A.I. can now identify brain tumors better than humans
Computers could help prevent the coming "pathologist gap."
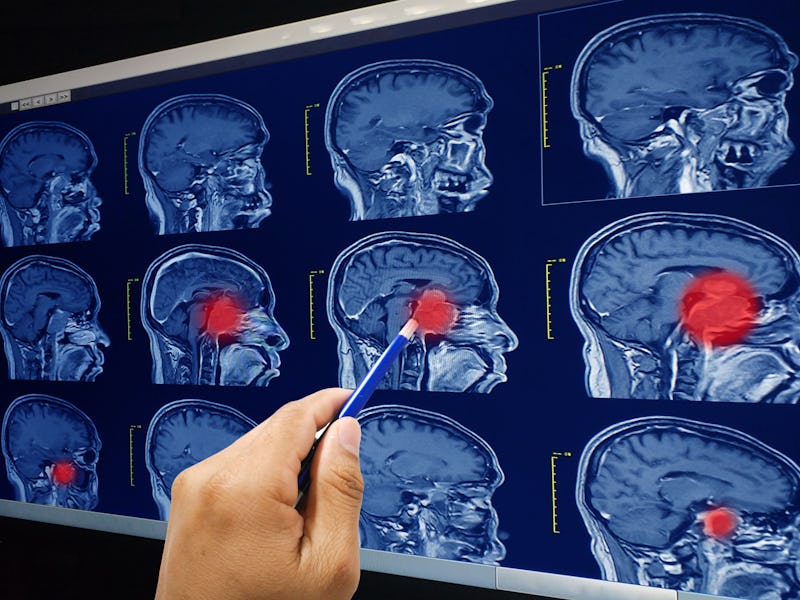
Analyzing biopsied tissue samples for signs of malignant growth is a crucial part of cancer diagnosis. But many places around the country suffer from a lack of neuropathologists, which prevents these fundamental procedures from happening in a timely manner. Recent studies have warned of a “pathologist gap” which may grow through 2030. Scientists have demonstrated an A.I. not only capable of completing such procedures in less than three minutes, but can do so more accurately than a human.
Of the 15.2 million people around the world diagnosed annually with some form of cancer, nearly 80 percent will undergo biopsy surgery to remove and test a piece of the tumor. In the case of brain tumors, which this new study focused on, the testing process can take up to 30 minutes (if there’s a neuropathologist on hand to conduct the test) and requires time and labor-intensive freezing, thawing and chemical staining of samples in order to make a diagnosis. While scientists aren’t looking to necessarily replace the expertise of these pathologists, they believe added automation could benefit both patients and neuropathologists alike.
The study that explores this idea was published Monday in the journal Nature Medicine and used a combination of a deep learning convolutional neural network (CNN) and an optical imaging technique called stimulated Raman histology (SRH) to create an A.I. pathologist that could diagnosis cancer in patients at least as accurately as human neuropathologists. To train the CNN the team fed it 2.5 million pieces of labeled samples of brain cancer diagnoses and the related tissue characteristics and taught it to identify 13 different histological categories (i.e. different types of brain cancer.)
What stands out about this study, study co-author and associate professor of Neurosurgery at NYU Langone Health, Daniel Orringer, tells Inverse, is that it demonstrates how A.I. can be used in the medical field today.
“Our paper describes how computer vision can help to classify brain tumors,” Orringer tells Inverse. “I think what is most notable about our study is that our study shows how AI can be used today! There’s no speculation here. We’ve shown how this works in a large number of patients at three different centers. The other unique and, I think spectacular, thing about our work is that we used a technique called activation maximization to “ask” the algorithm about the features it’s using to classify brain tumors. What it “told” us was that it uses features (that it came up with entirely organically) that are similar to those that are used by human pathologists to detect cancer: cell shape, size, DNA pattern, etc.”
After successfully training their A.I. to think like a neuropathologist, it was time to put it to the test against the real thing.
Compared to the A.I. diagnosis procedure, neuropathologists put in more effort and can take longer to make the same diagnosis.
The second section of a chart comparing A.I and human neuropathologists.
The third section of the chart. A fully trained A.I only needs 180 seconds for such a test, while it would take a human doctor approximately half an hour.
To do this the team took 278 samples from tumor patients across three different hospitals and gave half to the human neuropathologists and half to the A.I. The team found that the A.I. was not only able to process the image and make a diagnosis in less than 150 seconds but actually did so more accurately than the neuropathologists who took about 30-minutes. When looking at results the team saw that the CNN made only 14 errors (meaning it was 94.6 percent accurate) compared to 17 made by the human testers (which made it only 93.9 percent accurate.) For the CNN, most of these errors were made when diagnosing tumors with similar structure and for the human testers, most of their errors were made by misidentifying malignant tumors and metastatic ones.
Interestingly, the team found that the human testers were able to correctly identify samples the CNN could not and that vice versa the CNN was able to identify samples the human testers diagnosed incorrectly. This result, Orringer tells Inverse, led the team to believe that using an A.I. in addition to a professional neuropathologist could be an effective way to provide accurate, real-time diagnoses during surgery.
“Based on these results, we suspect that the performance of even some of the most well trained pathologists (who read the cases in our study) could be improved with AI,” says Orringer. “More importantly, in under-resourced centers, it might be possible to use algorithms like ours to provide diagnosis during surgery independent of a traditional pathology laboratory.”
Additionally, while this study did focus specifically on brain tumors the researchers say this approach could be easily repackaged to help with in other fields that make similar post-surgery analyses, such as dermatology, head and neck surgery, breast surgery and gynecology.
Abstract:
Intraoperative diagnosis is essential for providing safe and effective care during cancer surgery. The existing workflow for intraoperative diagnosis based on hematoxylin and eosin staining of processed tissue is time, resource and labor intensive. Moreover, interpretation of intraoperative histologic images is dependent on a contracting, unevenly distributed, pathology workforce. In the present study, we report a parallel workflow that combines stimulated Raman histology (SRH)5–7, a label-free optical imaging method and deep convolutional neural networks (CNNs) to predict diagnosis at the bedside in near real-time in an automated fashion. Specifically, our CNNs, trained on over 2.5 million SRH images, predict brain tumor diagnosis in the operating room in under 150 s, an order of magnitude faster than conventional techniques (for example, 20–30 min). In a multicenter, prospective clinical trial (n = 278), we demonstrated that CNN-based diagnosis of SRH images was noninferior to pathologist-based interpretation of conventional histologic images (overall accuracy, 94.6% versus 93.9%). Our CNNs learned a hierarchy of recognizable histologic feature representations to classify the major histopathologic classes of brain tumors. In addition, we implemented a semantic segmentation method to identify tumor-infiltrated diagnostic regions within SRH images. These results demonstrate how intraoperative cancer diagnosis can be streamlined, creating a complementary pathway for tissue diagnosis that is independent of a traditional pathology laboratory.