A Brain-Computer Interface Can Translate Simple Thoughts Into Speech
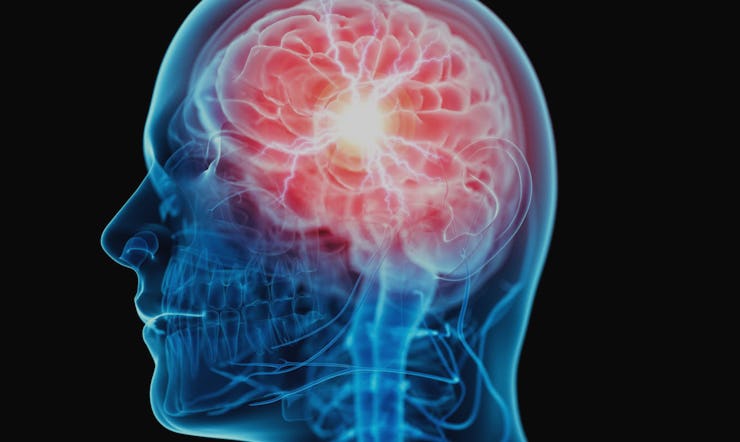
Neuroengineers have created a new system that can translate simple thoughts into recognizable speech, using artificial intelligence and a speech synthesizer, according to a study published Tuesday.
A team of New York-based researchers was able to reconstruct words using only brain activity, an innovation that could pave the way for brain-controlled technologies like, say, a smartphone that can translate your thoughts into text messages.
Dr. Nima Mesgarani, an associate professor at Columbia University, led the study and tells Inverse that he sees great potential to help restore speech to people recovering from a stroke or living with amyotrophic lateral sclerosis (ALS). Further down the line, this type of tech could also open up doors to brain-connected smartphones that could let users text using their minds, though that’s still a ways away. His work was published in the journal Scientific Reports.
“One of the motivations of this work…is for alternative human-computer interaction methods, such as a possible interface between a user and a smartphone,” he says. “However, that is still far from reality, and at the moment, the information that can be extracted using non-invasive methods is not good enough for a speech brain-computer interface application.”
A representation of former Grateful Dead drummer Mickey Hart’s brain created by researchers at the University of California in a separate study.
Listen to the speech generated by the brain-computer interface.
To develop the new technique, Mesgarani and his colleague, Dr. Ashesh Dinesh Mehta from the Northwell Health Physician Partners Neuroscience Institute, began by examining the brain activity of epilepsy patients for their study. These patients already had electrode implants in their brains to monitor seizures, which Mesgarani and Mehta were able to use to gather data for their research.
The duo asked willing participants to listen to speakers recite the numbers between zero and nine, and then recorded the brain signals from that interaction. Next, they trained a neural network — a program that imitates neuron structure in the human brain — to recognize patterns in the signals and translate them into robotic-sounding words using a speech synthesizer, known as a vocoder.
The result was a short voice clip of what sounds like Microsoft Sam counting from zero to nine. The impressive part is just how clear the speech is compared to other methods the researchers tested. There’s still a lot of work to be done, though.
The type of surgically placed implant that the patients of this study had.
"It may take a decade before this technology becomes available."
“It may take a decade before this technology becomes available,” says Mesgarani. “We need more progress both in long-term, bio-compatible implantable electrodes and/or breakthrough technologies in non-invasive neural recording methods. We also need a better understanding of how the brain represents speech, so that we can refine our decoding methods.”
The patients who were a part of this study, for example, all had brain surgery to implant electrocorticography monitors. This is an extremely invasive process that requires open brain surgery, something that most people might not be willing to undergo, even if there was a possibility of restoring some of their speech capabilities.
For now, this study introduced a method for decoding brain signals into speech. If we figure out how to accurately detect brain activity without surgery, we’ll be one step closer to not only revolutionizing speech therapy, but potentially toward bringing about brain-connected smartphones.
Brain-computer interface research has been receiving newfound interest in the past few years. In April 2017, Facebook announced it was working on a BCI during its annual F8 conference. And Elon Musk announced in November 2018 that Neuralink, his own BCI startup, was hiring.
Abstract
Auditory stimulus reconstruction is a technique that finds the best approximation of the acoustic stimulus from the population of evoked neural activity. Reconstructing speech from the human auditory cortex creates the possibility of a speech neuroprosthetic to establish a direct communication with the brain and has been shown to be possible in both overt and covert conditions. However, the low quality of the reconstructed speech has severely limited the utility of this method for brain-computer interface (BCI) applications. To advance the state-of-the-art in speech neuroprosthesis, we combined the recent advances in deep learning with the latest innovations in speech synthesis technologies to reconstruct closed-set intelligible speech from the human auditory cortex. We investigated the dependence of reconstruction accuracy on linear and nonlinear (deep neural network) regression methods and the acoustic representation that is used as the target of reconstruction, including auditory spectrogram and speech synthesis parameters. In addition, we compared the reconstruction accuracy from low and high neural frequency ranges. Our results show that a deep neural network model that directly estimates the parameters of a speech synthesizer from all neural frequencies achieves the highest subjective and objective scores on a digit recognition task, improving the intelligibility by 65% over the baseline method which used linear regression to reconstruct the auditory spectrogram. These results demonstrate the efficacy of deep learning and speech synthesis algorithms for designing the next generation of speech BCI systems, which not only can restore communications for paralyzed patients but also have the potential to transform human-computer interaction technologies.
Related Video: Brain Wave Sensing Robots Can Serve as Extensions of the Human Body