Graphene May Hold the Key to Connecting Our Brains to Machines
With huge benefits for neural prostheses, learning, and Parkinson's.
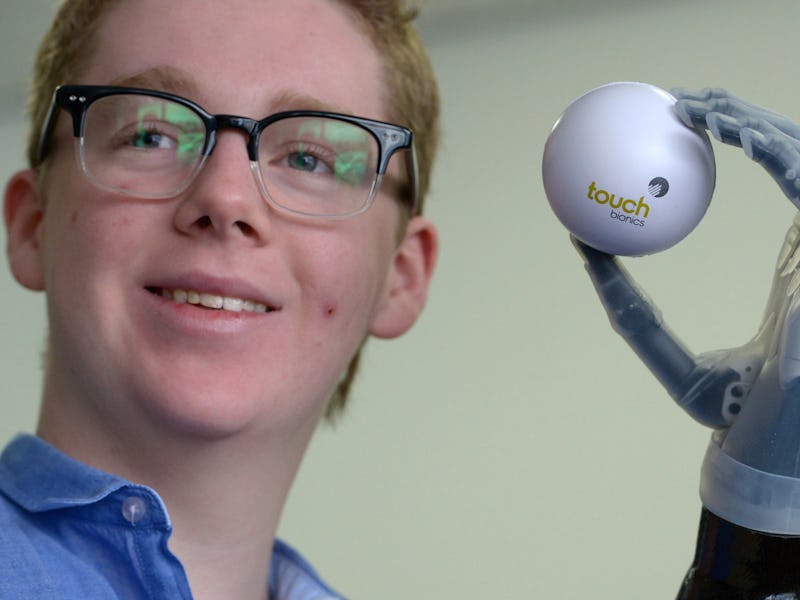
Your brain is a hive of electric activity — signals firing, data streaming. It’s also a black box of total chaos. Till now, the best ways to hook your neurons to devices and computers has been able to only survey wide swaths of neurons and take a broad consensus as to what they’re driving at. But an apparent breakthrough in graphene tech offers hope that we might be able to tap into individual neural signals in an existing biological setting, with huge ramifications for protheses, learning, and the preservation of mental health.
A team of researchers from Spain, Italy, and the UK have demonstrated that graphene can successfully interface with neurons, and carry an electrical signal from them. This work builds on previous efforts in which graphene was coated with peptides to promote neuronal adhesion, and showed that such coating is unnecessary. Unlike previous attempts and other technologies, this work did not trigger scar tissue, which, over time, has rendered other implants useless. Too, this version using untreated graphene has a high signal-to-noise ratio that makes it more practical for biological applications.
The first goals for this work are as a treatment for Parkinson’s. Existing neural interface technologies read the output of a neuron and translate it into something else. By directly interfacing with neurons, it’s hoped that this work can be used to interfere with the signal. Since Parkinson’s is a failure to inhibit neural signals, a technology that can artificially block extraneous signals might solve this problem. It’s thought that this is how existing implantable electrodes work: by non-specifically broadcasting electrical impulses that interfere with these inappropriate signals. Individual neuron resolution might provide far more control.
Graphene is an ideal material for biological interface: It’s flexible, stable, and biocompatible. Because it’s also able to carry an electrical charge, it has piqued the interest of researches for use in neural applications.
Existing neural interface technology tends to evaluate an entire field of neurons by using an array of electrodes (such as the recent example that was used to control individual fingers). Though this can be useful in some settings, it can be tricky to sift through the output of many, many neurons to find the signal you want. But get down to the resolution of interfacing with individual neurons, and the potential is unprecedented control — with all kinds of potential for neural prosthesis.
You still need a sophisticated mechanism to ensure that only the appropriate neurons are contacted; you must disentangle which signal is coming from where; and you have to translate this cacophony of signals.
Implanting the electrodes may also be tricky. Existing technologies plunge electrodes into brain tissue and almost certainly damage certain connections along the way. Because this technology is only concerned with field recordings, the damage of a few neurons is not problematic. If the goal is to interface with individual neurons, this could be a significant issue.
Further, the system might need to be “calibrated.” Timing and strength of neural signals are critically important. Normally, your brain calibrates itself. When you practice swinging a baseball bat, for example, you send feedback, positive or negative, to reinforce connections and use just the right amount of force and direction. If you had to manually adjust these things in a system that doesn’t self-correct, it could make things more challenging. (It’s worth noting that the brain is very good at being “plastic” and adapting, though, so it might solve its own problem by simply modulating its own output based on your reactions.)
These types of problems are engineering problems, though, and not impossible to solve. Once these challenges are solved, the ability to interface with individual neurons could be profound. For example, “coincidence detectors” in your brain detect incoming neural impulses from more than one neuron. If the timing of the input from both is close enough, it will trigger an impulse in the coincidence detector itself. This mechanism is used is several contexts, one of which is in learning.
Because this mechanism is great at associating different neural events, they can be used to build concepts that bridge distant parts of the brain together, and to therefore learn a new idea. If this process could be manually controlled, then one can imagine a Matrix-esque style of learning, in which the coincidence detectors are manually triggered to associate different concepts and to build a thought without ever setting foot in a classroom. In the short-term, though, simply blocking inappropriate signaling in Parkinson’s will be far less difficult. Look for graphene to preserve smooth movements first — before perhaps making memories easier to acquire later.