Smarter Robots Are More Likely to Stab Each Other in the Back
But they'll cooperate, too, in the right situation.
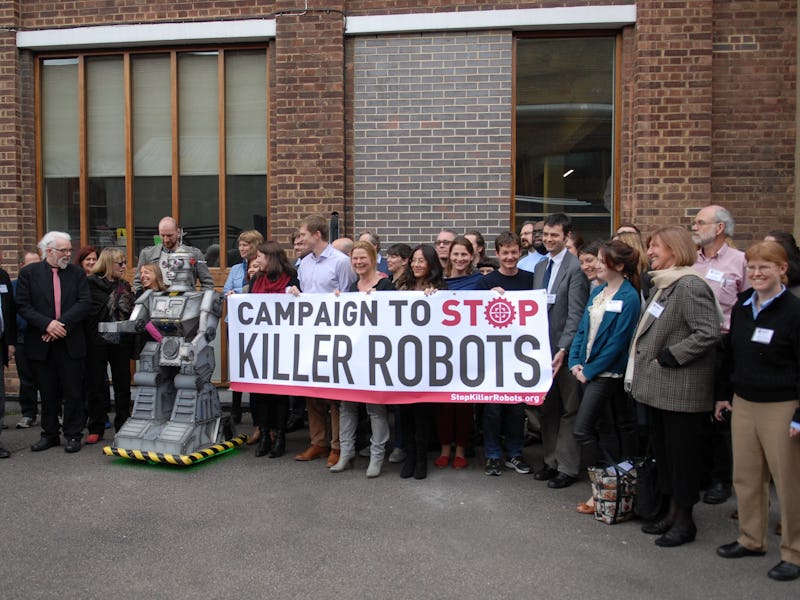
Is it better to cooperate with your peers and achieve goals together or eliminate your competition and achieve goals alone? In a research paper published this week, new DeepMind simulations suggest that whether robots are more likely to kill or cooperate could depend on how intelligent they are. Okay, so they don’t kill, per se. But in two different games, researchers found that neural nets that are capable of considering a greater number of factors when making complex decisions show a differing likelihood of betraying their peers versus cooperating with them. In one situation, the more complex agents defected, while in the other, the more complex agents cooperated.
DeepMind researchers have already proven themselves adept at game theory, with their AlphaGo A.I. kicking ass at a complex strategy game. This new research explores different territory. The paper, published online Thursday, explains that the choice to cooperate or defect in a game is much more complicated than simply considering a single action. To make these choices requires an agent to broaden its perspective, considering the context of a situation.
To demonstrate this concept, DeepMind researchers had neural networks play two different games: a fruit-gathering game and a wolf pack game.
In the fruit game, each agent gathers apples. The agents have the option of pointing a beam at their opponent, temporarily disabling the opponent. When the researchers lowered the number of apples available, they found that the agents responded to the scarcity by freezing each other more often, allowing them to collect apples at their own pace. In times of abundance, they left each other alone.
But when the researchers employed agents powered by more complex neural nets, nets that more closely mimic certain elements of the human brain, the agents were much more gung-ho about freezing each other, regardless of the number of apples available. In short, the smarter they were, the more likely they were to disable their peers, even when they didn’t need to.
An interesting twist on this concept emerged in the wolf pack game. In this game, the agents had to chase prey. An agent received a reward if it caught the prey. But if both agents were in the vicinity of the prey when one caught it, they both received an even greater reward. This game rewarded cooperation.
While the more basic agents tended to act independently, the agents powered by more complex neural nets tended to cooperate, maximizing rewards for all. So in this case, the researchers concluded that default behavior was defecting, and the more complex behavior was cooperating.
“Cooperation and defection demand differing levels of coordination for the two games,” write the paper’s authors. “Wolfpacks cooperative policy requires greater coordination than its defecting policy. Gathering’s defection policy requires greater coordination (to successfully aim at the rival player).”
So in the end, there’s no solid way to tell whether a robot is more likely to kill you based on its intelligence alone. And the truth is, this study doesn’t yet have any real-world application. But it does add an important piece to our understanding of how artificial intelligence agents work. In a blog post, the researchers expressed their desire for a greater understanding of cooperation among A.I. agents to help us make more efficient use of complex systems such as traffic and economies.
Here is the paper’s abstract:
Matrix games like Prisoner’s Dilemma have guided research on social dilemmas for decades. However, they necessarily treat the choice to cooperate or defect as an atomic action. In real-world social dilemmas these choices are temporally extended. Cooperativeness is a property that applies to policies, not elementary actions. We introduce sequential social dilemmas that share the mixed incentive structure of matrix game social dilemmas but also require agents to learn policies that implement their strategic intentions. We analyze the dynamics of policies learned by multiple self-interested independent learning agents, each using its own deep Qnetwork, on two Markov games we introduce here: 1. a fruit Gathering game and 2. a Wolfpack hunting game. We characterize how learned behavior in each domain changes as a function of environmental factors including resource abundance. Our experiments show how conflict can emerge from competition over shared resources and shed light on how the sequential nature of real world social dilemmas affects cooperation.