How Machine Learning and Jazz Piano Luminary Art Tatum Power Online Radio
Art Tatum played so fast they named the musical atom after him.
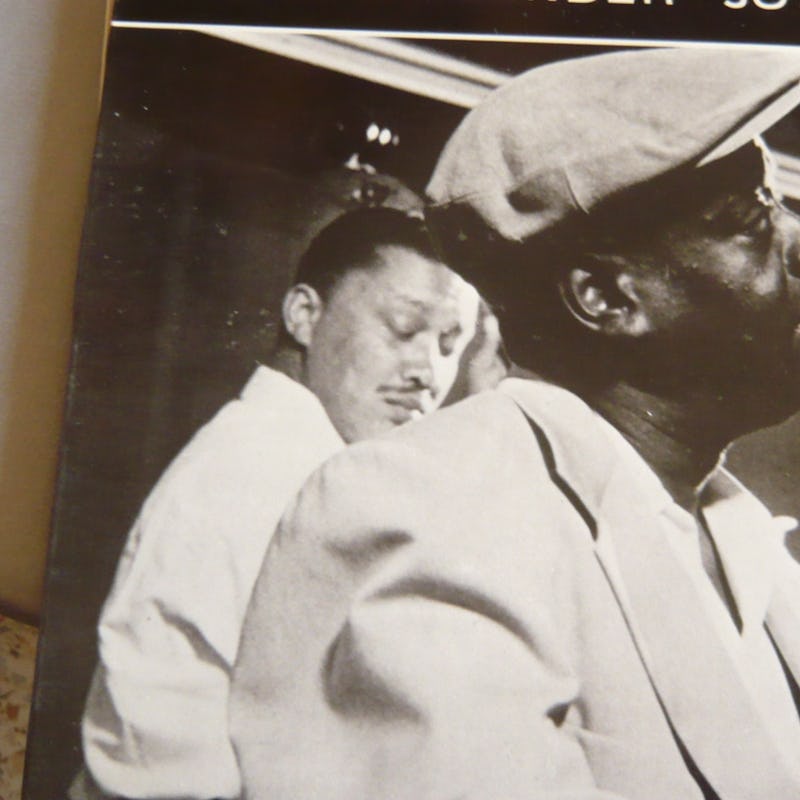
When Jeffrey Bilmes wrote his master’s thesis at MIT in 1993, he couldn’t have known his work on music perception would help launch the world of online radio. Today, an innovation of his is contributing to smart playlists and automated music personalization at the two biggest players in the business: Pandora and Spotify. To understand what makes these services so innovative and ubiquitous today, it’s worth looking back at Bilmes’ insights, and at the musician whose acumen inspired the young scholar to explore the purpose of rhythm: Art Tatum, the jazz luminary who famously played piano like a one-man duet.
In his thesis, “Timing is of the Essence,” Bilmes presented a new concept he named the “tatum.” Sometimes described as a “musical atom,” the tatum represents the smallest unit of time that best corresponds to the rhythmic structure of a song. The pioneers of “music information retrieval” — the science of categorizing and analyzing music computationally — expanded on his work to bring customized music to the masses. The tatum is key to this endeavor. It’s where the human perception of music overlaps with numbers that an algorithm can understand, an attempt to teach machines to grasp music as we do.
The Tatum
As a graduate student at MIT’s Media Laboratory, Bilmes was trying to model musical rhythm – and to teach computers to reproduce it expressively – by breaking it down into its component parts. This is not an easy task. It’s simple to write a program that plays rhythmically flawless music, but the outcome is lifeless, sterile; it doesn’t feel human. This is the standard problem of artificial intelligence: How can we build a program that can duplicate a complex human task? How can we write an algorithm to mimic human instinct?
“When you’re learning to play music and you’re learning to play jazz, there’s a utility to intuitively understanding what it is about music that makes it human,” Bilmes tells me. “Humans are intuitive beings, and humans often aren’t able to describe how they’re able to do human things … I felt at the time that maybe I was violating a sacred oath in defining these things.”
Bilmes needed a model of rhythm that would allow him to compare a recorded human performance to a regular metrical grid. That way he could identify the human deviations from that grid. Most people are familiar with the fundamentals of rhythm. Songs can be split into bars (or measures), and bars can be split into beats. This structure didn’t go far enough for Bilmes’ purposes. For one thing, the succession of beats we all perceive when listening to music is in some sense illusory — where do beats even come from? As Bilmes wrote in his thesis:
“Beats are rarely solitary, however; they are arranged in groups, and the groups are arranged in groups, and so on, forming what is called the metric hierarchy. Beat grouping … is a perceptual illusion which has no apparent practical purpose. For the illusion to occur, the beats can not be too far apart (no more than two seconds), nor too close together (no less than 120 milliseconds).”
For a computer to analyze a piece of music, the researcher must represent the music digitally and impose a regular sequence of reference points throughout the piece. Beats are a natural point of reference to the human ear — but less so to an algorithm.
“The granularity is not high enough to represent the rhythmic subtleties that exist in music,” Bilmes says, speaking particularly of those in African-derived musical styles such jazz or Afro-Cuban rumba. That is, the beats aren’t close enough together to explain to the computer how much music is happening between them.
Musicians rarely play only one note per beat — usually they play two or more — so beat-level analysis would not capture anything between beats. But this is where most of the magic happens. During his previous life as a jazz bassist, Bilmes noticed another rhythmic structure below that of beats.
“When you’re playing music, you have some kind of underlying pulse,” Bilmes says. “When you’re playing beat-based music … there’s a kind of beat in your head, a clock, a high-frequency clock, and you deviate around that clock. You dance around that clock to make it sound human.”
It was this “high-frequency clock” that Bilmes called the “tatum,” to honor a musician famous for playing unbelievably fast. Born in Toledo, Ohio, in 1909, Art Tatum was mostly blind from age four. Although he took classes at the Toledo School of Music — and learned to read Braille sheet music — Tatum was “primarily self-taught, cribbing from piano rolls, phonograph recordings, and radio broadcasts,” according to NPR’s “Jazz Profiles.”
Tatum’s skill drew attention throughout his life. He traveled to New York in the 1930s to accompany Adelaide Hall. His style wowed musicians as diverse as Sergei Rachmaninoff and Charlie Parker. Legend has it that another jazz piano star, Fats Waller, once said of Tatum: “I just play the piano. But tonight, God is in the house.”
For the New York Times in 1990, Peter Watrous sized up Tatum’s talent as a fusion of classical training — a culture that would not have embraced Tatum in the era of Jim Crow — and the outer reaches of jazz. “He reveled in both cultures, and brought the two techniques and sensibilities together as effortlessly as any jazz musician ever has,” Watrous wrote. “Never afraid of his training, he showered his pieces with keyboard-long runs, arpeggios, and dense, progressive harmonies; breathless at times, his pieces cram in idea after idea, luxuriating in musical literacy and choice, in the abundance of pleasure given by information. It is the sound of the modern, industrial world.”
The sound divides audiences generations later. It’s cascading beauty at the brink of madness, pyrotechnic in its showiness, a gaudy pleasure. It’s no wonder Bilmes chose Tatum as the standard-bearer for the base units of musical perception.
“I think Art Tatum is a genius,” Bilmes says. “Ever since I was a teenager, I was just floored by his piano performance … I think his tatum rate was beyond anybody else, or certainly unmatched. When you listen to his performances, obviously to me it sounds like there was some sort of fundamental timing grid that was much faster than the beat of the music. It was incredibly fast. It was so fast that if it had gotten any faster it would have turned into a tone itself.”
From tatums to smart playlists
Later MIT scholars Tristan Jehan and Brian Whitman, who founded the Echo Nest in 2005, used the tatum to build the system that powers iHeartRadio, Spotify and a number of other streaming services. The folks at Pandora’s Music Genome Project go about things in a slightly different way, but they too employ machine-listening techniques that utilize the tatum.
Like Bilmes, Jehan and Whitman did research at MIT’s Media Laboratory, where they found a way to use computers to identify such basic musical characteristics as tempo, key, and mode. This evolved into the Echo Nest’s Analyzer, a program available for free use on the Echo Nest’s website. The Analyzer “listens” to a song, then spits out a bunch of data about that song’s musical properties. The tatum is essential to this process.
“If you listen to [Art Tatum] play, there’s a lot of notes, but your perception of beats might not be faster — it’s just that he plays a lot of notes between each beat,” Jehan tells me. “So the tatum concept was, ‘What is the smallest entity of time that we can use to best represent the notes?’”
The Analyzer divides a song into small chunks called “segments,” roughly equivalent to a single audible event – a note, a chord, a drum beat. From here, the software calculates the song’s tatum rate, the subdivision of beats that most accurately reflects the timing of musical events. The tatums then provide a regular grid that allows the Echo Nest to compare songs, even those of different tempos and lengths.
“You can compare two things that don’t align … they’re at different rates but you can still compare them,” Jehan says. “You can say, ‘If this song was slightly faster or slightly slower, this is how they would compare.’ You don’t have to use an absolute time representation. You can warp things as you wish. You still compare sequences of events, tatum events as opposed to time.”
Something similar happens at Pandora’s Music Genome Project, even as Pandora relies on a team of 30 human musicians to sort and classify music. The company also uses computers, and there the same principle applies: The tatum is used to build a representation of the song that a computer can analyze.
“As far as our human music analysis goes, we don’t really think about the notion of a tatum,” says Steve Hogan, manager of music analysis at Pandora. “It’s more useful in the machine listening.” Still, the Music Genome Project’s human listeners do identify the fundamental rhythmic subdivision of a piece – that is, how many notes occur between beats. Pandora uses the human analysis to test its machine listening techniques; the company pits its computers against its human listeners to see if the machines can reproduce human results.
The point is to identify songs that are similar, to predict a song that users will like, based on songs we already know they like. In the biz, this is called “discovery.” It’s fairly remarkable when you think about it. How do I recommend music to my friends? Do I engage in an exhaustive analysis of a vast database of songs? Maybe I do — subconsciously. But it seems like more of a touch-and-go kind of thing. The fact that Pandora and Spotify have any success at all is impressive. But discovery isn’t the end-all, be-all.
“My personal goal is to understand everything there is to understand about the acoustics of music,” Jehan says. “How music is structured, how it sounds, and how do I really perceive music myself? Why do I like what I like? … It’s basically A.I. for music. Being able to create artificial music intelligence and being able to synthesize new music.”
That’s the Holy Grail of music science. And one day it might be possible, thanks to Jeffrey Bilmes, who taught machines to listen, and Art Tatum, whose irreducible brilliance in the 1930s inspired the machine learning of tomorrow.