Katharina Volz is using A.I. to solve the biggest problem in science
“The way we solved disease before ... that just won't work anymore.”
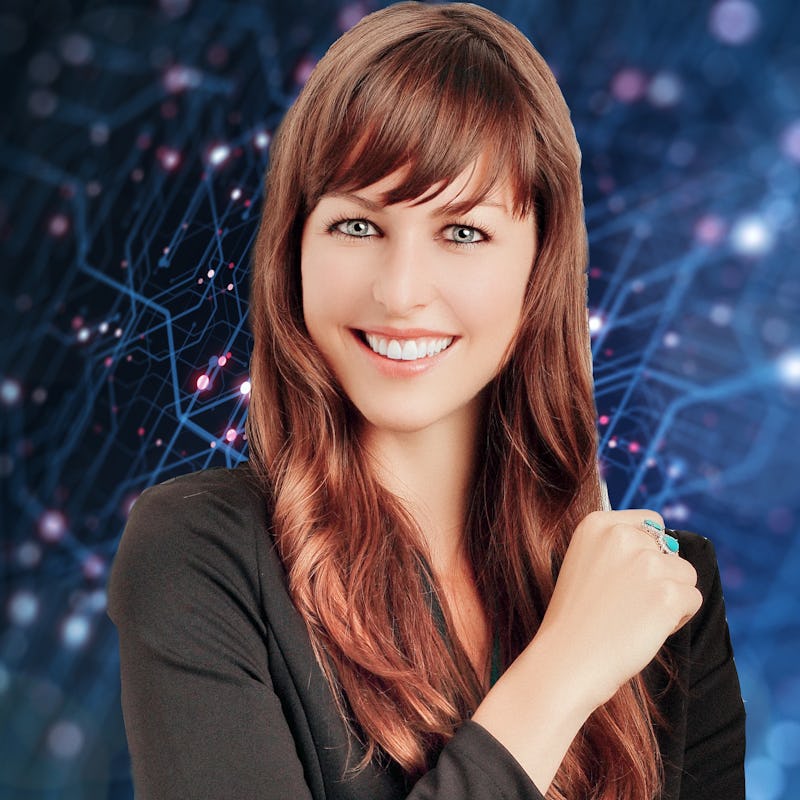
Katharina Volz is applying machine learning to finding and developing cures for brain-aging diseases, one of science’s biggest conundrums. First up on their list: Parkinson’s disease.
Born in Ulm, Germany (the birthplace of fellow scientist Albert Einstein), Volz was the first-ever Ph.D. in stem cell biology and regenerative medicine at Stanford University. Entrepreneurship did not come easily to Volz, a trained scientist. But a dogged passion for unearthing a cure for brain-aging disease is what pushed her to persevere. As the founder and CEO of OccamzRazor, a biotechnology firm, she's set out to treat a disease that hit close to home.
To solve this monumental problem, the company has developed algorithms that mine important information from already available scientific papers and clinical data — a task far too large for humans to ever do by hand. The result is a 3D graph, called the Parkinsome, which maps out everything we know about Parkinson’s today, revealing potentially crucial insights that would have most likely gone undiscovered.
Volz appeared on Forbes’ 30 under 30 list in the science category in 2017, and this year, MIT’s Technology Review picked her as one of their 35 Innovators Under 35. Investors in OccamzRazor include Jeff Dean, the head of A.I. at Google, and Justin Rosenstein, the co-founder of Asana.
The following interview, edited for clarity and brevity, is part of Inverse’s FUTURE 50 series, a group of 50 people who will be forces of good in the 2020s.
The motivation to found OccamzRazor came from learning that someone you knew had been diagnosed with Parkinson’s disease. Do you believe the best innovations come from a personal place?
When this person very close to me was diagnosed with Parkinson's, I knew that I could do something about it; I felt deeply responsible. Having a personal reason for innovation, I think, really does help a lot, particularly for staying persistent.
Things that have never been done before or that have failed, they’re not easy — or else they would have been already done. Particularly trying to solve a disease where 100 percent of clinical trials before failed. Of course, there are some symptomatic treatments for Parkinson’s, but nothing that is actually stopping or reversing disease progression.
Going through the ups and downs that every startup has, I always remind myself that there's no way in this world I would ever consider giving up. And if you have this mindset, you will find a solution.
Did you always want to pursue science?
I didn't know that I would become a scientist when I was little, but I was definitely the curious type. I had difficulty memorizing things at school because I didn’t want to just learn something off; I always wanted to know the why behind it.
What really excited me about science was all the questions that we didn’t know the answer to. I really found my love for science when I attended a new biotechnology high school in Germany.
As a child, there was always a part of me that was fearless. When I saw tricks at the circus, I repeated it at home — and of course, that often didn't go that well and I would have to go to hospital. [Laughs.] I was also actually pretty sick as a child and teenager, and so that really made me empathize with patients, too.
“There's no way in this world I would ever consider giving up.”
What’s the best compliment you've ever received?
It’s funny, actually, being from Germany, because German culture is not one where you get many compliments. In fact, it was only when I went to Harvard Medical School for research, I received what I think was the first compliment I’d ever gotten in my life. My mentor, this wonderful lady, told me she thought I did a phenomenal job on an experiment. And I remember asking my friend, “What do you say if somebody says something like that to you?” I really didn't know.
How did you respond? Did you say thank you?
[Laughs.] I think I was just so perplexed. I don’t think I said anything.
What was the experience of founding a startup like?
It was really, really, really tough.
I mean, I'm a scientist — I didn't even know the English terminology for startups. Maybe I was naive or crazy, but I just jumped into it. Looking back, that was probably a good idea, because if I had known everything that I know now, it might’ve been too scary. But, oh gosh, I made a lot of mistakes.
The most difficult part was getting a team together. It wasn’t just a case of bringing in the most intelligent or hard working people. For instance, we had to have machine learning scientists who could speak the language of computational biologists and the pharmaceuticals industry — basically, an entire range of disciplines. And then for me, not actually knowing how to evaluate engineering or machine learning, because that's not my background, made it difficult.
Was there any time you feel you really messed up?
I mean, all the time. I guess that’s the nature of a startup.
The first two years, there were so many mistakes that I made. Luckily, I had incredible advisors and mentors who were very kind and patient and didn't give up on me.
I actually had to exchange the entire team after one year because it ended up not being the right team. That was really difficult, and also kind of a breaking point for the company.
Unless you have a team that really can solve that problem, it's going to be really hard. And, particularly for what we’re trying to do, it's going to be impossible.
We had to figure out how to get the top talent on board, in areas like machine learning and computational biology and neuroscience and drug development, if we were to be able to solve this problem. At the beginning, we couldn't actually hire a machine learning Ph.D. because they’re obviously in such high demand.
So, what we did is we created a dataset that we knew how to do really well, and we approached the A.I. labs at Stanford University, where we showed them this dataset. And this was how we really jump-started things: They helped us to spring off the ground. With their help, we published the first version of our machine learning platform.
How has the company shifted during the pandemic?
We are actually lucky in that we’ve already been distributed between New York and San Francisco. And so, we already had all of our processes set up remotely and had a great routine in place. Our partner lab that had been doing our validations shut down, so we did have to figure out a way of how to deal with that.
But we’re now turning into a fully distributed company, because we realized we’re actually even more productive.
“I absolutely believe this is solvable.”
Has the pandemic made you think differently about the future of science?
On one side, biology and medicine are needed now more than ever; we suddenly see that a virus or a disease can affect the entire world. One of the beautiful things about what happened because of the pandemic is researchers and institutions working together in a way that they have never done before.
In my field, we know that if we encounter a new disease, it's very difficult to start from scratch. But with a virus like Covid-19, it’s a little simpler than others. We know where to target, that there are different entry points in the body where the virus can go in, and therefore, we know how to hijack the virus.
For neurodegenerative diseases like Parkinson's, however, we don't know where to hijack. They’re actually by an order of magnitude more complex. I’m hopeful people will dedicate as much resources and time into solving these diseases as they did with Covid-19.
The world is aging as a population; the incidence of brain-aging disease is significantly increasing, and there are no treatments that stop or reverse brain degeneration. And we need to make it as high a priority as other diseases, like Covid-19, if we’re going to solve them. The effect on the world and the economy and the lives of people and families will be traumatic if we don’t.
What’s the plan for the next few years? Is the company planning to take the approach beyond Parkinson’s?
We are focusing on Parkinson's currently. However, the way we built the platform means we can scale it to any different type of disease. So, data from diseases such as Alzheimer's or multiple sclerosis, or ALS informs our understanding of Parkinson's, and the other way around, too.
Next on our agenda is running clinical programs to get treatments to patients as quickly as possible. For example, one of the things we’re doing is looking into compounds that may be sitting on a pharma company’s shelf, that may have passed some of the clinical trials already, but maybe have failed for efficacy reasons. And we want to see if we can potentially repurpose those drugs.
When it comes to neurodegenerative diseases, it feels like one area of science where little progress has been made. Do you think the end is in sight?
I absolutely believe this is solvable.
We’ve been working with the old-fashioned paradigm for so long to solve these complex and new diseases. The way we solved disease before, through very linear thinking, that just won’t work anymore. I mean, 70 percent of clinical trials for neuro drugs fail at the second stage of clinical trials.
I'm so hopeful that by using machine learning and taking all of the expert knowledge to combine it into one big map, we’ll be able to see how everything connects and what is actually going wrong. Once we do that, we’ll just need to know where to actually repair.
And that is why I believe we can solve neurodegenerative diseases; we just haven't looked at it in the right way.
Katharina Volz is a member of the Inverse Future 50, a group of 50 people who will be forces of good in the 2020s.