A.I. can help us have better conversations about death and dying
Scientists are studying the speech patterns of conversations near the end. Here's what they're finding.
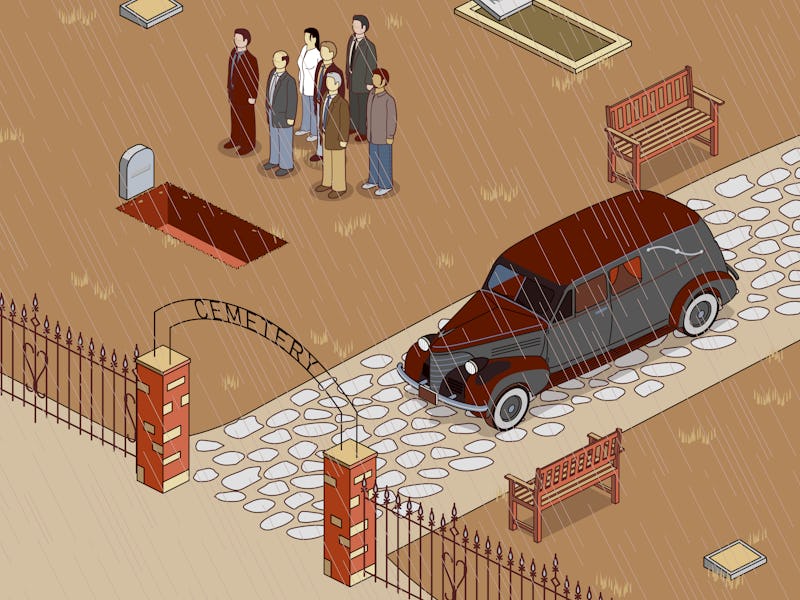
End of life conversations can be some of the most difficult, and important, conversations in our lives. These conversations take place in hospitals and hospice every day but do not always effectively prepare patients for what’s to come. To help bridge this communication gap, new research from the University of Vermont is using machine learning to better understand how these conversations unfold.
The study was published Monday in the journal Patient Education and Counselling and used a natural language processing machine-learning algorithm to analyze the audio recordings of 354 palliative care consultation sessions. The session audio recordings were freely consented to and consisted of conversations between 231 patients and 45 clinicians. Within this study cohort, the authors report that all patients had some form of metastatic cancer and were largely aged 64 and older.
The team evaluated these conversations by breaking them all into ten sections each, with even distributions of words in each section, in order to find patterns in the usage and frequency of words pertaining to time, illness terminology, sentiment, and words indicating possibility and desirability changed.
Robert Gramling, director of the lab in UVM’s Larner College of Medicine and a lead author on the study, said in a statement that this kind of meta-analysis is essential to help understand the elusive inner mechanisms of communication.
“We want to understand this complex thing called a conversation,” said Gramling. “Our major goal is to scale up the measurement of conversations so we can re-engineer the healthcare system to communicate better.”
From studying these conversations the researchers write that several distinct patterns arose. First, as conversations progressed, the use of negative and past-oriented words such as ‘bad,’ ‘tumor,’ or ‘bleeding’ shifted noticeably to more positive and future-facing words, such as ‘will,’ ‘you,’ or ‘can’ — all as coded by a sentiment score the researchers derived through crowdsourcing.
The research also found that the conversations progressed from discussing symptoms at the beginning toward more positively discussing treatment options at the end.
Gramling told Inverse that these trends all represent a kind of average narrative arch for these kinds of conversations, but that future research will look to find how many distinct narrative patterns emerge from these conversations. From there, they can then begin to determine what kind of response works best in different scenarios.
And while it might seem initially counterintuitive to use an algorithm to quantify something some emotionally charged, Gramling told Inverse this couldn’t be further from the truth.
“We’ve been using these methods to try and develop scalable measures of conversation,” says Gramling. “Meaning that we recognize scientifically that we don’t really understand [conversation] well. And what I mean by that is there isn’t one set of things that happen in a conversation that’s going to work for everyone… So we really need to be able to mature our ability to study conversations.”
Gramling continued to say that traditional methods of evaluating such conversational characteristics are cumbersome to do by hand and that scaling this understanding is really best done in conjunction with an algorithm.
Sheldon Solomon, a professor of psychology at Skidmore College who studies human awareness of death and was not involved with this study, tells Inverse that he agrees that A.I. is an appropriate approach but emphasizes that algorithms alone won’t be the only way this problem is solved.
“[An] A.I. approach is fine for these kinds of problems to the extent that these are sophisticated techniques that provide information from large piles of data that would be impossible to discern patterns from otherwise (or at least it would take a really long time and great effort to do this otherwise),” says Solomon. “[B]ut I am wary of sole reliance on such data-driven methods in the absence of theory-driven hypotheses which help researchers determine what to look for in the first place.”
Another question raised by the study, says Solomon, is how these kinds of conversation trends would change over a longer period of time.
Questions like these, Gramling told Inverse, are what he hopes future research can help uncover. He says that he hopes these better understandings of language can help eventually lead to desperately needed over-hauls to the healthcare system at large.
Gramling also emphasized to Inverse that these kinds of approaches are made possible by diverse teams coming together to ask these questions and that this diversity of thought is what he believes will drive such research forward.
>Abstract:
Objective: Serious illness conversations are complex clinical narratives that remain poorly understood. Natural Language Processing (NLP) offers new approaches for identifying hidden patterns within the lexicon of stories that may reveal insights about the taxonomy of serious illness conversations.
Methods: We analyzed verbatim transcripts from 354 consultations involving 231 patients and 45 palliative care clinicians from the Palliative Care Communication Research Initiative. We stratified each conversation into deciles of “narrative time” based on word counts. We used standard NLP analyses to examine the frequency and distribution of words and phrases indicating temporal reference, illness terminology, sentiment and modal verbs (indicating possibility/desirability).
Results: Temporal references shifted steadily from talking about the past to talking about the future over deciles of narrative time. Conversations progressed incrementally from “sadder” to “happier” lexicon; reduction in illness terminology accounted substantially for this pattern. We observed the following sequence in peak frequency over narrative time: symptom terms, treatment terms, prognosis terms and modal verbs indicating possibility.
Conclusions: NLP methods can identify narrative arcs in serious illness conversations.
Practice implications: Fully automating NLP methods will allow for efficient, large scale and real time measurement of serious illness conversations for research, education and system re-design.