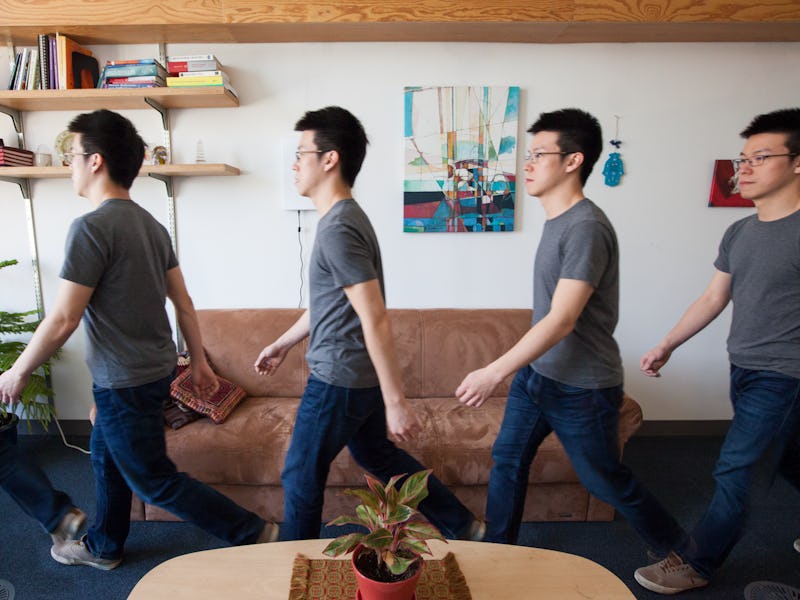
A teen switches abruptly from cleaning with music to calling a friend on the phone. A single parent approaches a closed bedroom door with both arms full of laundry. A lone grandmother falls, and can’t get up without help.
To address all these problems, and far more, a network of household technology would need to have an understanding of not just where a user is in the home, but what that user is doing there. This week, MIT’s CSAIL lab unveiled an incredible new technology that can do precisely that.
Called WiGait, it’s a breakthrough tech that co-creator Dina Katabi told Inverse could make it possible to track a user’s activities “all the time, 24-7, throughout the home.”
WiGait is an improvement on the same team’s prior work on a project called WiTrack, which basically comprises the first half of WiGait. In WiTrack, a person’s location can be found and tracked by using a trio of sensors to detect radio waves bounced off the body, even through walls. This allowed the researchers to tell where in the home the user was — but just what is the computer supposed to do with that information? There’s the obvious ability to turn the lights on and off as needed, but the fact remains that a person’s location, all on its own, does not actually provide a whole lot of insight.
“This device doesn’t see how the person looks,” another of the study’s authors, Chen-Yu Hsu, said in a phone call to Inverse. “It basically sees a person as a blob moving through a space.”
With the announcement of WiGait, the team at CSAIL has taken the next logical step in person-blob analysis: figure out how person-blobs move in different situations, and use that insight to make real predictions about just what a user is most likely doing at any given moment. This could include identifications as simple as, “This person has fallen down,” and extend all the way to, “This person is getting bored with their current activity.”
This gets complicated due to context. “If someone is cleaning, or cooking,” Katabi explains, “and they move from room to room, they might move very slowly. But that’s not because they’re sick, or hurt. It’s because they’re doing something.”
So, if a blob-tracker were told to monitor the movements of a person with Parkinson’s Disease, how would WiGait know the difference between slow movement due to sickness, and slow movement due to any of the other causes of that particular behavior?
The answer is, with many different levels of analysis, some of which involve a machine learning approach. The simplest level of analysis looks at a person’s stride length and speed, but by trying to determine when a person is cleaning, it’s possible to look at their movement speed in context. If a person is moving more slowly than normal, but as expected for their current activity, then their slow movement speed likely means nothing at all.
By figuring out what a person is up to, the system could do things like put on a particular type of music (“Put on music the user likes to have on during work”), or adjust that music’s volume as different events occur in the house (“Turn down music so the user can talk to the pizza delivery guy”), or call for an ambulance (“That’s the floor, not a bed!”).
Hsu said that a single WiGait rig should currently work for about the space of a one-bedroom apartment, but that it is designed to work with multiple, layered rigs all collaborating to cover the entirety of a house. Though the range of the system might be extended over time, consumers may find they prefer the coverage resolution provided by smaller tracking radii; if a single setup could cover an entire house, it wouldn’t be possible to set it up so that it covered everything except, say, the bedroom.
“For coverage,” said Hsu, “it’s basically the same as with wifi routers.”
One obvious possible application for the technology also illustrates why it needs to be approached with caution: house arrest without the need for tracking anklets. With this technology, it could be possible to track people against their will, both with and without their knowledge.
“Privacy is of course always a big topic that we think about very carefully,” says Katabi. “You have to careful to be very careful about who owns the information, and who has access to it.” The “people” tracked by the system are, remember, mere person-blobs.
Still, a couple of years ago MIT’s own CSAIL lab did produce a wifi tracking study that could identify individuals based on their movements, and in any case a criminal or spy would presumably already have some knowledge of the identity of a person whose movements they wanted to track. It would probably be difficult to set up WiGait in a home without the home-owners knowledge, simple because its sensors must be central in the home, but a hacked version doesn’t need to be invisible to compromise individual (and corporate) security.
There’s no denying that WiTrack is an incredibly powerful new technology, one that could coordinate the Smart Home so it can finally fulfill the prophecies of life-changing abilities that we’ve all been hearing for so long. There’s also no denying it could fundamentally change the amount of privacy any person can realistically expect in the future, and greatly expand the powers of cyber-criminals and cyber-attackers of all stripes.
Some see installing a piece of smart technology in the home as a bit like inviting a spy to take up residence — but that’s not true. These days, human spies don’t have anywhere near the level of insight that technology is starting to be able to provide, all on its own.