Watch this A.I. build fake faces that look entirely real
"Enhance!" is a standby of '90s TV shows. Now, a university team can create realistic faces out of blurry images. Except they're fake.
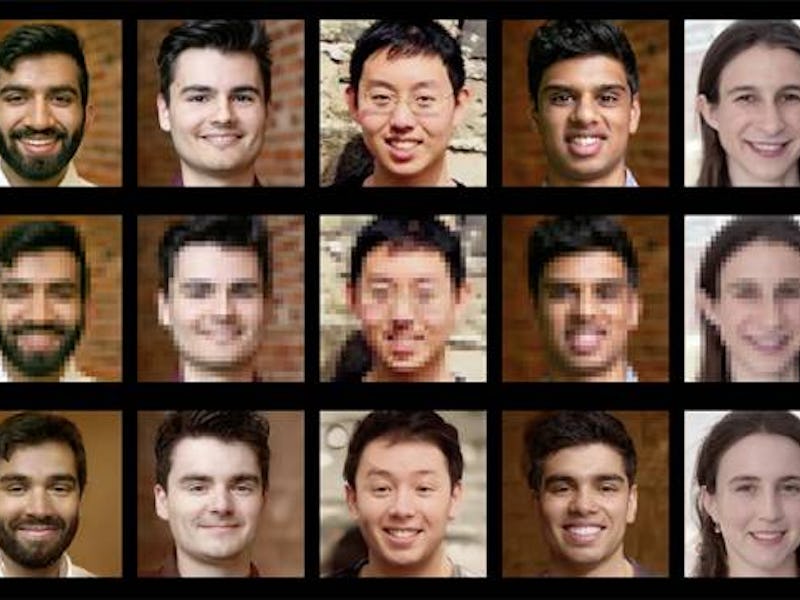
It’s the oldest trope in any crime-fighting TV show: a grizzled detective looks over a screen and barks out, “Enhance!” Suddenly, a once-blurry suspect comes into focus, making identification very easy and allowing the plot to continue. Famously, this is not how cameras work. They still don’t, but researchers at Duke University have developed an A.I that can turn blurry photos into new faces of people who don’t exist.
What’s the good in that? It’s important to understand that this new method of visualization, PULSE, likely won’t be used in the real world to create fake faces. Faces are just proof-of-concept, and PULSE can create low-res shots of almost anything and create sharp, realistic-looking pictures, ranging from astronomical phenomena to human organs. The details that change in the faces include stubble, fine lines, and eyelashes. So while the photos couldn’t be used to identify someone, they create very realistic people that come close.
Cynthia Rudin, a computer scientist at Duke who led the PULSE team, noted the method’s ability to create highly detailed images.
“Never have super-resolution images been created at this resolution before with this much detail,” she said in a press statement.
How the A.I changes a photo.
PULSE, which stands for Photo Upsampling via Latent Space Exploration, is detailed in a paper that the team has placed on pre-print depository arXiv. There, they detail how PULSE can take a 16 x 16-pixel image of a face, one where facial features are barely detectable, and rush in with millions of added pixels, creating 1024 x 1024-pixel images akin to high-definition resolution. It’s similar to watching a Nintendo SNES game suddenly turn into a Playstation 4 hyper-realistic game, except all the faces have changed.
Co-author Alex Damian, a Duke math major, notes that even when a person could barely identify facial features in a photo, “our algorithm still manages to do something with it, which is something that traditional approaches can’t do.”
The authors of the study, with their faces transformed through PULSE.
What explains the big improvements? For the Duke team, it was a matter of finding better source material. Most A.I attempts at photo enhancement do their best to “guess” at specific sections of a photo, like hair or teeth. A traditional replacement can result in photos that look a little off, with textured hair or skin that doesn’t quite line up.
That’s why the Duke team decided to use what’s known as a “generative adversarial network,” or GAN. First developed in 2014, a GAN essentially sets up a game between two competitive neural networks. One of these networks is known as a generative network, which creates images sometimes based on images, sometimes made out of thin air. The other is called the discriminator. The game evolves as the generative network consistently tries to fool the discriminator, hopefully getting better and better with each attempt.
In this case, the generative network was coming up with human faces based on the blurry photos, and the discriminator was determining if they were convincing enough to be real. Eventually, the generator won.
There are certainly privacy concerns with an A.I like PULSE, although researchers say it cannot be used to identify people due to its nature of adding in identifying features. However, full accuracy isn't exactly a requirement in current standards of security, as the use of the police sketch shows. There, recent studies have shown, even half-an-hour in delaying descriptions can radically alter how a person remembers a face. Humans holistically remember faces, as opposed to remembering individual sections.
So while PULSE couldn't give someone an exact replica, it's certainly possible that it could help push someone in a certain direction.
ABSTRACT: The primary aim of single-image super-resolution is to construct a high-resolution (HR) image from a corresponding low-resolution (LR) input. In previous approaches, which have generally been supervised, the training objective typically measures a pixel-wise average distance between the super-resolved (SR) and HR images. Optimizing such metrics often leads to blurring, especially in high variance (detailed) regions. We propose an alternative formulation of the super-resolution problem based on creating realistic SR images that downscale correctly. We present a novel super-resolution algorithm addressing this problem, PULSE (Photo Upsampling via Latent Space Exploration), which generates high-resolution, realistic images at resolutions previously unseen in the literature. It accomplishes this in an entirely self-supervised fashion and is not confined to a specific degradation operator used during training, unlike previous methods (which require training on databases of LR-HR image pairs for supervised learning). Instead of starting with the LR image and slowly adding detail, PULSE traverses the high-resolution natural image manifold, searching for images that downscale to the original LR image. This is formalized through the “downscaling loss,” which guides exploration through the latent space of a generative model. By leveraging properties of high-dimensional Gaussians, we restrict the search space to guarantee that our outputs are realistic. PULSE thereby generates super-resolved images that both are realistic and downscale correctly. We show extensive experimental results demonstrating the efficacy of our approach in the domain of face super-resolution (also known as face hallucination). Our method outperforms state-of-the-art methods in perceptual quality at higher resolutions and scale factors than previously possible.