How to spot a liar using a classic mathematical trick
Opinion: Can mathematics distinguish lying from a genuine misunderstanding?
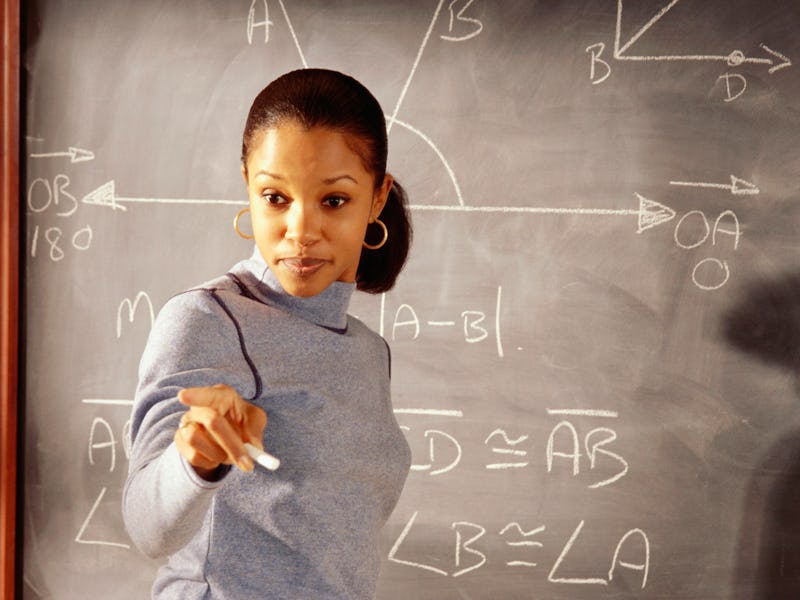
Understanding the human mind and behavior lies at the core of the discipline of psychology. But to characterize how people’s behavior changes over time, I believe psychology alone is insufficient — and that additional mathematical idea needs to be brought forward.
My new model, published in Frontiers in Psychology, is inspired by the work of the 19th-century American mathematician Norbert Wiener. At its heart is how we change our perceptions over time when tasked with making a choice from a set of alternatives. Such changes are often generated by limited information, which we analyze before making decisions that determine our behavioral patterns.
Math v. information
To understand these patterns, we need the mathematics of information processing. Here, the state of a person’s mind is represented by the likelihood it assigns to different alternatives — which product to buy, which school to send your child to, which candidate to vote for in an election, and so on.
Opinion polling before an election can give clues to who will win.
As we gather partial information, we become less uncertain — for example, by reading customer reviews, we become more certain about which product to buy. This mental updating is expressed in a mathematical formula worked out by the 18th-century English scholar Thomas Bayes. It essentially captures how a rational mind makes decisions by assessing various uncertain alternatives.
When combining this concept with the mathematics of information (specifically signal processing), dating back to the 1940s, it can help us understand the behavior of people, or society, guided by how information is processed over time. It is only recently that my colleagues and I realized how useful this approach could be.
So far, we have successfully applied it to model the behavior of financial markets (market participants respond to new information, which leads to changes in stock prices) and the behavior of green plants (a flower processes information about the location of the sun and turns its head towards it).
I have also shown it can be used to model the dynamics of opinion poll statistics associated with an election or a referendum and drive a formula that gives the actual probability of a given candidate winning a future election based on today’s poll statistics and how the information will be released in the future.
In this new “information-based” approach, the behavior of a person — or group of people — over time is deduced by modeling the flow of information. So, for example, it is possible to ask what will happen to an election result (the likelihood of a percentage swing) if there is “fake news” of a given magnitude and frequency in circulation.
But perhaps most unexpected are the deep insights we can glean into the human decision-making process. We now understand, for instance, that one of the key traits of the Bayes updating is that every alternative, whether it is the right one or not, can strongly influence the way we behave.
If we don’t have a preconceived idea, we are attracted to all of these alternatives irrespective of their merits and won’t choose one for a long time without further information. This is where the uncertainty is greatest, and a rational mind will wish to reduce the uncertainty so that a choice can be made.
But if someone has a very strong conviction on one of the alternatives, then whatever the information says, their position will hardly change for a long time — it is a pleasant state of high certainty.
Such behavior is linked to the notion of “confirmation bias” — interpreting information as confirming your views even when it actually contradicts them. This is seen in psychology as contrary to the Bayes logic, representing irrational behavior. But we show it is, in fact, a perfectly rational feature compatible with the Bayes logic — a rational mind simply wants high certainty.
The rational liar
The approach can even describe the behavior of a pathological liar. Can mathematics distinguish lying from a genuine misunderstanding? It appears that the answer is “yes,” at least with a high level of confidence.
A lie-detector.
If a person genuinely thinks an alternative that is obviously true is highly unlikely — meaning they are misunderstanding — then in an environment in which partial information about the truth is gradually revealed, their perception will slowly shift towards the truth, albeit fluctuating over time. Even if they have a strong belief in a false alternative, their view will very slowly converge from this false alternative to the true one.
However, if a person knows the truth but refuses to accept it — is a liar — then according to the model, their behavior is radically different: they will rapidly choose one of the false alternatives and confidently assert this to be the truth. (In fact, they may almost believe in this false alternative that has been chosen randomly.) Then, as the truth is gradually revealed and this position becomes untenable, very quickly and assertively, they will pick another false alternative.
Hence a rational (in the sense of someone following the Bayes logic) liar will behave in a rather erratic manner, which can ultimately help us spot them. But they will have such a strong conviction that they can be convincing to those who have limited knowledge of the truth.
For those who have known a consistent liar, this behavior might seem familiar. Of course, without access to someone’s mind, one can never be 100 percent sure. But mathematical models show that for such behavior to arise from a genuine misunderstanding is statistically very unlikely.
This information-based approach is highly effective in predicting the statistics of people’s future behavior in response to the unraveling of information — or disinformation, for that matter. It can provide us with a tool to analyze and counter, in particular, the negative ramifications of disinformation.
This article was originally published on The Conversation by Dorje C. Brody at the University of Surrey. Read the original article here.
This article was originally published on