This Algorithm Diagnoses Depression From Your Instagram Feed
Your choice of filter matters more than you think.
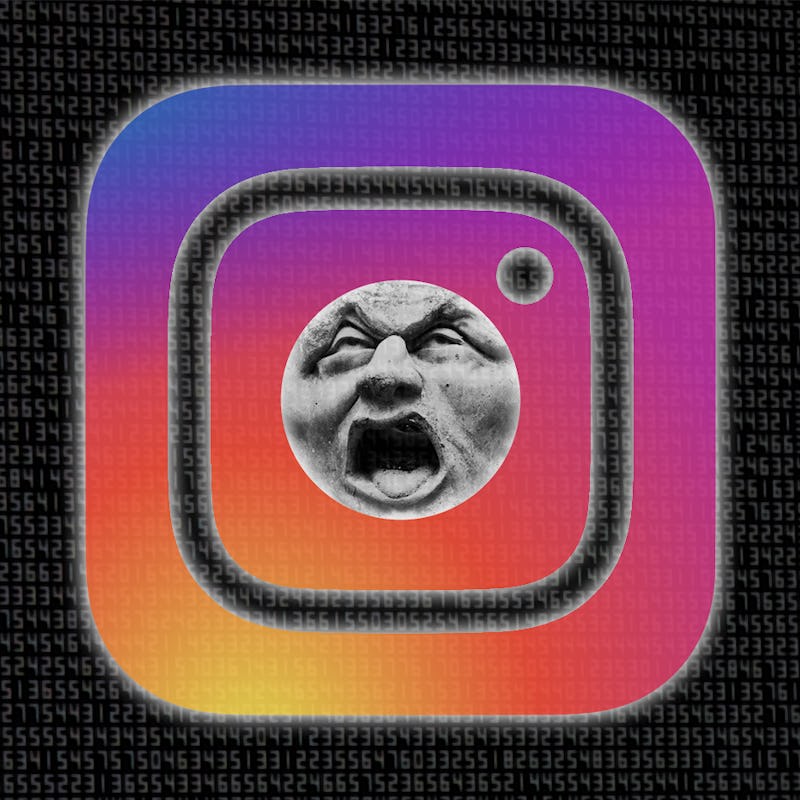
A psychology graduate student and a professor of mathematical, natural, and technical sciences may have just cracked clinical depression wide open. The answer, they found, may lie in your Instagram feed — if their groundbreaking machine learning algorithm gets a look at your photos.
Harvard University’s Andrew Reece and the University of Vermont’s Chris Danforth crafted an algorithm that can correctly diagnose depression, with up to 70 percent accuracy, based on a patient’s Instagram feed alone.
“When someone posts a photo to Instagram,” Reece tells Inverse via email, “that’s a purposeful choice that’s meant to express something. What that something is, and how closely it hews to the poster’s worldview, is harder to infer.”
For Reece and Danforth’s algorithm, however, it’s not.
As algorithms get wiser, your behavior in virtual space becomes ever more valuable. In the modern, connected world, there are few remaining havens where you’re not a cog in the data machine, outputting personal information to a legion of algorithms that, each second, analyze the shit out of you. Everyone is a faceless member of some demographic, a resource to be mined by companies that profit off of data. Googling Natural home remedies for yellowing teeth, for instance, tells the internet’s uncaring gods that you are an ashamed smoker. Visiting OkCupid tells them that you’re lonely, horny, or both. Everything you do on Facebook — down to where your cursor hovers — informs what you see, and what Facebook thinks about you.
Instagram, on the other hand, seems relatively innocent. There’s not much data to harvest: Users can take or upload a photo or video, edit it or apply filters, and jot down a caption with or without hashtags. Followers can like or comment on these posts. And, like Facebook, which acquired Instagram in 2012, user behavior informs their suggestions and Explore tab. In other words, if you click on puppy photos all the time, Instagram will know your soft spot, and increase the supply of cute dogs.
It’s not a stretch to call some of this a good thing. In 2016, people spend about as much time, if not more, traversing virtual terrain as they do with real people in the real world. Online, algorithms are learning details that previously only people close to you knew; they know your preferences, your moods, even your insecurities. The impersonal internet is becoming your friend. Algorithms can parse the endless stream of data; they can see beyond the ebbs and flows and generate personalized tide charts. Most algorithms, however, rely on speech, written text, or browsing patterns, which are great sources for sentiment analysis.
Instead, Reece and Danforth’s algorithm relies on photos — and it’s particularly clairvoyant. After a careful screening process, the team analyzed almost 50,000 photos from 166 participants, all of whom were Instagram users and 71 of whom had already been diagnosed with clinical depression. Their results confirmed their two hypotheses: first, that “markers of depression are observable in Instagram user behavior,” and second, that “these depressive signals are detectable in posts made even before the date of first diagnosis.”
The duo had good rationale for both hypotheses. Photos shared on Instagram, despite their innocent appearance, are data-laden: Photos are either taken during the day or at night, in- or outdoors. They may include or exclude people. The user may or may not have used a filter. You can imagine an algorithm drooling at these binary inputs, all of which reflect a person’s preferences, and, in turn, their well-being. Metadata is likewise full of analyzable information: How many people liked the photo? How many commented on it? How often does the user post, and how often do they browse? But Reece and Danforth discovered that, beyond those more obvious characteristics, a photo’s subtleties are even more telling. Its very appearance — down to its per-pixel color and brightness statistics — indicates more than most Instagram users would care to know.
Many studies have shown that depressed people both perceive less color in the world and prefer dark, anemic scenes and images. The majority of healthy people, on the other hand, prefer colorful things. Reece and Danforth went beyond these now-platitudes. They collected each photo’s hue, saturation, and value averages. Depressed people, they found, tended to post photos that were more bluish, unsaturated, and dark. “Increased hue, along with decreased brightness and saturation, predicted depression,” they write.
Reece and Danforth also found that happy people post less than depressed people. And happy people post photos with more people in them than do their depressed counterparts. “We intended presence of faces in photos to stand in as a proxy for social engagement, which is often reduced or avoided by individuals suffering from depression,” Reece says. “The thinking goes, more faces means bigger social groups for the person who posted the photos.”
And, further, depressed participants were less likely to use filters. When participants did use filters, their choices proved telling. The overwhelming filter preference for healthy participants was Valencia; for depressed participants, it was Inkwell.
“It seems natural to wonder whether these machine-extracted features pick up on similar signals that humans might use to identify mood and psychological condition,” Reece and Danforth write, “or whether they attend to wholly different information.” To answer this question, and to test the effectiveness of their algorithm, they enlisted people to judge selections from their Instagram content pool. The judges proved decent at determining people’s depression or well-being, but “exhibited extremely low correlation with computational features.” In other words, the algorithm discerns more than its creators, perceives something beyond what should be perceptible.
A dog named Tuukka in #nofilter, #valencia, and #inkwell.
These findings are significant: Diagnosing depression is serious business, and professionals are at best mediocre. Early detection is crucial, but, as it stands, doctors often rely on unreliable methods. “General practitioners were able to correctly rule out depression in non-depressed patients 81% of the time, but only diagnosed depressed patients correctly 42% of the time,” Reece and Danforth write. Even professionals, despite their best efforts, fail to obtain objectivity, and, as humans, are fallible. False diagnoses, the duo points out, are “costly for both healthcare programs and individuals.”
Reece and Danforth’s discovery suggests a future in which mental health algorithms pick up on depression before people do. Algorithms approximate objectivity: Data collection is uniform and relatively unbiased, and the output is easy to interpret. The current version, at 70 percent diagnostic accuracy, is already excellent; future iterations could be near-flawless. An algorithm like this one, rather than being an advertiser’s wet dream, could be an opt-in resource for people struggling with their mental health. “Just this year a study was published showing that pancreatic cancer could be predictively screened for, based on the symptoms people searched for online. That’s the kind of early warning system I think a lot of people might want to have access to,” Reece says.
What’s unclear is how aware the participants were of their depression, and whether such knowledge would change what someone posted. (The depressed participants had already been diagnosed, but Reece and Danforth were careful enough to study the subset of pre-diagnosis photos independently; the algorithm, despite a smaller sample size, did fairly well compared to the entire data set.) “We don’t know if people already thought of themselves as depressed, or whether they were unconsciously exhibiting depressive symptoms (reduced attention to color, etc.).” Reece admits that it’s “hard to separate these two,” but either possibility is compelling.
No matter the individual’s self-awareness, and no matter the brand they attempt to cultivate on Instagram, Reece and Danforth’s discoveries raise uncomfortable questions about privacy. “The privacy issues arise when you zoom out and look at a lot of posts all at once, algorithmically,” Reece says. “At that point, personal details might emerge that were never intended to be public.” For this study, they “guaranteed all of our study participants that no personal details whatsoever, including their Instagram posts, would ever be made public,” he explains. “Only our research team members, who are all trained in handling human subjects research data, and who are legally required to operate under strict ethical guidelines, were ever permitted to see confidential data.”
It’s not unreasonable to assume — given their track records — that advertisers, analysts, and government agencies will be less cautious than researchers with this mineable data. Much of the data is public, and the remainder is but a few queries away. “Allowing algorithms to access your personal data history has the potential to become a Pandora’s box,” Reece says. “It’s fine to allow benevolent watchmen access to your data, for the purpose of providing better and faster health care. But once your data are out there, who watches the watchmen?”