A simple computer game could help identify opioid users' relapse risk
This game works by identifying how likely someone is to accept unknown risks.
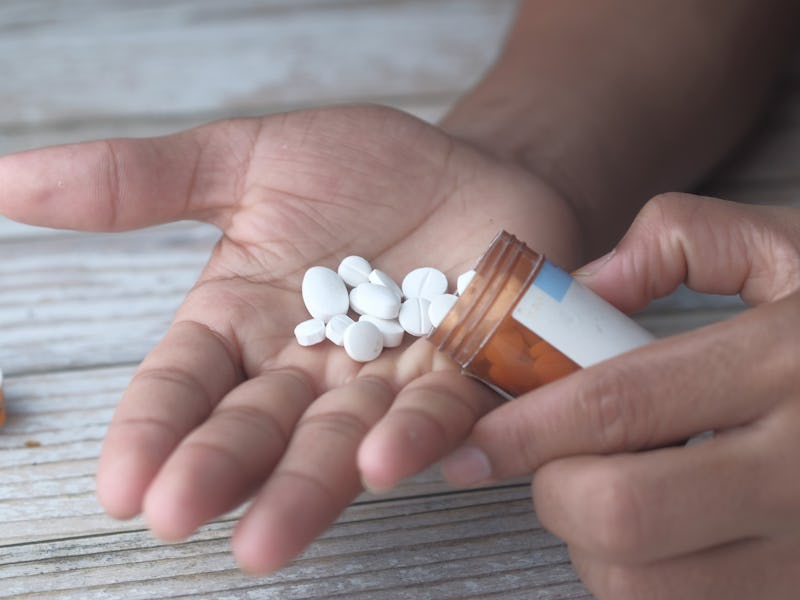
Opioid addiction is a public health crisis in America. In 2018, according to the U.S Department of Health and Human Services, a staggering 10.3 million people misused opioid prescriptions. Even when someone struggling with this addiction manages to get help and treatment, relapse is still incredibly common. But now a new approach to opioid addiction care could help clinicians predict relapse behavior before it happens.
While many clinical approaches to opioid addiction care focus on helping individuals after relapse or “slip-ups,” researchers of this study have used tools from computational psychiatry and decision neuroscience to preemptively identify when patients may be most likely to relapse. The study’s senior investigator and neuroeconomist, Paul Glimcher, told Inverse that this more accessible approach is extremely important for those with opioid addictions who don’t receive more consistent treatment and care.
“For patients receiving buprenorphine (sometimes called suboxone) therapy, it’s common for them to come to the clinic only once a month,” says Glimcher. “For those patients there is, I fear, very little monitoring. This seems a pressing point because buprenorphine, in part because these monthly visits make it so much cheaper, is rapidly growing in popularity.”
The study was published Sunday in the Journal of the American Medical Association (JAMA) Psychiatry and used a simple computer-based betting game to look at how participants’ willingness to accept an unknown risk matched with spikes in their opiate cravings. The researchers conducted this study at an addiction therapy center at NYC Health + Hospitals/Bellevue and studied 70 recovering opioid users as well as a control group of 55 participants who had never been addicted to opioids.
Participants completed up to fifteen sessions over a 7-month period, with more frequent sessions concentrated during the beginning of participants’ recovery when the risk of relapse was highest.
During these sessions participants first completed clinical assessments to determine their anxiety, craving, withdrawal, and adherence to treatment and then were asked to participate in a simple betting game to measure their current tolerance level of risky behavior. In the betting game, the participants were offered two different scenarios: one with known risk and one with unknown risk.
In the known risk scenario participants were offered either a fixed $5 pay-off or a lottery option with predetermined probabilities. But, in the unknown risk scenario, the probabilities were only partially known.
The researcher’s found that participants whose clinical assessments demonstrated higher levels of drug cravings were also significantly more likely to choose the riskier unknown bet. The study says that such participants would be 85 percent likely to reuse within the week.
Glimcher told Inverse that clinicians can do literally life-saving work with this preemptive knowledge.
“When clinicians see that a patient is having trouble [like this] they have a number of ways that they can help,” said Glimcher. “For patients receiving buprenorphine or methadone, which are really the gold standards for treatment, clinicians can adjust dosing. So if we see that a patient is struggling or at additional risk, giving a slightly higher dose of medication for that week can be a real lifesaver – literally. Clinicians can also suggest that patients attend a therapy session when they are struggling or patients on their own can do their best to avoid risky situations like hanging out with friends who use.”
The team is currently working on transforming this betting game into an easy to use app and while Glimcher says its primarily targeted at clinical environments, that there’s no reason it couldn’t be used as a public tool as well.
“As clinical researchers, we think of it primarily as a tool for use in a clinical setting,” says Glimcher. “But we are interested in whether patients and their families might be able to use this kind of information on their own. We are hoping to see what impact keeping family members informed of these risks can have in a future clinical trial.”
Read the study’s key points here:
Question: Can tools from computational psychiatry and decision neuroscience be used to understand and predict opioid reuse risk?
Findings: In this longitudinal study, we serially examined computational markers of risky decision-making in opioid users studied over 7 months of treatment. We found that an increase in an individual marker of tolerance to ambiguity (a context in which there is limited information about environmental risk) was significantly tied to imminent opioid use (within 1-4 weeks) in a manner statistically independent of standard clinical predictors.
Meaning: Computational markers of risky decision-making capture distinct latent cognitive processes with clinical utility for detecting opioid reuse vulnerability.