Video shows the lunar sensors powering MIT’s new autonomous car
These sub-zero cars are really cool.
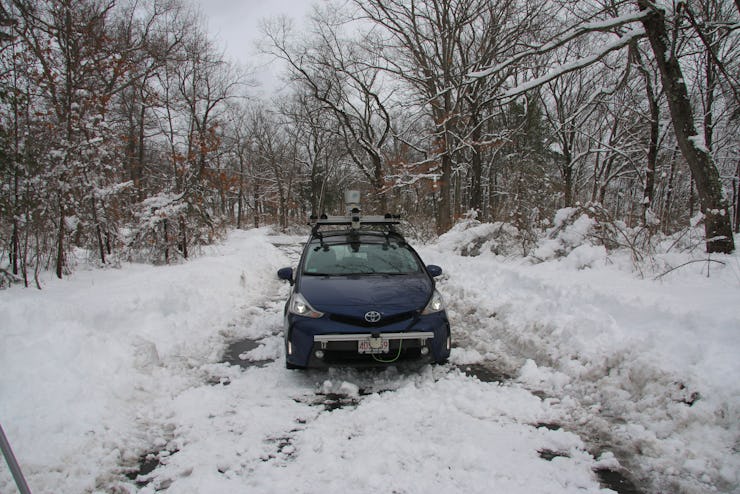
Autonomous cars may seem cool, but when the temperatures really drop they can start to suffer.
Although these self-driving vehicles may currently struggle under snowy conditions, a team at MIT’s Computer Science and Artificial Intelligence Lab may have the answer. They employed a "ground-penetrating radar," or a GPR, to look under the car while it's driving. This is the first time an autonomous car has employed the sensor, usually used on situations like detecting landmines and exploring the moon. The research, announced Monday, is set to be published in the journal IEEE Robotics and Automation Letters later this month.
"While the team has not yet integrated GPR directly into systems that also incorporate camera and lidar, this would be a logical next step for creating a more comprehensive and holistic self-driving system," an MIT spokesperson tells Inverse. "In future work they plan to explore what would be needed to make that happen effectively."
Self-driving car systems currently under development, like at Google's self-driving project now branded Waymo, use a combination of sensors to help the in-car computer make informed choices. They use cameras for sight, and combine them with lidar sensors that bounce lasers and measure the time they take to return to understand depth. Some, like Tesla's under-development system, use ultrasonic sensors. But these sensors can get confused in snow, like when a camera can't see in the brilliant white or when a lidar's lasers bounce back in the snowfall.
The GPR could help. This uses electromagnetic sensors to measure the combination of rocks, soil and roots. This unique fingerprint can be used to help the car identify its current position even when cameras and lidar can fail to pick up cues. This specific version was a localizing ground-penetrating radar, or LGPR, developed at the MIT Lincoln Laboratory.
“If you or I grabbed a shovel and dug it into the ground, all we’re going to see is a bunch of dirt,” CSAIL PhD student Teddy Ort, lead author on the paper, said in a statement. “But LGPR can quantify the specific elements there and compare that to the map it’s already created, so that it knows exactly where it is, without needing cameras or lasers.”
The LGPR fared impressively in the six months and 10.5 miles of tests, traversing a closed country road at a low speed. The margin of error in snowy conditions was around an inch compared to clear weather. Unfortunately, this increased to 5.5 inches in rain as it changes the condition of the soil. In the whole period of testing, the team never had to take over.
The sensor on the rear.
The system would have to work in conjunction with other sensors for a full self-driving solution, though. But the maps required for the sensor would use around 20 percent less space than other maps, and they would have the benefit of changing less often than other, above-ground maps.
The sensor would need to continue development, to allow for more complex road layouts like intersections. It's also relatively bulky at this stage, measuring six feet across. But most observers of the autonomous car space, used to long delays and timelines, probably won't flinch at the idea of another wait.
Abstract: Most autonomous driving solutions require some method of localization within their environment. Typically, onboard sensors are used to localize the vehicle precisely in a previously recorded map. However, these solutions are sensitive to ambient lighting conditions such as darkness and inclement weather. Additionally, the maps can become outdated in a rapidly changing environment and require continuous updating. While LiDAR systems don’t require visible light, they are sensitive to weather such as fog or snow, which can interfere with localization. In this paper, we utilize a Ground Penetrating Radar (GPR) to obtain precise vehicle localization. By mapping and localizing using features beneath the ground, we obtain features that are both stable over time, and maintain their appearance during changing ambient weather and lighting conditions. We incorporate this solution into a full-scale autonomous vehicle and evaluate the performance on over 17 km of testing data in a variety of challenging weather conditions. We find that this novel sensing modality is capable of providing precise localization for autonomous navigation without using cameras or LiDAR sensors.