A.I. system SPOCK determines which planets live or die
What stops planets from crashing into each other?
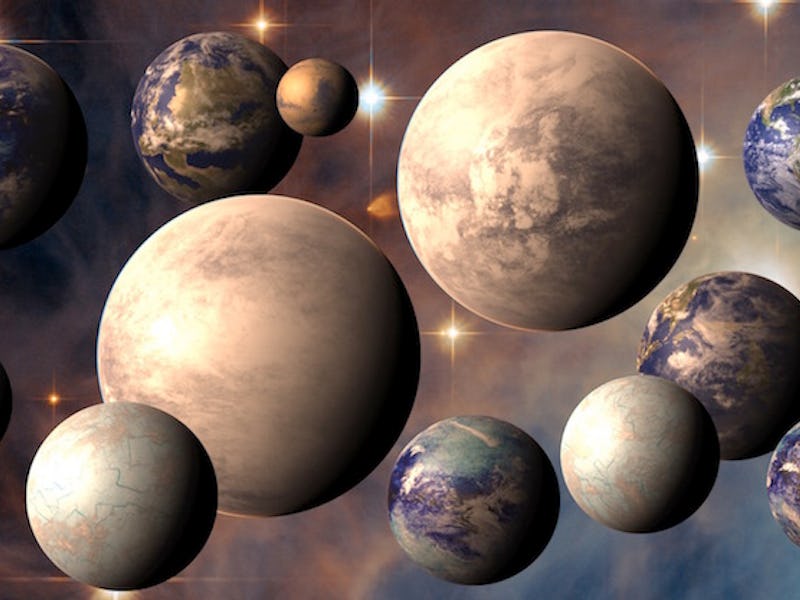
When it comes to cosmic survival, it's not only the survival of the fittest but also the most coordinated.
As planets orbit around their common host star, astronomers have often wondered what keeps them from crashing into each other while in orbit. However, they failed to determine what makes for orbital stability, and what makes planets like the ones in the Solar System survive the test of time, and gravitational pull?
A team of researchers may have found the answer, developing an artificial intelligence system aptly named SPOCK (or Stability of Planetary Orbital Configurations Klassifier) which determines which star systems will fail and which ones will live long and prosper.
The study was published this week in the Proceedings of the National Academy of Sciences journal, and provides astronomers with helpful insight when trying to find worlds outside of the confines of the Solar System.
Planets are born from clouds of dust, gas and rock that encircle a young star. For multi-planetary systems like our own Solar System, there are many things that could go wrong in terms of how they orbit around their host star.
There are many orbital configurations that are deemed unstable, and will likely spiral into quick doom as planets cross over each other's orbits in a matter of millions of years.
Daniel Tamayo, a NASA Hubble Fellowship Program Sagan Fellow in astrophysical sciences at Princeton, and lead author behind the new study, said in a statement that the problem was "brutally hard":
"Separating the stable from the unstable configurations turns out to be a fascinating and brutally hard problem."
An illustration that shows two possible orbital configurations for the Kepler-431 system, with the left-hand image showing unstable orbits of the three planets.
Astronomers have had to observe planetary systems for billions of years, calculating the motions of the planets around the star and determining the possible configuration for stability.
However, the team behind the new study turned to super computers to simplify this long and tedious process by combining simplified models of the planets' interactions, and machine learning.
The process begins by simulating 10,000 orbits and calculating 10 summary metrics of the planetary system's dynamics. And then, in comes SPOCK.
The A.I. system predicts which of these 10 features would have a stable future if it kept on going, up until the planets orbit around their common host star for about one billion times.
"We can't categorically say 'This system will be OK, but that one will blow up soon,'" Tamayo said. "The goal instead is, for a given system, to rule out all the unstable possibilities that would have already collided and couldn't exist at the present day."
SPOCK is speedy, operating at 100,000 times faster than the traditional method of determining the orbital stability of a planetary system.
By speeding up this process, astronomers get a better understanding of what are some of the factors that lead to a system's speedy failure. By doing so, astronomers are able to identify the composition and properties of exoplanets, or planets that exist outside of our Solar System.
"This new method will provide a clearer window into the orbital architectures of planetary systems beyond our own," Tamayo said.
Some exoplanets are too small to directly observe by a telescope, and it is therefore difficult to determine their orbits around their host stars. However, this new method establishes models of stable planetary orbits that could be applied to these faint, distant worlds.
Abstract: We combine analytical understanding of resonant dynamics in two-planet systems with machine learning techniques to train a model capable of robustly classifying stability in compact multi-planet systems over long timescales of 109 orbits. Our Stability of Planetary Orbital Configurations Klassifier (SPOCK) predicts stability using physically motivated summary statistics measured in integrations of the first 104 orbits, thus achieving speed-ups of up to 105 over full simulations. This computationally opens up the stability constrained characterization of multi-planet systems. Our model, trained on ≈100,000 three-planet systems sampled at discrete resonances, generalizes both to a sample spanning a continuous period-ratio range, as well as to a large five-planet sample with qualitatively different configurations to our training dataset. Our approach significantly outperforms previous methods based on systems' angular momentum deficit, chaos indicators, and parametrized fits to numerical integrations. We use SPOCK to constrain the free eccentricities between the inner and outer pairs of planets in the Kepler-431 system of three approximately Earth-sized planets to both be below 0.05. Our stability analysis provides significantly stronger eccentricity constraints than currently achievable through either radial velocity or transit duration measurements for small planets, and within a factor of a few of systems that exhibit transit timing variations (TTVs). Given that current exoplanet detection strategies now rarely allow for strong TTV constraints (Hadden et al., 2019), SPOCK enables a powerful complementary method for precisely characterizing compact multi-planet systems. We publicly release SPOCK for community use.
This article was originally published on