A.I. Can Predict Whether You Have Sepsis Before Doctors Even Know It
"We need fewer buzz words; we need systems that work."
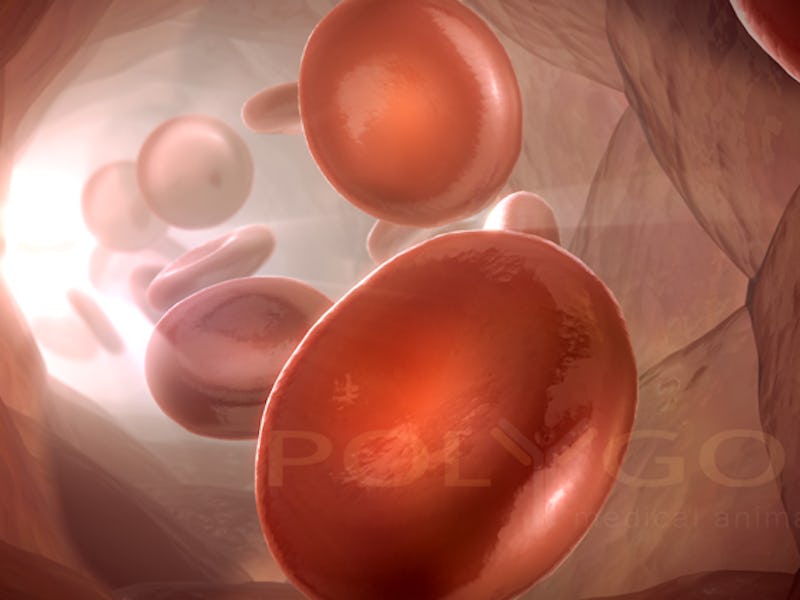
Severe sepsis is an infection complication that strikes more than a million Americans a year, and usually, by the time doctors identify it, it’s too late. New A.I. programs are helping doctors identify it early, even when there are no observed symptoms.
With machine learning — a type of A.I. that gives computers the ability to learn — we can predict how diseases and treatments will impact patients, says Suchi Saria, assistant professor of computer science, health policy, and statistics at Johns Hopkins University.
Known for her algorithms that can detect health risks in premature newborns and septic shock (severe sepsis plus very low blood pressure and organ failure), Saria presented her findings at the 11th Annual Machine Learning Symposium recently at the New York Academy of Sciences. By collecting data about group (like age, race, gender) and individual health, doctors can use machine learning algorithms to tailor treatments.
It kills more people each year than breast cancer, prostate cancer, and AIDS combined.
“A tool like this can identify people who are likely to have a kind of disease,” Saria tells Inverse. “You can identify these individuals very early by using data that’s stored.”
When patients visit the doctor, they often have to undergo routine tests. With Saria’s system, doctors can input the data into an electronic health record, and A.I. can predict if a person’s health condition will decline, improve, or stay stable. This can often be difficult for doctors to predict, especially since diseases can take unexpected pathways.
It can also predict how different types of treatments can affect patients. For example, doctors can use the system to predict how three different doses of medicine for managing blood pressure to find the best next step.
Saria’s system just went live at Johns Hopkins, and she’s hoping it will eventually be adopted on a large scale. “That’s where I’m hoping the field will go,” she said.
What are the everyday implications of your research?
It depends on each disease area. For example in scleroderma, because there’s so much diversity in the symptom profile, and different people have different sets of complications, the disease affects different people differently. We’re trying to give the clinician a picture of what a specific individual’s future trajectory is going to be, and this allows physicians to tailor treatments.
How will the machine learning system you worked on be used in hospitals?
Sepsis is the 11th leading cause of death. The challenge with sepsis is that it doesn’t get recognized early enough. We’ve deployed a live integrated system that can take clinical tests that are routinely measured when patients are admitted to a hospital and can infer who is at risk for sepsis. Our approach also makes recommendations for treatments and allows physicians to take action.
I often get emails from health care providers where they read our papers in order to implement these algorithms. Seven out of ten get stuck because they are unfamiliar with the techniques involved. The data are really messy. Also, for them, this is a foray into state-of-the-art engineering and machine learning. This made us consider implementing a secure cloud-based version so that others users can use it readily.
Our system just went live at Hopkins. We’re doing a pilot trial that allows us to measure physician behavior and how it’s affecting practice. We’re hoping in the next few months to collaborate with a few external institutions to deploy this.
What’s the benefit of using machine learning systems for treatment?
For many decisions about our health, it is unclear what is the right course of action: should we take some aggressive treatment course with strong side-effects or should we settle for a less invasive therapy. These are the types of scenarios where machine learning can help. For example, if you’re an elderly person who is fragile and in the advanced stages of a disease, you might choose palliative care so as to sustain yourself and enjoy your family if you learn using your own data that the treatments are not very likely to be effective.
How can engineers train A.I. in making the best decisions for a diverse range of patients?
Because the system has so much memory, the system can learn and restore as many models as possible. The system can afford to learn all sorts of nuances, which is different from how humans think. We want succinct pieces of information, and the system can be precise. It’s almost easier to imagine how computers integrate many sorts of data, so we can much more precisely tackle disease.
Did any doctors have concerns about machine learning making health care decisions for us?
Yes, they have tons of concern about using these types of systems. If the machine gives you any specific answer, how do you know it’s correct? And, we need a way for it to tell us when it is unsure. We need these recommendation systems to produce answers that can be relied upon. For these, we need to benchmark these systems more rigorously. And, provide a more natural way for the care team to understand reliability.
Where are the gaps and what will it take to do this faster?
Many existing companies make tall claims about what they can do. In part, this is because of the pressure from marketing to sound like they are beating the company next door. In the process, many of the claims are false. We need a way to rigorously benchmark or validate data-driven systems. We need fewer buzz words; we need systems that work and have clean data supporting when and how well.
Also, we need better policy for opening up electronic health records (EHRs). EHRs are databases where clinics and hospitals store your data every time you visit the doctor. At the moment, EHRs control who can get access to your data. So, for example, if you wanted your hospital to use a service for sepsis monitoring, it is only possible if the EHR vendor builds it themselves or agrees to allow this third party access to your data. There are no clear incentives for EHR vendors give third parties access to the data.
Going forward, what do you plan on doing with your research?
On the practical side, the one I’m most excited about is the system we’re building for sepsis. We have developed a system that is real-time, highly interactive, using state-of-the-art principles. We think this can scale to thousands of hospitals. It’s exciting to think that this system could change status quo and in a few years, we may be able to help change practice so that sepsis is no longer one of the leading causes. I want to see it move down that list. It’s exciting that we don’t have to wait for several more years to make that happen.