This Neural Network Can Restore Any Damaged Photo to Its Pristine Original
Say goodbye to blurry selfies.
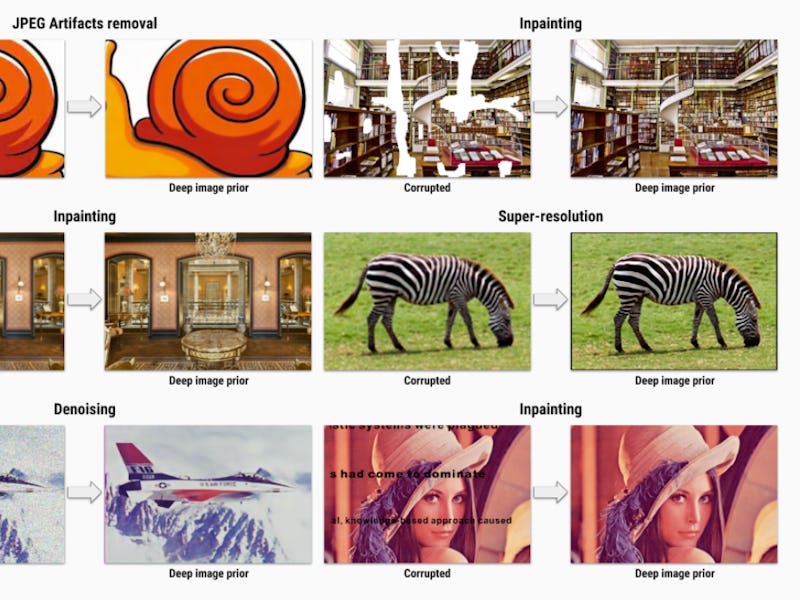
There’s nothing worse than opening an image on your computer only to find that it’s so grainy that you can’t even begin to make it out.
Some people might say get a better camera. These people are mean. But computer scientists — the good, helpful people — are saying use a neural network, a computer system designed to mimic the thinking of the human brain.
Three computer scientists from Oxford University and the Skolkovo Institute of Science and Technology in Moscow who specialize in computer vision have developed a neural net that can make that uselessly pixelated photo of avocado toast into an image that’s perfectly Instagrammable. They call it Deep Image Prior.
Neural networks are loosely modeled to resemble a human brain. They’re made up of thousands of nodes that they use to make decisions and judgments about the data being presented to them. Just like toddlers, they start off not know anything but after a couple thousand training sessions they can quickly become better than humans at everyday tasks.
Many neural networks are trained by feeding them large datasets, which gives them a huge pool of information to pull from when it comes to making a decision.
Deep Image Prior takes a different approach. It works out everything from just that single original image, not needing any prior training before it can turn your crappy, corrupted image back into a high-res shot.
The three computer scientists used a generator network to redraw blurry picture thousands of times until it gets so good at it that it creates an images better than the original. It uses the existing input as context to fill in the missing or damaged parts. Some of the results were even better than output from pre-trained neural networks.
“[The] network kind of fills the corrupted regions with textures from nearby,” said Dmitry Ulyanov a co-author of the research in a reddit post.
He admitted there are some instances where the network would fail, such as the complexity of reconstructing the human eye: “The obvious failure case would be anything related to semantic inpainting, e.g. inpaint a region where you expect to be an eye — our method knows nothing about face semantics and will fill the corrupted region with some textures.”
Aside from restoring photos, Deep Image Prior was also able to successfully remove text placed over images. Which raises the concern that this model could be used to remove watermarks or other copyright information from images online. A real-world possibility that perhaps went overlooked during this research.
This experiment proves that you don’t need access to a colossal dataset to create a functioning neural network. Beyond all the good this could do for your photos folder, that might end up being this project’s most lasting contribution.