Emotional Search Engine Says Media Loves Trump, but Engineers Are Debugging It
Lava is looking to put a different algorithmic spin on your search results.
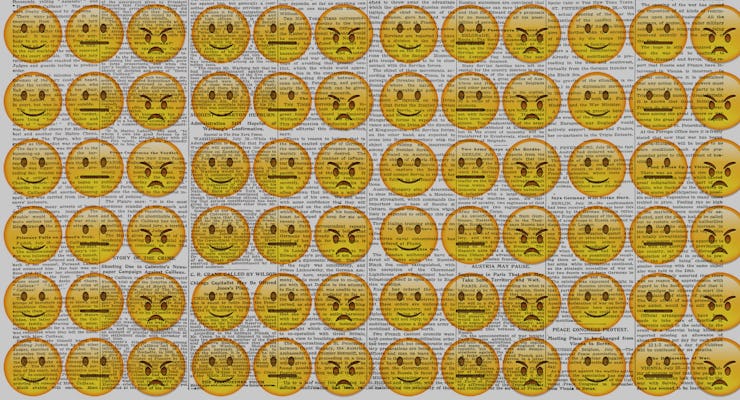
Next time you’re wondering whether or not the press is doing your preferred candidate justice, you don’t have to rely on talking heads to confirm your neurotic hypothesis. There’s an algorithm for that. Next time you want to make sure that the media is fawning over Bieber as it should be, you don’t have to pick through Billboard and Rolling Stone reviews. There’s an algorithm for that. Next time a major corporation does something terrible, you can kick your feet up and watch public sentiment plummet. There’s an … yeah, you get it.
A Google search can only go so far. Way back in 1998, Google decluttered the vast, cobwebbed attic that was the internet. Now, your searches surface articles with specific qualities indicating their timeliness or relevance (SEO headlines, links that work, strong secondary terms). What Google does not offer is an interpretation of its own results. If you’re looking to understand more than just the fact that Facebook’s Trending News section is itself trending, you’re out of luck. That’s where Lava, the search engine defined to analyze semantics, comes in. It doesn’t just answer your query directly, it answers it emotionally.
Thus far, Lava’s indexed eight million news articles. It’s effectively churned through each major UK newspaper’s online archive. For each new article Lava indexes, it spits out a subjectivity score and, if it is not completely neutral, a sentiment score. Lava will show whether the article in question is positive, negative, or something in the middle.
“The program works through a piece to understand the ‘entities’ mentioned — the people, subjects, and things,” Lava co-creator James Finlayson explained to Inverse over email. Next, the algorithm picks out the article’s adjectives. Each is “assigned a score” that represents the given adjective’s positivity or negativity. “Finally,” writes Finlayson, the algorithm “works out how those adjectives are assigned or ‘directed’ towards the entities and then adds up the score, across adjectives, for each entity.”
This function is not altogether groundbreaking. Twitter, for instance, allows users to search by sentiment. But Finlayson said those analyses aren’t up to snuff. He and his team chose to limit their algorithm to newspapers because articles, unlike social media posts, “don’t use slang, use well constructed sentences, and aim to be clear in their writing.” The writing is easier for the algorithm to process reliably. “It’s a lot easier to get retrieve a valid representation of the author’s feelings about a person from a 400 word article than a 140 character tweet,” Finlayson explained.
There’s still plenty of work to do: Lava is still in beta. Finlayson said that when Trump won the New York primaries, for instance, articles in “papers that are very anti-Trump” still turned up positive. Lava’s mistaken judgment, in this case, was due to the fact that Trump’s victory was quote-unquote impressive and indicated that he was on track to win the nomination. No matter how slanted the articles were about Trump himself, writers could not help but use positive adjectives to describe his resounding victory.
If you mess around with Lava, you may notice that some articles show up on both lists, meaning they’re both very positive and very negative. Finlayson explained that this algorithmic schizophrenia is likely highlighting the fact that the article is well-balanced. In a news article, a “glowing quote from someone” about Trump, for instance, may immediately precede a paragraph that details Trump’s innumerable flaws.
"Donald Trump" in green; "Hillary Clinton" in yellow; "Bernie Sanders" in black.
Finlayson told Inverse that Lava enabled him to see more than just an article’s sentiment. He said he was “fascinated to see how scathing, even vitriolic, some publications can be about certain subjects. When you see subjectively negative subjects (particular politicians, religious groups, etc.) turn up more negative sentiment than objectively negative terms (murder, rape, etc.) then you really start seeing the agenda of different publications come to the fore.”
Lava seems to be an exciting first pass at what could become a reliable search mechanism and a means of extracting the finger-pointing from our never-ending national dialogue on media bias. For now, it’s analyzing sentiment fairly well, and it’s a neat tool with which to play around. Lava will soon tackle international newspapers, and that’s when things could get far more interesting: You’ll be able to compare different societies’ biases for or against public figures, athletes, brands, as well as potential despots.