Studies show why your decisions must rely on the past
Even down to your morning coffee order.
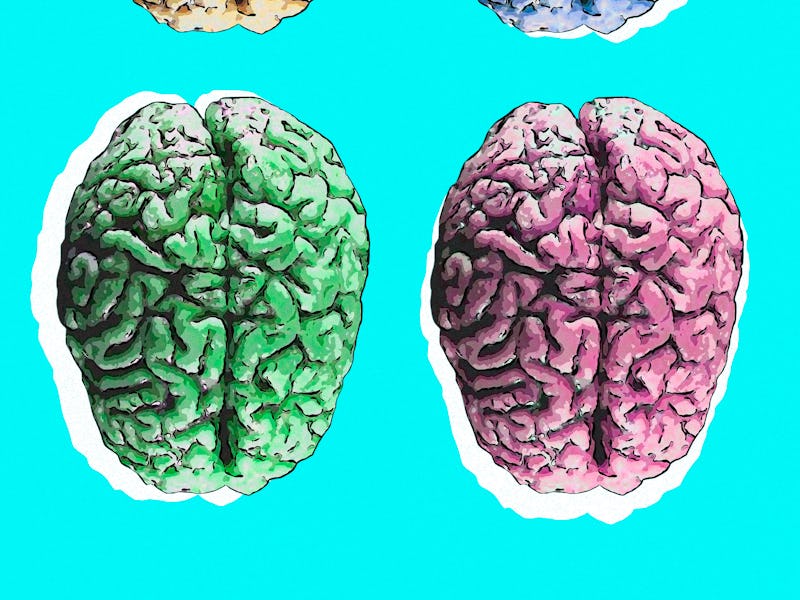
Whenever you make a decision, networks of specialized cells called neurons in your brain go to work to determine the best choice. These cells’ computational work actually lights up our brains. (Stanford researchers this year unveiled a new device they developed that captures this light.)
How do neurons actually reach decisions? They rely on different parts of the brain to analyze our experiences and the context around a decision, then work together before arriving on an ultimate decision, according to recent research.
Past experience storage
Many times when we make decisions, we rely on our past experiences or what we have learned from others. After analyzing tens of thousands of mice neurons (human and mice brains have many similarities), UC San Diego neurobiologists discovered that an area of the brain called the retrosplenial cortex is responsible for these value-based decisions. The researchers said an example of a decision the RSC is responsible for is where you buy your morning coffee.
“When you have two coffee shops to choose from, no one is telling you which one to go to — you rely on the internal value in order to choose one over the other,” said senior study author Takaki Komiyama. “We found that the RSC, which previously had not been studied in the context of value-based decision-making, showed the strongest value information most persistently over time.”
Putting it together
The context around every decision that we make has a significant impact on what we decide. For example, when crossing the street in the US, we first look left, but it’s the opposite in the UK. Columbia researchers have found that a part of the mouse brain called the anterior lateral motor cortex is responsible for this contextual part of decision-making. The ALM had been previously thought to be the part of the brain that guides movements of a mouse’s tongue and facial muscles.
“Context-dependent decision-making is a building block of higher cognitive function in humans,” said senior co-author Michael Shadlen.
The researchers discovered the ALM after conducting an experiment with mice in which they had to remember a specific odor. After a brief pause, the researchers puffed a second odor over the nostrils of the mouse. If the odors matched, the mouse had to lick a tube to the left to get water. If the two odors differed, it had to lick a tube to the right. The researchers expected that the primary brain process for success in the experiment was the olfactory system, but they found that when they disabled the mice’s ALM, they couldn’t complete the task.
“The first odor acts as a contextual clue, priming the ALM to then indicate that relationship by deciding which way to lick in response to the second odor,” said neuroscientist Ashok Litwin-Kumar.
Crowdsourcing decisions
After analyzing data recorded from the neurons of a macaque monkey tasked with making a simple decision, Sante Fe Institute researchers discovered that it appears decisions are a group effort before individual neurons step up for a final call.
“As the monkey initially processes the data, polling many neurons is required to get a good prediction of the monkey's decision,” according to a release summarizing the study. “Then, as the time for committing to a decision approaches, this pattern shifts. The neurons start to agree and eventually each one on its own is maximally predictive.”
Study co-author Bryan Daniels theorizes that the crowdsourcing step is necessary to filter out useful information from a lot of noise, using the wisdom of the crowd to produce a “coherent” decision. Then each individual neuron shares their individual decisions to arrive at a consensus and execute.
A central question in cognitive neuroscience is how unitary, coherent decisions at the whole organism level can arise from the distributed behavior of a large population of neurons with only partially overlapping information. We address this issue by studying neural spiking behavior recorded from a multielectrode array with 169 channels during a visual motion direction discrimination task. It is well known that in this task there are two distinct phases in neural spiking behavior. Here we show Phase I is a distributed or incompressible phase in which uncertainty about the decision is substantially reduced by pooling information from many cells. Phase II is a redundant or compressible phase in which numerous single cells contain all the information present at the population level in Phase I, such that the firing behavior of a single cell is enough to predict the subject's decision. Using an empirically grounded dynamical modeling framework, we show that in Phase I large cell populations with low redundancy produce a slow timescale of information aggregation through critical slowing down near a symmetry-breaking transition. Our model indicates that increasing collective amplification in Phase II leads naturally to a faster timescale of information pooling and consensus formation. Based on our results and others in the literature, we propose that a general feature of collective computation is a “coding duality” in which there are accumulation and consensus formation processes distinguished by different timescales.
This article was originally published on