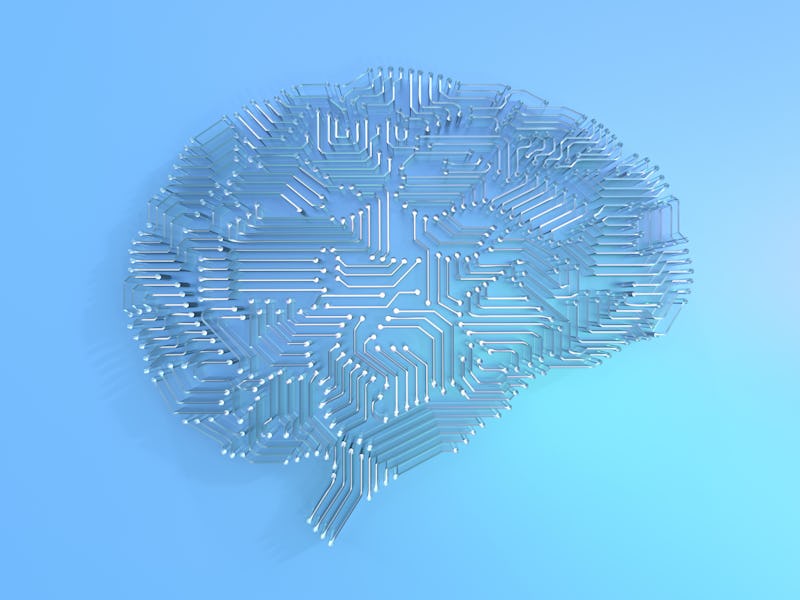
Despite its name, artificial intelligence isn't all that smart -- at least when compared to human brains. A.I.'s are excellent number crunchers and pattern finders, but when it comes to actual human-level cognition and problem-solving, they've still got a ways to go. But that distance could be quickly shortening, thanks to the emergence of a next-generation of A.I. called neuromorphic computing.
Instead of teaching A.I.'s rigid logic gates and processors to learn through strict rules and datasets, neuromorphic computing takes a more biological approach to learning and designs computing systems that mimic a human brain's architecture of neurons and synapses. Authors of a new review published Monday in the journal Nature Nanotechnology write that using nanomaterials to design this architecture will not only drive down energy costs but can enable a more realistic mimicry of our own brains.
While today's A.I. has made a huge impact on how we use technology and data, the authors of the review write that bottlenecks created by its data storage architecture have driven energy costs far too high. This architecture, named after the famous computer scientist and mathematician John von Neumann, relies on physically separate memory and logic blocks in a computer. For example, a computer's CPU is separate from its RAM. This structure worked well when von Neumann designed it in the 1940s, but has become inefficient as technology has matured.
The authors write that parallel processing enabled by neuromorphic computers could provide a strong contrast: hyper-connectivity and saving power at the same time.
"Inspired by the co-location of logic and memory, robustness against local failures, hyper-connectivity and parallel processing in the human brain, alternative neuromorphic computing architectures promise substantially lower power consumption by physically emulating neurons and synapses at the small circuit or device level."
But switching to a neuromorphic design, where logic and memory can coexist as they do in the brain, isn't a simple solution either. Current neuromorphic systems primarily use silicon-based superconducting neural networks that the authors write are set to far surpass their energy limit by 2040 at their current rate.
"[I]f data storage and communication continue to increase at the current rate, the total energy consumed by binary operations using CMOS [silicon-based complementary metal–oxide–semiconductor] will surpass ~1027 Joules in 2040, which exceeds the total energy being produced globally," write the authors.
This kind of design is currently used by in systems like IBM's TrueNorth chip, which is designed to recognize different, unique objects in a video, and the European Union Human Brain Program's SpiNNaker Project, which is designed to execute cognitive tasks.
With this kind of design's clear limitations, the authors suggest focusing instead on different classes of nanomaterials. As described in the review, these nanomaterials come in three distinct flavors and all with different neuromorphic advantages. The smallest material, zero-dimensional nanomaterials, are materials so small that their entire measurement is in the nanoscale. These include nanoparticles like quantum dots. Super tiny materials like these have a promising future in optical calculations write the authors, and along with slightly larger one-dimensional nanomaterials could be used to design neuromorphic wearables like smart prosthetics.
"Neuromorphic computing holds promise for ultralow power computing, particularly for computing applications that include large amounts of data such as machine learning, image processing, and artificial intelligence," coauthor and professor and material science and engineering at Northwestern, Mark Hersam, tells Inverse. "Since neuromorphic computing is attempting to mimic the human brain, it may also be applicable in smart prosthetics and related bioelectronics applications."
Meanwhile, slightly larger two-dimensional nanomaterials, whose measurements cannot be entirely made on the nanoscale, can be used to design synaptic resistors and multilevel memory in chips. These kinds of materials also demonstrate a great deal of synaptic plasticity between different components in the system, write the authors. Synaptic plasticity is much like the plasticity found in the human brain which allows us to learn and connect new information.
Because these computers would better mimic the brain's internal structure, Hersam tells Inverse that neuromorphic computers could decrease computing energy costs by a staggering 100,000-fold.
"The ultimate goal of neuromorphic computing is to achieve supercomputer-level computation at the low power consumption of the human brain," says Hersam. Current supercomputers tend to be at the few megawatt power level, whereas the human brain is at the 20 watt power level – i.e., neuromorphic computing has the potential to be ~100,000-fold lower power consumption compared to conventional digital computing."
The authors write that these materials' ability to closely mimic biological systems demonstrates the potential for how they could be further developed in the future to enable a new generation of intelligent computers.
"The additional chemical sensing, mechanical flexibility and biocompatibility attributes of nanomaterials provide further opportunities to realize edge computing and afferent neurons in artificial skin," write the authors. "In this manner, since the properties and reduced dimensionality of nanomaterials closely mimic biological systems, they possess significant potential for realizing neuromorphic computing systems that better emulate animate neural networks."
Abstract: Memristive and nanoionic devices have recently emerged as leading candidates for neuromorphic computing architectures. While top-down fabrication based on conventional bulk materials has enabled many early neuromorphic devices and circuits, bottom-up approaches based on low-dimensional nanomaterials have shown novel device functionality that often better mimics a biological neuron. In addition, the chemical, structural and compositional tunability of low-dimensional nanomaterials coupled with the permutational flexibility enabled by van der Waals heterostructures offers significant opportunities for artificial neural networks. In this Review, we present a critical survey of emerging neuromorphic devices and architectures enabled by quantum dots, metal nanoparticles, polymers, nanotubes, nanowires, two-dimensional layered materials and van der Waals heterojunctions with a particular emphasis on bio-inspired device responses that are uniquely enabled by low-dimensional topology, quantum confinement and interfaces. We also provide a forward-looking perspective on the opportunities and challenges of neuromorphic nanoelectronic materials in comparison with more mature technologies based on traditional bulk electronic materials.