A.I. is electrified about helping protect you from lightning strikes
Lightning kills. Robots want to help.
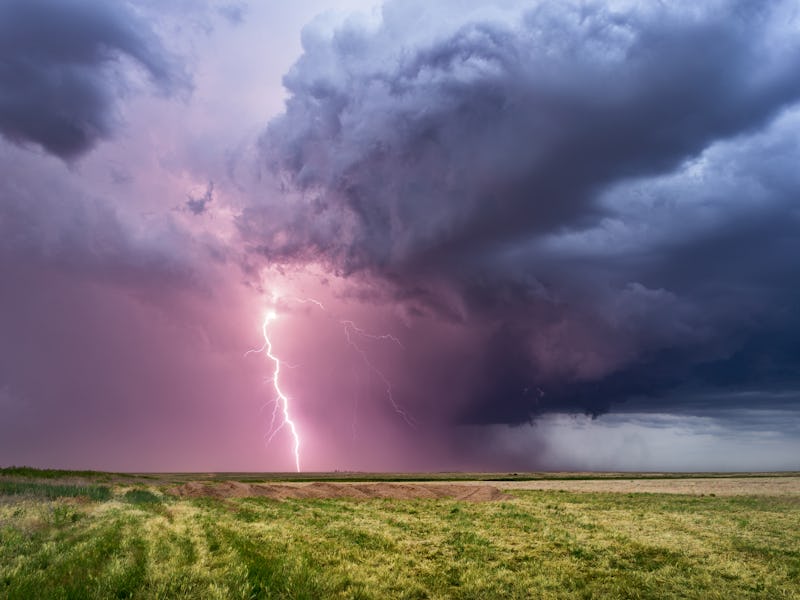
If you’ve ever been at a community pool during a thunderstorm warning only for the storm to never manifest, then you know how challenging it can be to accurately predict these dangerous and capricious storms. Scientists hope that they may be able to improve this process, and potentially save lives, through a lightning prediction algorithm that uses machine learning and atmospheric data.
The research was published Friday in the journal Climate and Atmospheric Science and demonstrates how a supervised learning approach, where the A.I. is trained on labeled data before being exposed to the real thing, could not only be effective at predicting these storms but could surpass previous prediction methods as well. Researchers looked at data from 12 Swiss weather stations in a variety of terrains between 2006 and 2017 and trained the algorithm to extract patterns from the weather data. Once trained on parts of this data the algorithm was able to make predictions about the other unlabeled data with an accuracy of up to 80 percent.
Amirhossein Mostajabi, the Ph.D. student who came up with the technique, said in a statement that this approach would enable them to make accurate predictions much faster than previous models.
“Current systems are slow and very complex, and they require expensive external data acquired by radar or satellite,” said Mostajabi. “Our method uses data that can be obtained from any weather station. That means we can cover remote regions that are out of radar and satellite range and where communication networks are unavailable.”
The machine learning algorithm designed in this study could predict lightning strikes 30 minutes in advance with 80 percent accuracy.
The algorithm looked at four different types of data, including atmospheric pressure, air temperature, relative humidity and wind speed. The algorithm drew connections between these parameters in order to make future predictions about impending lightning conditions in windows of 0-10, 10-20 and 20-30 minutes ahead of a storm.
Researchers compared their algorithm to manual methods such as persistence (“today equals tomorrow”), CAPE (convective available potential energy), and E-FIELD (electrostatic fields). In addition to widely matching the accuracy of these methods, the researchers write that an added benefit of their model is that specific radar data is not needed. That’s an added benefit for rural areas which might lack datasets on their lightning.
Despite its successes, the machine learning approach has drawbacks. The authors write that changes in environment, such as large buildings going up nearby, could throw off the prediction scheme. The researchers also struggled with an imbalance of predictions by the algorithm of “lightning inactive” and “lightning active” classifications, especially for further out predictions. To remedy this the authors write that the algorithm would need to have additional special techniques developed to correct it.
So, while these approaches show promise, for the time being you still should probably hop out of the pool when the thunderstorm warning goes off.
>Abstract:
Lightning discharges in the atmosphere owe their existence to the combination of complex dynamic and microphysical processes. Knowledge discovery and data mining methods can be used for seeking characteristics of data and their teleconnections in complex data clusters. We have used machine learning techniques to successfully hindcast nearby and distant lightning hazards by looking at single-site observations of meteorological parameters. We developed a four-parameter model based on four commonly available surface weather variables (air pressure at station level (QFE), air temperature, relative humidity, and wind speed). The produced warnings are validated using the data from lightning location systems. Evaluation results show that the model has statistically considerable predictive skill for lead times up to 30 min. Furthermore, the importance of the input parameters fits with the broad physical understanding of surface processes driving thunderstorms (e.g., the surface temperature and the relative humidity will be important factors for the instability and moisture availability of the thunderstorm environment). The model also improves upon three competitive baselines for generating lightning warnings: (i) a simple but objective baseline forecast, based on the persistence method, (ii) the widely-used method based on a threshold of the vertical electrostatic field magnitude at ground level, and, finally (iii) a scheme based on CAPE threshold. Apart from discussing the prediction skill of the model, data mining techniques are also used to compare the patterns of data distribution, both spatially and temporally among the stations. The results encourage further analysis on how mining techniques could contribute to further our understanding of lightning dependencies on atmospheric parameters.