Are health algorithms racist? A new study has the answer
Even though this algorithm didn't look at race, Black patients still experienced bias.
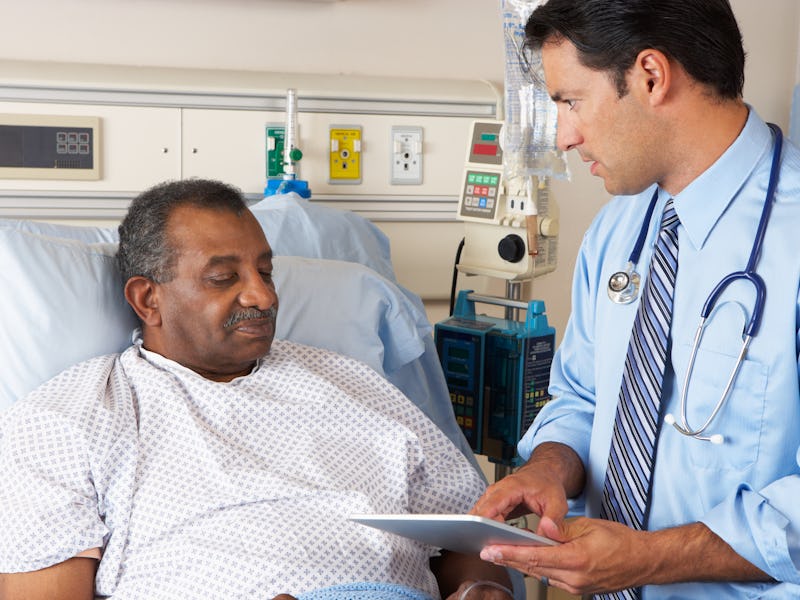
The promise of a world driven by algorithms we design is that they will be less subjective and more just than we are. But in reality, many are riddled with all our same biases and prejudices.
And new research has now found that a widely used healthcare algorithm that predicts which patients will need extra care is no different: It has been recommending white patients for further care over Black patients, despite the Black patients actually being sicker.
The study, published Thursday in the journal Science, looked at data input and output, as well as objective function — what the algorithm is meant to minimize — and compared those factors to patient records in order to reverse engineer the algorithm and see exactly where the biases arose. The examined 43,539 white patients and 6,079 Black patients (all of whom had some form of healthcare insurance) enrolled at a research hospital and evaluated how they were sorted into categories associated with low- or high-risk health needs. The study found that, despite having more chronic illnesses, Black patients were recommended for extra care at a lower rate than their less sick white counterparts.
Even though the algorithm purposefully didn’t consider race when making these decisions, the compounding factors it ignored showed up as algorithmic bias, says Sendhil Mullainathan, Ph.D., professor of computation and behavioral science at The University of Chicago Booth School of Business and senior author of the study. Namely, instead of looking at patient health as a determining factor in who needed extra care, the algorithm instead focused on overall healthcare costs.
Despite their best intentions, scientists that don't think critically about the algorithms they're creating can introduce bias without even meaning to.
“Instead of being trained to find the sickest, in a physiological sense, [these algorithms] ended up being trained to find the sickest in the sense of those whom we spend the most money on,” Mullainathan says in a press release. “And there are systemic racial differences in health care in who we spend money on.”
When the researchers turned to look at the healthcare costs of the individuals in the data set, the amount of money spent on care appeared roughly the same — despite the aforementioned health disparities — but when examined more deeply, the kinds of care being paid for by Black patients told a different story. When less sick, white patients paid more for things like inpatient care or surgeries, while Black patients paid more for emergency room visits and dialysis.
This, the researchers say, points toward further inequality in the access Black patients have to healthcare. Despite having insurance, socioeconomic disparities in these communities still persist, as well as reluctance to seek care. The researchers say that this reflects reduced trust in the healthcare system and negative doctor-patient relationships between Black patients and non-Black physicians.
Ziad Obermeyer, Ph.D., acting associate professor of health policy and management at UC Berkeley and lead author of the paper, says that this less nuanced understanding of how Black patients may use the healthcare system resulted in algorithmic bias.
“Because of the structural inequalities in our health care system, Blacks at a given level of health end up generating lower costs than whites,” Obermeyer says. “As a result, Black patients were much sicker at a given level of the algorithm’s predicted risk.”
A healthcare algorithm recommended Black patients for extra care at lower rates than white patients.
So, even though the Black patients were sicker, the amount they spent on healthcare didn’t reflect their sickness — at least in the eyes of the algorithm. The authors write that this system, which is used for over 200 million patients annually in America, is symbolic of how such biases play out in policing and job searches as well.
But, in this case at least, the authors say it was still possible to fix the algorithm. In collaboration with the algorithm’s creators, the researchers shared their data and approaches to fixing the biases found in the algorithm. Still not quantifying race itself, the researchers and company were able to design a new algorithm that focused on patient health and instead of their healthcare costs, which led to an 84 percent reduction in bias.
“Algorithms can do terrible things, or algorithms can do wonderful things. Which one of those things they do is basically up to us,” Obermeyer says. “We make so many choices when we train an algorithm that feel technical and small. But these choices make the difference between an algorithm that’s good or bad, biased or unbiased…. [and] those choices are hard.”
Abstract:
Health systems rely on commercial prediction algorithms to identify and help patients with complex health needs. We show that a widely used algorithm, typical of this industry-wide approach and affecting millions of patients, exhibits significant racial bias: At a given risk score, Black patients are considerably sicker than White patients, as evidenced by signs of uncontrolled illnesses. Remedying this disparity would increase the percentage of Black patients receiving additional help from 17.7 to 46.5%. The bias arises because the algorithm predicts health care costs rather than illness, but unequal access to care means that we spend less money caring for Black patients than for White patients. Thus, despite health care cost appearing to be an effective proxy for health by some measures of predictive accuracy, large racial biases arise. We suggest that the choice of convenient, seemingly effective proxies for ground truth can be an important source of algorithmic bias in many contexts.