This simple AI could speed up the Covid-19 cure
This silver bullet can help scientists sift through scientific literature in record time.
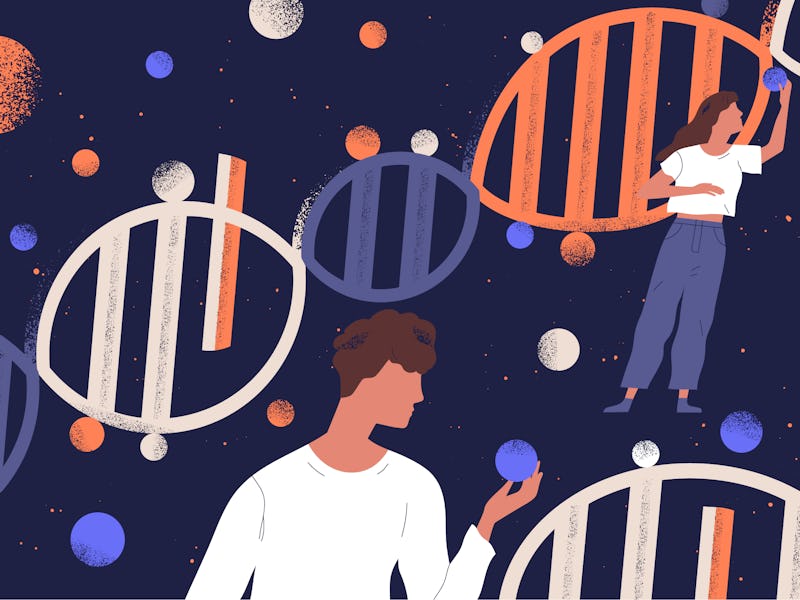
Scientists are working day and night to fight against Covid-19, which is a blessing and a curse. With different research being done all around the world, it's difficult to know which solutions deserve their time, money, and resources. Trying to speed the process along, scientists are forgoing the time-tested "peer review" model of printing findings in favor of so-called open science, which risks accuracy. It's a classic problem of trying to find the needle in the haystack, except the hay is coming from everyone and everywhere on Earth.
Using a machine-learning algorithm trained on thousands of multidisciplinary research papers, a team of computer scientists and sociologists from Northwestern University have developed a scalable method to help scientists identify the most promising solutions for this global problem.
The team says this approach could help speed up the research and development process for Covid-19, including the discovery of a vaccine.
The inspiration behind the algorithm can be traced back to another scientific problem plaguing researchers: science's replication crisis.
One of the main reasons we trust scientific research is because of the rigorous data collection, analysis, and peer-review process that studies must go through before reaching the public. This process is put in place to ensure that results presented in these studies can actually be replicated, meaning that someone else can also confirm they're true. For example, we can all independently test the effect of gravity by dropping objects and watching them fall -- this means that Newton's theory can be replicated.
"We don't think that the algorithm should be replacing human reviewers. It should be in support of human reviewers."
But in recent years the reliability of long-used research methods have come into question, including many in the social sciences. In 2015 a study tested the reproducibility of 100 psychology papers and found that 61 out of 100 tested couldn't be replicated. Worse yet, these weren't necessarily poorly written papers that had skipped peer-review. Instead, there simply seemed to be something wrong with the field's most basic scientific methods.
In the new study, published Monday in the journal Proceedings of the National Academy of Sciences, a research team led by Northwestern professor sociology and management, Brian Uzzi, has developed a machine-learning algorithm to improve how the replicability of studies is measured.
"Science is supposed to replicate," Uzzi tells Inverse. "So if a finding is published, people want to believe it will work the next time somebody uses it and the next time... Typically [peer-reviewers] judge papers replicability based on the statistics that are reported... We trained an artificial intelligence system not to use the statistics in the report but the narrative."
Uzzi tells Inverse that evaluating the narrative of a scientific paper to judge is replicability is not only more accurate than using statistics alone but it is also much harder for humans to do unassisted.
To do this the team first trained a machine-learning model to read the statistics and text of over 2 million study abstracts. The model was then given a new sample of studies to evaluate based first on their narrative text alone and then on the statistical data of the studies alone. Using two different evaluation metrics, the researchers found that judging these samples based on just their narrative content was more accurate than just statistics alone, with 74 percent accuracy for the narrative and 72 percent for the stats.
Both methods outperformed traditional evaluation schemes used by human evaluators by at least 10 percent. Interestingly, Uzzi tells Inverse that both of these methods, DARPA's SCORE evaluation and peer-review-like prediction market, both rely solely on statistical results.
But the researchers say that combining this algorithmic model with the keen eye of human reviewers would yield the best overall results.
"We don't think that the algorithm should be replacing human reviewers," says Uzzi. "It should be in support of human reviewers. We feel that for two reasons. One, as a researcher myself, and many other researchers feel this way, wouldn't it be great if there was an algorithm I could run my paper through before [publishing it] to get a sense of whether it's going to replicate? It could also be potentially used in the review process... it could allow you to have a broader perspective on that paper."
Uzzi tells Inverse that this algorithm could be an incredibly useful tool right now as researchers work to understand and treat Covid-19.
"There's a tremendous urgency to come up with a new drug, or therapy, or cure for Covid-19," says Uzzi. "And the way people are going to do that is they're going to go into the literature and start looking at what's been done before and they're going to try to build on it. An algorithm like ours could go into that literature right now and begin to pinpoint papers that are unlikely to replicate."
Uzzi also tells Inverse that their team is already working on expanding the application of this algorithm beyond life and physical sciences to economics and business as well. In the future, when business is beginning to rebuild after the Covid-19 financial crisis, Uzzi says this could help investors decide which companies would be best to support.
Abstract: Replicability tests of scientific papers show that the majority of papers fail replication. Moreover, failed papers circulate through the literature as quickly as replicating papers. This dynamic weakens the literature, raises research costs, and demonstrates the need for new approaches for estimating a study’s replicability. Here, we trained an artificial intelligence model to estimate a paper’s replicability using ground truth data on studies that had passed or failed manual replication tests, and then tested the model’s generalizability on an extensive set of out-of-sample studies. The model predicts replicability better than the base rate of reviewers and comparably as well as prediction markets, the best present-day method for predicting replicability. In out-of-sample tests on manually replicated papers from diverse disciplines and methods, the model had strong accuracy levels of 0.65 to 0.78. Exploring the reasons behind the model’s predictions, we found no evidence for bias based on topics, journals, disciplines, base rates of failure, persuasion words, or novelty words like “remarkable” or “unexpected.” We did find that the model’s accuracy is higher when trained on a paper’s text rather than its reported statistics and that n-grams, higher order word combinations that humans have difficulty processing, correlate with replication. We discuss how combining human and machine intelligence can raise confidence in research, provide research self-assessment techniques, and create methods that are scalable and efficient enough to review the ever-growing numbers of publications—a task that entails extensive human resources to accomplish with prediction markets and manual replication alone.