Can You Teach an A.I. to Be as Brilliant as Messi?
Unsupervised learning is starting to make real, practical achievements.
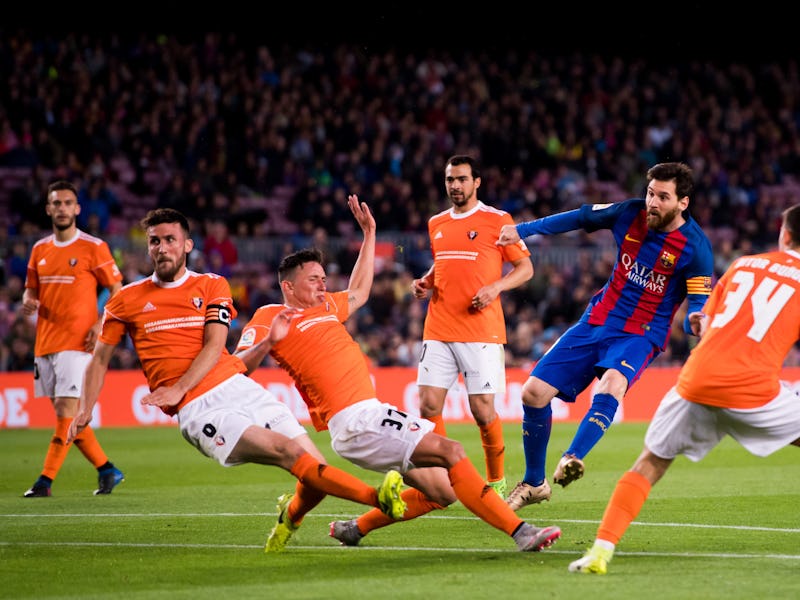
Hoang Le has been a soccer fan all his life — his childhood hero was Zinedine Zidane, and as a recent immigrant from Spain, he thinks FC Barcelona’s Lionel Messi is the best player who’s ever lived. He knows the game inside and out, but in order to teach a computer how to play the beautiful game, Le and his team at CalTech were recently forced to release their authoritative control, and take a step away from the teaching process.
It turned out that the expertise that comes with fandom was irrelevant to a computer. The team’s study, published in March shows that while a machine learning approach can let a computer discern the real principles of soccer, it might nonetheless require the human soccer experts to take a reliable step back from the process.
So-called “unsupervised learning” is starting to make practical breakthroughs, and it’s taking A.I. into not just uncharted, but unchartable territory. It could not only spur new products and innovations, but go on to become the basis for the A.I.s of science fiction. It could challenge our concept of what makes us human.
This particular breakthrough started outside of soccer. In 2013, the NBA’s Toronto Raptors introduced a coaching idea called “ghosting,” which analyzed player data and found holes in a team’s defense. It also found holes in the defense of opponents.
When Le and the team at CalTech heard about “ghosting,” they didn’t know precisely how it worked — but they knew its analysis was based on the expert insight of the Raptors coaching staff. That means that while their basketball software might well be a basketball A.I., it’s still limited in precisely the same ways as the coaches who created its strategic preferences.
The Raptors program can be far more observant than a human being in looking at the plays, but it can’t invent new understandings of those plays. It didn’t have that capability.
In the parlance of machine learning, Le and his team identified the limitations of using supervised machine learning algorithms, or those that have specific, human-defined rules that govern what sorts of short- and long-term goals they’re trying to achieve.
Unsupervised machine learning approaches have been interesting to researchers but, for obvious reasons, too unfocused to be very useful for problem-solving. Now, the sophistication of their self-directed pattern finding can be useful on data without human direction. Difficult and time-consuming though it is to have an A.I. bootstrap its way to new insight, it’s the only way to produce the sorts of findings that A.I. creators couldn’t conceive on their own.
“The original motivation was to see if we could teach an A.I. directly from the data,” Le tells Inverse. Luckily, the CalTech’s ongoing work with Disney meant that they also had a corporate connection to ESPN. The team was able to get access to masses of precisely the right kind of data, particularly on soccer, and immediately set about designing a machine learning system to crunch that data and see what it could see.
The nice thing about supervised learning, though, is that the creators can give it an unfair advantage in the form of labeled data. By contrast, Le and his colleagues had trouble getting their A.I. to differentiate different player types, thus to separate its study of defensive play from offensive play, and to avoid having goalkeeper data muck up every other position.
Le pointed out that to actually identify one particular collection of data as a player type, however, is a remarkably difficult thing to do (goalie aside).
“What Does It Mean to Be a Left-Back?”
“I come to you and I ask, ‘What does it mean to be a left-back?’ That’s actually quite a tough question to answer.”
The naively simple needs of a machine show that even experts in a game have difficulty answering fundamental questions about the design of the system they know so well.
By using their unsupervised approach, the team was able to start categorizing players simply by having an A.I. watch their real, tracked movements. Though it doesn’t have a concept of a left-back, the A.I. can notice that about one-eleventh of the tracker points play very similarly to one another, and that they tend to conform to reliably different rules than data points that seem to be in other, equally reliable categories. These automatic position assignments saw through the variability of different individuals’ approach to their positions, as well as any transient changes in play, such as when a goal is near and everybody is playing defense.
What this means is that they can have their A.I. quickly learn the strategies of a new team simply by giving it a mess of tracking data. “If you’re a coach you can ask if, given this same situation, how would another team have reacted?” Le says. This is true both for large-scale formation strategy, and the minutia of moment to moment play. “You can do game analysis to analyze strategy for your team or opponents.”
If a coach wanted to drive home the importance of a different style of defensive play, they could literally swap in players they’d prefer and re-run scenarios to show their team how much more successful they could have been.
“We think that with good tracking data, this could be extended to other sports, especially continuous sports like basketball and hockey.” Le hopes that some version of their approach will eventually work its way into the hands of Raptors training staff whose original ghosting system helped motivate that approach in the first place.
Of course, unsupervised learning can be applied much more widely than soccer. Just like this soccer A.I. was able to make sense of disorganized data on its own, Google’s incredible Go-playing A.I., AlphaGo, produced a number of moves that have astounded the Go world with their insight.
That’s a level of sophistication that had to come from a basic, unbiased understanding of the game, on the A.I.’s own terms and without the introduction of the same preconceptions that have produced the current, human style of play.
In sifting soccer data for its latent player structure, the A.I. looked at things like whole-team formations and coordination between positions, truly engaging with the strategy of the game. It isn’t a version of AlphaGo tailored to the non-existent game of top-down-2D-soccer, but its abilities show that modern learning algorithms could produce such an A.I. and that it would have the capacity to produce all-new insights into the game.
These are the sorts of learning techniques that could produce robust machine intelligence that could autonomously explore a dark crater on Mars and decide for itself what’s interesting enough to collect for later analysis.
Le is now working toward an unsupervised approach to machine vision in drones, improving their ability to autonomously traverse a complex, highly kinetic world in real time.
His love of soccer hasn’t been diminished by watching a machine begin to autonomously grasp the nuances of the game, but he does think it could eventually come to change the nature of play in ways human coaches might never have managed.
“It’s a very exciting time,” he says. “You think, ‘what will A.I. do next?’”