Georgia Tech's Stereotyping Robot Is the Future of AI, Not Racism
Still, it has got to learn that not all firefighters have beards.
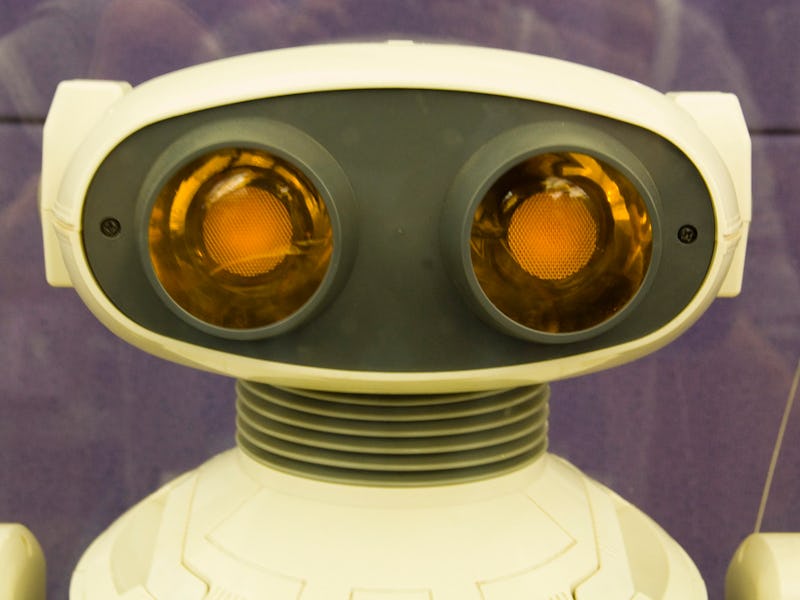
To ears sensitized by after-school specials and diversity seminars, this is going to sound bad, but we want robots to make quick judgments based on appearance. Overcoming prejudice is good, but the inability to stereotype diminishes intelligence — artificial and otherwise. Alan Wagner, Ph.D., a roboticist at Georgia Tech, is the chief proponent of stereotyping technology. He argues that this sort of logic doesn’t need to be applied to race or gender, just situations and behaviors.
In an early test of his stereotype algorithm, Wagner trained a naive robot to make conclusions from what it saw. The robot learned and became perceptive, which allowed Wagner to start thinking critically about the ethics of robot assumptions, especially the pre-programmed ones. He spoke to Inverse about his work and its ramifications.
Walk me through how the experiment worked.
The robot interacts with different types of individuals — firefighter, EMT, or whatnot — but it has no prior experience with any of these categories of individual. It is, basically, experiential learning.
The idea was to show that the robot could use perceptual features from the individual to predict their needs in terms of tool use. The way the algorithm worked, the robot’s camera would perceive different aspects of what the individual looked like — their uniform color, for example, whether they had a beard, and their hair color.
It would also ask them questions about what they look like. Of course, asking questions is not what you want to do in the field, but the robot’s perception is so limited right now. We needed a way to bootstrap the process about learning about a person. The person would select the tool, and then the robot would select the tool, and over time the robot would learn what tool each type of person preferred.
Did you expect the robot to learn that a badge means police officer or a heavy reflective coat means a firefighter?
We kind of expected it. But there were some surprising things, too. For example, the robot falsely recognized that a beard is predicted with a firefighter — that was odd, but when you look at the data, it wasn’t surprising. The first several people that interacted with it were firefighters that had beards. So we argue a need for perceptual diversity, an idea that if the robot could see large, broadly different types of individuals in a category, it would better develop and understand the category.
Would you say autonomous robots should be trained to iron out these quirks, so a robot won’t think if this person has a beard, he’s a firefighter?
Absolutely. It’s critical that we iron out these things. It’s critical that we have these robots that work from a diverse set of individuals.
What might that learning look like?
It would allow the robot to focus on things that better characterize firefighters. For example, a firefighter might not even be wearing a jacket. The robot would then notice other aspects of firefighting, perhaps the boots, perhaps the gloves, perhaps helmets. It would say, “OK this person really is a firefighter in this environment.”
If you had enough people, it might be able to recognize a firefighter at a fire versus a firefighter at a Halloween party. It’s subtle perceptual details, like the difference between the quality of the types of uniforms, or contextual environments.
Besides associating beards with firefighters, how successful was this algorithm?
There were two things we really wanted to look at: One, what can you do with it? If robots can recognize firefighters, does that really help in some way? The paper showed that it allowed you to narrow your search. Instead of looking at beards for hair color, looking for eye color or whatever else you might look for, you could focus on the features that really mattered. Is the person wearing a firefighter coat? That could speed up the process.
Another really critical thing that we looked at is, what if the category that the robot predicts is wrong? How does that impact you? You can imagine search and rescue environments can be chaotic: You maybe work in smoke-filled conditions, the robot may not be able to perceive everything very well, it might have errors. You could imagine a worse case, where the robot thinks the person is a victim when in actuality they’re a firefighter. So it’s trying to save a firefighter. That would be terrible. We wanted to see where it breaks, how it breaks, what features impact it the most and what are the different kinds of errors.
You can use this approach in different ways — if they can’t see the person at all, but can see the actions that they’re doing. If I can see the person selecting an axe, then I can predict they have a helmet.
How do you approach getting a robot to assess context and make a prediction?
We’ve tried to look at a couple of different types of environments — a restaurant, a school, and a nursing home. We tried to capture features about the environment and what objects are in the environment, what actions the person is selecting, and what the people in the environment look like, and try to use that to make a lot of social predictions. For example, in a school environment, people raise their hands before they talk. So if I see the action that people are raising their hand, what type of objects would I expect to see in the environment? Do I expect to see a chalkboard; do I expect to see a desk? I’d expect to see children.
The hope there is to use this information. If the robot is performing an evacuation procedure, it would see what types of people are there and where they might be.
Let’s say there’s a robot that comes to your door and says, “Please follow me to the exit.” Something as seemingly simple as that is actually very complex. If a robot knocks on a door in an apartment building, you have no idea who you’re going to interact with. It could be a four-year-old child, it could be a 95-year-old person. We’d love for the robot to tailor its interactive behavior to the type of person it sees in order to rescue them. We’re taking some of these contextual lessons and trying to develop that application.
Do you use a similar definition of “stereotype” for robots and humans, or is there something else going on?
The term stereotyping has a negative context. The way we’re using it is simply to develop categories of people, and use categorical information to predict characteristics of a person. I know in psychology, a lot of work focuses on facial stereotypes and gender stereotypes. We’re not doing anything like that. Is the process the same? I don’t know. No idea.
Are you worried people might have misconceptions about your work?
A couple of years back, we developed this idea of robots that could deceive people. In the media there was a bit of a misperception that this would lead to robots stealing people’s wallets.
I’d like to use the emergency evacuation situation: You don’t always want to be entirely honest with a person in an evacuation, right? For example, if someone asked you, “Is my family all right?” It could be terrible if the robot said, “No, they all died. Please follow me to the exit.” There are some situations in which the robot does actually need to be briefly dishonest. But my experience was that people felt like we were trying to lead to the end of the world.
We are always interested in the pro-social aspects of these human-robot techniques. We’re trying to help people, not be something bad.