A.I.'s are being taught quantum mechanics to help speed-up chemistry
Say hello the SchNOrb. It could change chemistry.
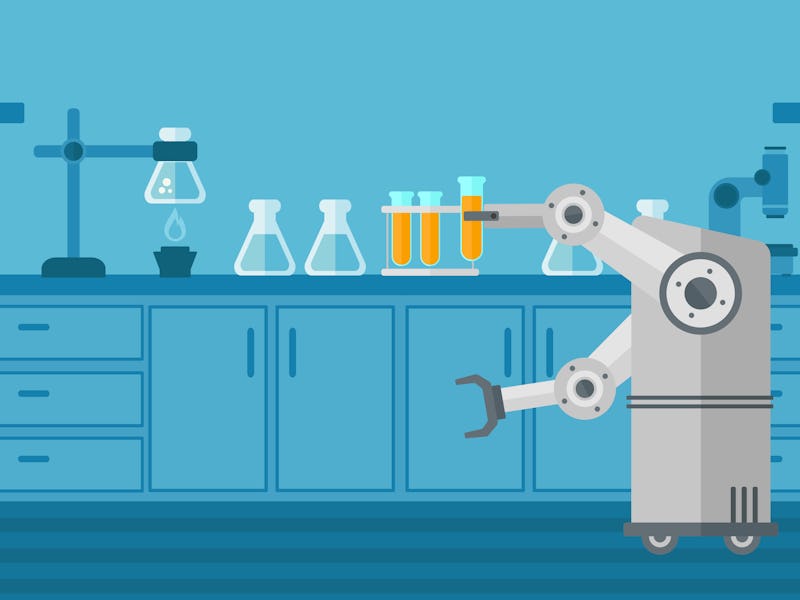
In the fast-paced, complicated world of quantum chemistry, A.I.’s are used to help chemists calculate important chemical properties and make predictions about experimental outcomes. But, in order to do this accurately, these A.I. need to have a pretty strong understanding of the fundamental rules of quantum mechanics, and researchers of a new interdisciplinary study on the topic say these quantum predictions have been lacking for some time. A new machine learning framework could be the answer.
While previous renditions of quantum-savvy A.I. algorithms have been useful, say the new study’s researchers, they have also failed to capture some of quantum chemistry’s most important characteristics in their prediction models. Namely, these previous models have neglected to account for electronic degrees of freedom in these trials which are the number of changing factors required to describe a specific state of a system.
Quantum mechanics, famously, allows for states to simultaneously exist and not exist, and using degrees of freedom can help scientists better understand how to accurately and usefully describe a system. Without accounting for these degrees of freedom, previous A.I.’s have described these quantum chemistry experiments in more classical scalar, vector and tensor fields, which required much more calculation time and energy.
Researchers of this new study have instead designed a framework that will describe them in the more quantumly accurate, and faster, form of ground-state wavefunctions. The study describing their approach was published last week in the journal Nature Communications.
One of the study’s authors, Reinhard Maurer from the Department of Chemistry at the University of Warwick, said in a statement that their algorithm’s combined flexibility and quantum know-how will help make it an important tool for quantum chemistry.
“This has been a joint three year effort, which required computer science know-how to develop an artificial intelligence algorithm flexible enough to capture the shape and behaviour of wave functions, but also chemistry and physics know-how to process and represent quantum chemical data in a form that is manageable for the algorithm,” said Maurer.
The study authors write that this deep-learning framework, called SchNOrb (which we can only imagine is as fun to pronounce as it looks), allows them to predict molecular orbits with “close to ‘chemical accuracy’” which in turn provides an accurate prediction of the molecules’ electronic structure and a “rich chemical interpretation” of its reaction dynamics.
The capabilities demonstrated by this algorithm would help chemists more effectively design “purpose-built molecules” for medical and industry use.
Quantum mechanics happens at the sub-atomic level, where standard rules that we take for granted in the visible world don't always apply.
However, while the authors write that SchNOrb is proof that such an application is useful and feasible, the large number of atomic orbitals its able process also leaves it vulnerable to increased prediction errors as well. The authors write that the accumulation of these prediction errors eventually led to a bottleneck in the prediction process — in many ways the same kind of efficiency error they were trying to improve from previous approaches.
In order to account for this problem, the authors write that in future studies they’ll need to learn more about and improve the neural network used in this study.
That said, the authors are still confident that this preliminary research demonstrates a path forward toward more effective collaboration between quantum chemists and these quantum-savvy A.I.’s, and that this collaboration will become an essential part of the discovery process in years to come.
>Abstract:
Machine learning advances chemistry and materials science by enabling large-scale exploration of chemical space based on quantum chemical calculations. While these models supply fast and accurate predictions of atomistic chemical properties, they do not explicitly capture the electronic degrees of freedom of a molecule, which limits their applicability for reactive chemistry and chemical analysis. Here we present a deep learning framework for the prediction of the quantum mechanical wavefunction in a local basis of atomic orbitals from which all other ground-state properties can be derived. This approach retains full access to the electronic structure via the wavefunction at force-field-like efficiency and captures quantum mechanics in an analytically differentiable representation. On several examples, we demonstrate that this opens promising avenues to perform inverse design of molecular structures for targeting electronic property optimisation and a clear path towards increased synergy of machine learning and quantum chemistry.