The A.I. of Tomorrow May Think at the Speed of Light
Brain-inspired optical thinking machines may be the future of computing.
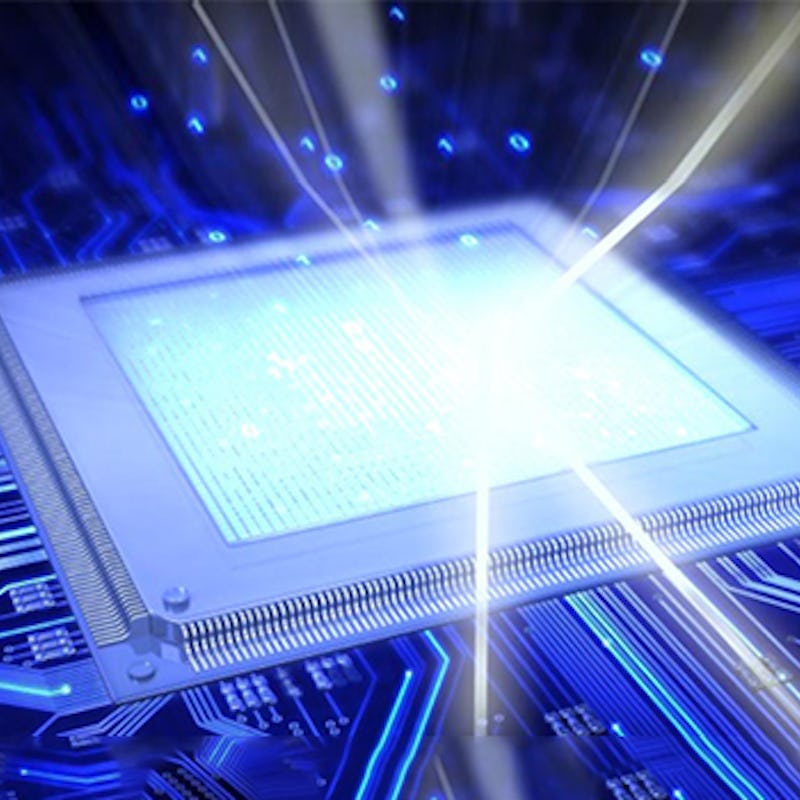
There’s a “neural” revolution going on within computing right now. Yet modern “neuromorphic” devices, which are inspired by the structure of the brain, remember information less like a brain and more like a computer. It’s become clear this distinction could hold allegedly brain-like computers back from achieving truly brain-like things. Scientists have been working on a variety of different approaches to building artificial neurons that actually compute like real ones, and a paper published in May from the Korea Advance Institute for Science and Technology (KAIST) offers the most provocative solution yet: A brain that thinks, and more importantly remembers, in the medium of light.
It’s an innovation the researchers believe could “enable devices to emulate the highly efficient neuromorphic operations of the brain,” and to do so not just as quickly as brains themselves, but significantly faster.
Neuromorphic computers are basically what you get when you physically build a version of the neural network software that enables everything from smartphone voice commands to super-targeted ad campaigns. Racks of servers at companies like Facebook simulate neural networks running complex data-mining algorithms, while neuromorphic computers actually run those algorithms directly. Neural computing can finish the same processes more quickly while using only a tiny fraction of electricity.
In the long term, this should help the A.I. revolution truly take off, with low-powered neuromorphic chips doing full-time data mining in consumer devices, and brain-inspired cloud servers doing unlimited crunching of that data for a pittance in electrical costs. There’s just one problem: Today’s most robust neuromorphic devices, like IBM’s incredible, million-neuron TrueNorth chips aren’t actually made of devices that function like real neurons. The biggest difference is memory: biological neurons have some built right in, and modern artificial neurons do not.
Think of a neuron like a computer. It takes information in, does some operation to that information, and puts the result of the operation out at the other end. But that basic concept can be much more useful if the computer can also remember what has happened to it in the recent past, because then it can start to take action only in response to a buildup of sustained activity, or even deaden its own sensitivity in response to too much stimulation. In biological neurons, this is called the “integrate and fire” model, and it allows brains to run much more complex and useful algorithms than they could without memory. As IBM explains, when neurons can track their past activity for themselves, it takes very few of them to match the pattern-finding abilities of even high-speed computers.
The older artificial neurons in chips like TrueNorth do have access to memory, but that access comes remotely from an external source. It’s a lot like how the RAM in a conventional PC is distinctly separate from the processor, and it has little in common with the human brain, which stores short-term memory in each individual neuron.
It’s a tough technical problem to fix: How do you manufacture millions of little neurons on a single chip, if each of them has to be a complex computer, complete with programmable memory of its very own? The answer seems to be to use some sort of basic material property, something reliable that nonetheless takes no energy to store information.
The most intriguing possibility is to store information not in a neuron’s level of resistance to the passage of electricity, but in its level of resistance to the passage of light. These devices exhibit all the most important learning and memory properties of real neurons, including both long- and short-term memory.
There are two big advantages to storing information this way: one, it doesn’t have to use any expensive materials or exotic phase-change properties, and two, light moves super, super fast.
The fastest neuron in the body can conduct a signal at about 268 miles per hour (120 meters per second), as opposed to a rough 670,398,000 miles per hour (299,695,000 meters per second) for light when it’s moving through air. That speed difference could allow much faster computing by the neurons themselves, and unlike electricity moving through a wire, light doesn’t lose much of its power to resistance as it moves around the computer.
“A photonic-based neuromorphic system can be a more favorable option to enhance computational speed” than prior technologies, the researchers write, “since it would have higher bandwidth, low crosstalk, and lower power-computation requirements.” In that spirit, to describe the function of these neurons the team had come up with the term “ultrafast synaptic computing.”
Remember, though, that the brain has the frontal cortex for storing what we humans think of as “memories,” and so none of this means neuromorphic computers won’t one day start to have portions specialized for storage of higher level ideas. That’s the next logical step: once scientists have created real-artificial neurons robust enough to be the building blocks of real-artificial brains, the only thing left to do will be to start making those brains with separated, interdependent sections, or, cortices.
That’s how true intelligence first got started, in biological brains: when each neuron became sophisticated enough that a group of them could organize themselves in several different arrangements with distinct advantages, the first brains arose as a Frankenstein monster of many of these possible structures all working together.
Truly neuron-like devices will be necessary to allow the same segmentation process to go on in artificial brains, and if those devices think at the speed of light it could be literally impossible for the human brain to keep pace.