Can Google’s "Superhuman" Neural Network Really Tell the Location of Any Image?
It's a step forward, but not necessarily a giant leap.
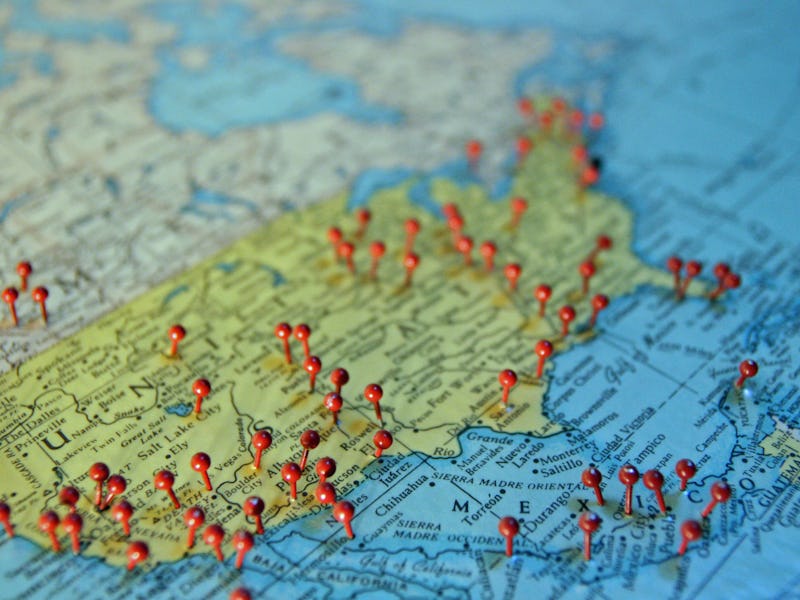
Searching for images is easier than ever. But if you’re trying to find a picture of something at a location that isn’t totally obvious (so not the Egyptian pyramids or the giant thumb sculpture in Paris), it’s harder than you think — even with the geolocation information based on what’s in the image.
Enter Google engineer named Tobias Weyand and a pair of his colleagues. According to a new paper in the journal arXiv (pronounced “archive”), the trio has built a deep-learning machine capable of pinpointing the location of almost any photo based solely on analysis of its pixels.
To get a machine to successfully accomplish a task like this, you want to give it the ability to intuit information based on visual clues. You want it to think, in other words, like a human being.
Weyand set about developing an artificial neural network — a machine system designed to mimic the neurological pathways of the brain, which allow it to learn, process, and recall information like a human could. This new system, PlaNet, is apparently capable of outperforming humans at determining locations of images no matter what the setting — be it indoor or outdoor, and featuring any kind of unique or nondescript visual cues.
Given a query photo (left), PlaNet outputs a probability distribution over the surface of the earth (right).
How does PlaNet worK? Weyand and his team divided up a map of the world into a grid that laid over 26,000 square-like shapes on different regions, depending on how many images were taken in those places. Dense places where a lot of pictures are taken fit in a smaller square, while bigger, more remote regions can cut into bigger squares.
The team then created a large database of images already geolocated — nearly 126 million different photos. About 91 million were used as a dataset to teach PlaNet how to figure out which image could be placed in which grid on the world map.
Then, the neural network was tasked with geolocating the other 34 million images from the database. Finally, PlaNet was set upon a data set of 2.3 million geotagged images from Flickr.
The results? PlaNet could determine the country of origin for 28.4 percent of the photos and the continent for 48 percent. Furthermore, the system could pinpoint a street-level location for 3.6 percent of the Flickr images, and city-level location for 10.1 percent.
While the Eiffel Tower (a) is confidently assigned to Paris, the model believes that the fjord photo (b) could have been taken in either New Zealand or Norway. For the beach photo (c), PlaNet assigns the highest probability to southern California (correct), but some probability mass is also assigned to places with similar beaches, like Mexico and the Mediterranean. (For visualization purposes we use a model with a much lower spatial resolution than our full model.)
And PlaNet is better at this than most human beings — even the biggest globetrotters. Weyand enlisted 10 well-traveled individuals to compete against PlaNet in a game of labeling locations of pictures found on Google Street View.
“In total, PlaNet won 28 of the 50 rounds with a median localization error of 1131.7 km, while the median human localization error was 2320.75 km,” the researchers wrote. “[This] small-scale experiment shows that PlaNet reaches superhuman performance at the task of geolocating Street View scenes.”
Is this for real? Did a Google engineer really just develop a “superhuman” A.I. system?
When it comes to geolocating images, perhaps. And that’s not all too surprising — the point of A.I. isn’t to fundamentally mimic the human brain in all ways, but to surpass human limitations in a few specific ways to accomplish much more difficult tasks. So in that sense, what the researchers write is true.
Still, it’s a stretch to call PlaNet a “neural network.” An ideal form of that kind of technology would be capable of learning about much more than image geolocation. A.I. systems are capable of writing similes and playing Super Mario, but this is small stuff compared to an ideal “master” system that can automatically monitor and maintain vitals, manage transportation or energy infrastructure, and much more.