A.I. Doesn't Get Black Twitter
The language of the internet doesn't follow the rules of English.
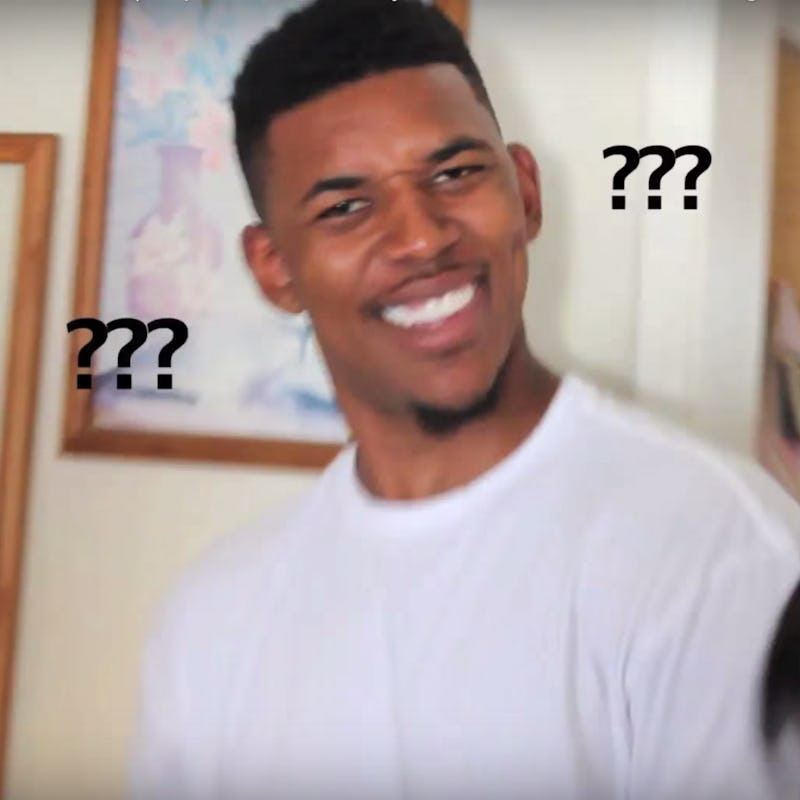
Approximately 8 of the 319 million people in the United States read the Wall Street Journal, about 2 percent of the population. If you look at the language — standardized English — being fed into many natural language processing units, it’s based on the language of that 2 percent. And many machines literally use the venerable, business-focused newspaper to better understand the English language.
It might seem like an obvious choice. Standardized English is taught in schools, it’s used in legal documents, and it sets the basis for formal society.
However, as Brendan O’Connor, assistant professor of computer sciences at the University of Massachusetts Amherst will tell you, the language of the internet doesn’t follow the rules of English. Yes, you can find the Queen of England on Twitter, but you can also find millions with dialects and languages from across the globe.
“It’s a little closer to speaking in some way,” O’Connor tells Inverse. “In spoken language, there’s always been much more diversity. In online writing, there’s no one teaching us how to use language on the internet or [in text messages].”
Enter Black Twitter
O’Connor, who has a Ph.D. in computer science with a specialization in natural language learning, teamed up with other researchers to study the use of African-American language online, examining 59 million tweets from 2.8 million users, collecting what they believe the largest data set to date. Black Twitter is the perfect test case, even if people don’t get it.
They went to the site because the Pew Center has shown to have a disproportionate number of black users. According to the Pew Center: “22 percent of online blacks are Twitter users.” Based on census data and Twitter’s geo-location features, the researchers used a statistical model that assumed a soft correlation between the language use and regional demographics.
With the help of Lisa Green, linguistics professor and director of the Center for the Study of African-American Language at UMass, they confirmed the patterns against known African-American dialect.
“I was always skeptical about if these speakers were actually from this community,” Green, who primarily focuses on primarily spoken dialects in South Louisiana, tells Inverse. However, in addition to the text correlating with modern slang, Green noticed patterns that were true to even African-American language in the 1950s and 1960. “That for me was really confirmation. It wasn’t just new slang but old patterns.”
“Some of the standard construction were really used in the ways you expected them to be,” she says. Speakers really tried to write it in ways that were true to pronunciation.
It’s that very hallmark of dialects, divergent from standard English, that cause natural language processors to fumble. Fed only articles from the Wall Street Journal, language from societal groups like Black Twitter isn’t seen by the machine as English at all.
When researchers evaluated their model against natural language processing tools, such as Google’s SynaxNet (“an open-source neural network framework”), researchers found that the software flagged African-American English as “not English” at a much higher frequency than standard English. In Twitter’s own language identifier, identification based on African-American language was twice as bad, despite the large presence of African-American users on the site.
“The standard tools being developed work worse on dialectical English,” O’Connor says. “Google’s parsers are going to do a worse job of analyzing it so it could be a case that our search systems might be worst at finding information from African-Americans. Language identification is the problem of you have a document and want language. This is a really crucial task. Your search engine only shows results written in English.”
This means that blogs or websites that employ African-American language could actually be pushed down in search results because of Google’s language processing.
O’Connor isn’t the first computer scientist to partner up with a linguist to shape his AI, but the extent to which their expertise is employed varies.
“There’s always a question of how much linguistic knowledge we need to build into our system,” says O’Connor.
While Green didn’t have prior experience in A.I. research, she saw the project as an important way of understanding how A.I. systems can become more diverse and incorporate more diverse players.
“As a linguist I’m always interested in language. There’s always been a question of the extent to which a computer can learn language,” she says.
“It’s always good to go for homogenous texts that are clean, you’re safe that way, but you’ll leave out a whole chunk of the population,” says Green.
As artificial intelligence increasingly becomes a staple of everyday life, diversity in the field becomes increasingly crucial in making sure that A.I. reflects the world it aims to serve.
O’Connor’s team isn’t the first to have noticed problems with existing natural language processing systems. Earlier this summer researchers at Boston University and Microsoft Research pointed out that Google’s neural network models, word2vec, create word embeddings based on datasets that promote sexist stereotypes. For instance, where the network would associate Paris: France, Japan: Tokyo, it would make similar associations between Father:Doctor and Mother:Homemaker.
“If the input data reflects stereotypes and biases of the broader society, then the output of the learning algorithm also captures these stereotypes,” reads an abstract for the paper. “As their use becomes increasingly common, applications can inadvertently amplify unwanted stereotypes.”
Diversity has become a question for all artificial intelligence systems, not just natural language learning. Any English-speaking Siri user with a non-American accent can tell you that even the most sophisticated voice processing still lags for some.
Olga Russakovsky, a post-doctorate researcher at Carnegie Mellon University, says that visual learning systems also have to combat the biases that are inherent in limited data sets.
“We talk about this all the time. When we analyze sort of closed-world sets of pictures, this represents biases of a visual world,” she tells Inverse
Russakovsky says that the key is to acknowledge the limitations of data sets and not making too broad of claims about the results.
“In the age of designing systems with big data, of course the type of data you put in will determine what it learns,” says Russakovsky. “Kind of like when you have a person who grows up with a certain set of experiences that what they learn.”
Projects like O’Connor’s are one way to combat limited data sets, but that kind of triage might not be necessary if preventative measures take place.
“Diversity in terms of people and research that gets done and how these issues are actually very intertwined,” says Russakovsky.
That’s why Russakovsky co-founded the Stanford Artificial Intelligence Laboratory’s Outreach Summer. The program sought to increase interest in A.I. amongst 10th-grade girls by contextualizing the social impact of A.I. A study after the program showed that the program statistically increased significant technical knowledge, interest in pursuing careers in A.I., and confidence in succeeding in A.I. and computer science amongst the girls.
“The way we develop A.I. comes from the way we teach A.I.,” says Russakovsky.
Russakovsky says A.I. is normally taught technology-first, application-second which can deter students who might be interested in things like the humanistic and societal impacts.
“This is becoming a huge part of our society”
Instead, the Stanford program focused on things like doing disaster relief through analyzing tweets.
“This is becoming a huge part of our society; we’re moving toward a world where A.I. really can transform so many different aspects of our lives. So if all we talk about is autonomous driving which is a great application there’s going to be tons of people who maybe don’t get excited about A.I.,” says Russakovsky. “That paradigm only appeals to the certain fraction of people.”
But until the percentage of minority employees in companies like Google and Facebook grows past its current rates, which hover around 2 percent, researchers like O’Connor have a long way to go in making sure discrimination gets noticed by the companies shaping the future of A.I.
“We talk to companies all the time,” say O’Connor. His lab is considering creating a software down the line to help companies account for the language discrimination his team unearthed.