Make Better Decisions With Bayesian Probability, the Smart Way to Consider Risk
A case for modern consumers to embrace Bayesian probability.
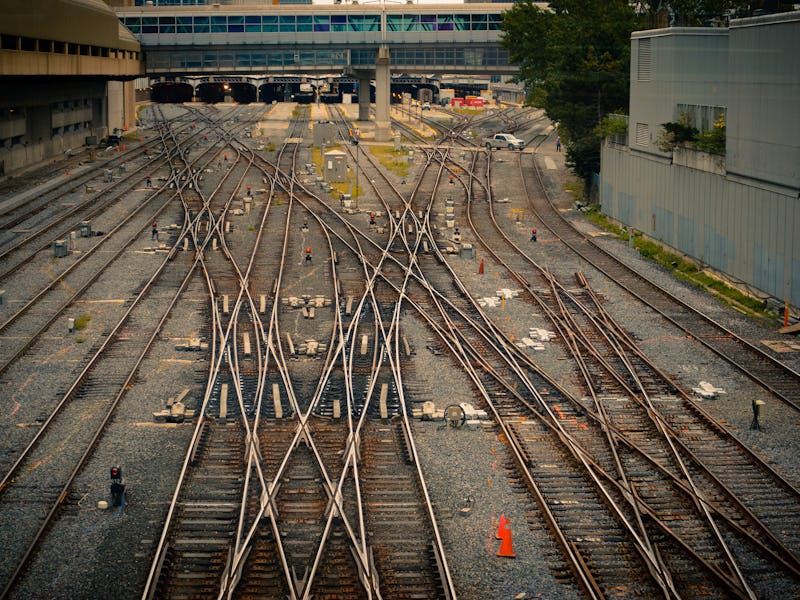
It’s estimated that human adults make about 35,000 decisions a day — the percentage of good decisions depends on the adult. These choices can be as banal as deciding to roll or crumple toilet paper or as emotionally complicated as resolving to leave a relationship. And because humans are subject to rather than masters of their emotional biases, strategies and intellectual frameworks are necessary for anyone hoping to function in a reasonable way. Unfortunately, we’re not always given the best tools. The way most people think about probability, for instance, is ill-suited to the modern.
On any given day, any given person living in a modern society will engage with organizations, machines, and pricing models that they don’t fully understand. Most people approach these daily puzzles in a practical way, by using the information they have to maximize the opportunity for a successful outcome. This is essentially what our parents teach us to do as children. This is often what people mean when they talk about “logic.” But this is also a frequently inadequate process. When there are significant knowledge gaps it differs only slightly from guessing. We are, in short, thinking about probability in an inefficient way. Rather than focusing on outcomes, we should focus on our understanding of situations using the core ideas of Bayesian probability.
Bayesian probability incorporates degrees of belief over historical frequencies: The idea is that decisions made out of uncertainty are informed by what someone originally knows and is updated as one encounters new information. The idea is to minimize risk while maximizing learning. Instead of approaching problems as monolithic, Bayesians cut them up into more digestible pieces. Knowledge is accumulated along the way.
To understand how this works, you’ve got to do the math. The central equation, also known as Bayes’ rule, was formulated by Thomas Bayes, an English clergyman and mathematician who died in 1761. It predicts the sequence of events leading up to an outcome. In the equation, T stands for the hypothesis being testing and E represents the new pieces of evidence that will either confirm or disprove the hypothesis. The beliefs here aren’t objective, but conditional to prior assumptions and what is learned along the way.
The equation allows decision makers to assign likelihoods to pieces of information and events at the same time, layering the likelihood of an underlying assumption proving out on top of the likelihood of a result.
In a 2011 paper Queen Mary University professor Norman Fenton argued that the most effective way to make decisions is through probabilistic models built off of Bayesian networks. He writes that the 2008 financial crisis was a wake up call that people and financial systems need to get better at risk assessment. While Bayesian probability has existed as a critical construct since the 16th century, it isn’t broadly applied or taught. And while it’s obvious that Bayesian thought applies to finances, it also makes sense of a myriad other situations.
“To deal with these kinds of problems consistently and effectively we need a rigorous method of quantifying uncertainty that enables us to combine data with expert judgement,” Fenton writes. “Bayesian probability is such an approach.”
Fenton makes the case for the increased application of Bayesian theory, but it has been adopted before — and to good effect. Alan Turing used Bayesian statistics when cracking codes during World War II. The only reason that it didn’t popularize a new way of thinking was that no one found out until the information was declassified in 2012. That was also the year Nate Silver used Bayes’ equation to predict the 2012 election results with impressive accuracy.
Bayesian probability is better than other future-predicting systems because it also is one of the few methods that accounts for how unpredictable humans really are. While it incorporates what one knows, it also responds to the fact human choice is constantly affected by contextual and situational variables. This is helpful whether you’re trying to figure out what stocks to invest in, or what fruit plate will be the most successful at your potluck.
But how can you apply it today? Simple: Think about what you think you know and why you think you know it before making a decision. Then think about whether that decision will allow you to confirm or deny your suspicions. It’s pretty much that easy. It’s a matter of having the discipline to focus on the why of what happens rather than the simple reality of events. Just because something happens doesn’t make it probable.