The Doctor of the Future is a Computer that Says When You Will Die
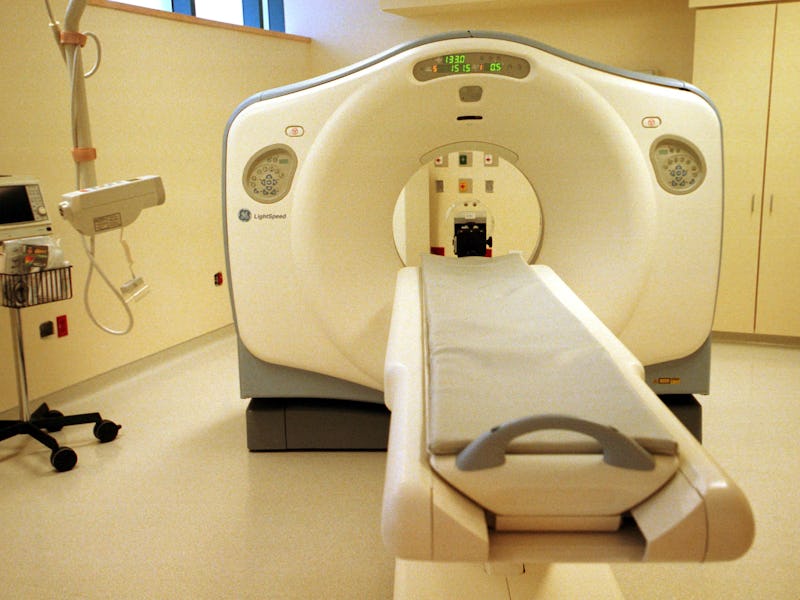
Scientists have developed a new A.I. system which uses deep learning algorithms to scan and analyze the CT images of a patients organs to determine which patients are most likely to die within five years, according to new results published in Scientific Reports last month. The new program has a strong potential to push forward illness diagnosis in the medical world to new heights of accuracy and efficiency — although it will certainly take a lot more work before we start seeing A.I. systems like this being utilized in hospitals around the world.
In 2007, 69 million computerized tomography (CT) scans were done on Americans (nice). While these high-quality x-rays can help doctors find egregious health problems, they often don’t know what they’re looking for. It turns out that it’s hard to get a close look at people’s organs until it’s too late, and early warnings signs for heart disease are hard to spot.
That’s why the new program, developed by scientists from the University of Adelaide in Australia, is so encouraging. But if you’re touched by a morbid curiosity, don’t ask the A.I. to tell your fortune just yet. While the goal is to design medical screenings that can catch early warning signs of heart disease, cancer, and other dangerous diseases nearly instantaneously, the tech isn’t there just yet.
The A.I., trained by looking through old CT scans of former patients and comparing that data to how long the patient lived afterward, was exposed to only small number of patients. Moreover, it was only correct about 69 percent of the time, which of course is still nice, but not nice enough. It means that for every three times that the A.I. concluded that a patient would be dead in five years, one of those people was actually fine.
Or rather, since in this early stage of research the computer was looking back at people who died in 2014, one in three of them happened to have lived longer than five years after receiving the CT scan in question.
A.I. can predict who will die within five years at 69 (nice) percent accuracy. While this leaves a lot of false positives, human doctors perform at just about the same rate.
They focused on an older population, and found that in people over the age of sixty, there were a number of telling signs in people’s hearts and other organs that gave away how much longer they might live. The research was conducted on just 24 people, which is abysmally low for biomedical experimentation. But the researchers behind the study are reportedly working on expanding their work and training their A.I. on over 12,000 more people, which they hope will make their technique more accurate in the future.
Time is fleeting, man. And if you want to know how long you’ve got left, computers like these might soon be able to give you a number. As the technology improves, the scientists who trained this morbid A.I. hope that their improved techniques to spot disease early could help people stick around longer. But for now, the best it can do is predict when you might croak.
Abstract:
Precision medicine approaches rely on obtaining precise knowledge of the true state of health of an individual patient, which results from a combination of their genetic risks and environmental exposures. This approach is currently limited by the lack of effective and efficient non-invasive medical tests to define the full range of phenotypic variation associated with individual health. Such knowledge is critical for improved early intervention, for better treatment decisions, and for ameliorating the steadily worsening epidemic of chronic disease. We present proof-of-concept experiments to demonstrate how routinely acquired cross-sectional CT imaging may be used to predict patient longevity as a proxy for overall individual health and disease status using computer image analysis techniques. Despite the limitations of a modest dataset and the use of off-the-shelf machine learning methods, our results are comparable to previous ‘manual’ clinical methods for longevity prediction. This work demonstrates that radiomics techniques can be used to extract biomarkers relevant to one of the most widely used outcomes in epidemiological and clinical research – mortality, and that deep learning with convolutional neural networks can be usefully applied to radiomics research. Computer image analysis applied to routinely collected medical images offers substantial potential to enhance precision medicine initiatives.