An A.I. Just Developed Its Own New Language
The technique mimics the incentives that led humans to form language.
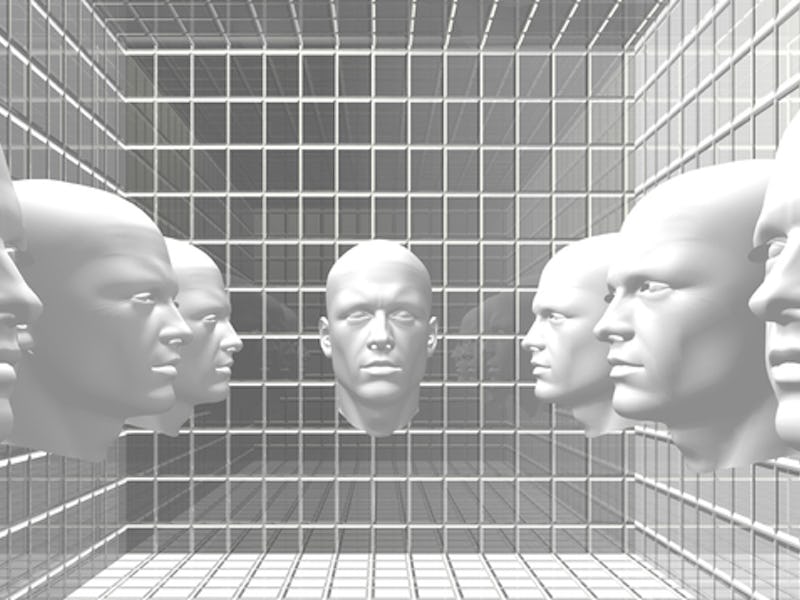
New research from OpenAI and UC Berkeley has created A.I. agents that can form and use their own new language, without instruction, whenever they need to. The languages are systematic and roughly grammatical, and even include aspects of non-verbal communication like body language!
It all makes for an incredible glimpse into how (and why) language may have arisen during biological evolution, and it shows the nuanced insight we can derive from modern learning agents.
Like so many studies that set out to elicit a specific A.I. behavior, this one began by creating a rough metaphor for real life. The experiment sets its A.I. agents in a simulated physical world containing landmarks at fixed positions, and then gives them the ability to roam freely within this two-dimensional space. The agents were then given a goal, usually to send another agent to a specific place in the world, and a set of nonsense symbols each could “say” aloud so the others could “hear” it. They don’t literally speak aloud like a physical robot — but that might not be too far out, either.
Metaphorically, these agents had a mouth, along with grunts to come out of it, and a world, along with legs to move through it, and goals to achieve in it. Those, and complex learning algorithms, were all that were needed to generate a real, novel language from scratch.
In the video below, the English word “red” is substituted for the nonsense symbol the agents used and, over time, assigned the meaning “red.” Each animation is the product of many learning runs in which the agents slowly assigned the required meanings to symbols, and learned how to use them properly.
Of course, the language is very simple. The agents began by giving each other simple, single-word instructions. When there’s only a single landmark in the space, a simple command like “Go” is enough — there’s only one place to go. But introduce more than one possible target, and suddenly we need both verbs and nouns. There’s even needs-based grammar. The phrase “Go Red,” means go to the red target, and is always preferred to “Red Go,” because in the first construction it’s possible for the agent to start moving in the direction of all targets even before it knows which of them is the final goal.
Another fascinating finding is that, if they remove the ability to vocalize but keep the goals in place, other forms of communication arise. Sometimes agents will lead each other to the destination, or just visually point the way. Take away the ability to point and even to see each other, and they will begin to simply push one another around the world! In all cases, the researchers simply provided the ability to speak, or to point, or to bump one another; it was the A.I.s that learned how to use those abilities to achieve their goals. Pushing is fairly simple but, it would seem, not all that much simpler than early verbal communication.
The research also replicated some of the difficulties of real language, including ambiguity. Tell an agent to go to the green landmark, but give it two identical green landmarks in the space, and it will simply sit and tremble at the midpoint between them.
Of course, there were also idiosyncrasies arising from the agents’ nature as software, rather than biological wetware. One is the ability to use complex patterns as language, requiring the team to add a slight extra cost to re-use of a word after it had already been uttered. Without that amendment to their language rules, the agents tended to develop Morse Code-like language based on patterns of spaces and a single, repeated word. That’s technically a language, but it’s much more difficult for the researchers to understand, and not a whole lot like mammalian communication.
The goal, here, is to have computers actually understand the language that they are using. It’s easy to teach a computer the rules of English grammar, for instance, and have it work with complex sentences that blow these little A.I. commands out of the water — but Microsoft Word doesn’t know why a sentence doesn’t make sense. If it sees the sentence, “I went to a happiness,” it knows that “happiness” isn’t the right kind of word to go with the verb “went.” It can point out the disagreement, without necessarily understanding that disagreement.
These meanings-based, “grounded” languages are almost certainly going to be necessary for computers to start to look at unfamiliar situations and produce novel, sensible strings of words that successfully improve the agent’s place in that environment. Some have asked how thought could arise without language, and how language could arise without thought — this study ought to show quite definitively how possible it is for basic mechanical language to arise without anything like a conscious brain.
As seen in the video below, the quest to let deep-learning neural networks develop human-like behaviors by human-like processes is not new. Some of these same researchers also worked on a robot that used child-inspired methods of trial and error to learn how to move.
This study hints at the even more interesting question, though: what could a novel machine language look like, with no human bias to direct it toward taking on a particularly human-like form? Would it naturally become much like our own for much the same reasons, or would computers find a totally different path of least resistance that better lines up with their unique abilities? Such an alternate path to language, when traveled by a robot psychology, could very well lead to syntax not unlike the code that underlies the agents themselves.
By capturing statistical patterns in large cor- pora, machine learning has enabled significant advances in natural language processing, includ- ing in machine translation, question answering, and sentiment analysis. However, for agents to intelligently interact with humans, simply cap- turing the statistical patterns is insufficient. In this paper we investigate if, and how, grounded compositional language can emerge as a means to achieve goals in multi-agent populations. To- wards this end, we propose a multi-agent learn- ing environment and learning methods that bring about emergence of a basic compositional lan- guage. This language is represented as streams of abstract discrete symbols uttered by agents over time, but nonetheless has a coherent structure that possesses a defined vocabulary and syntax. We also observe emergence of non-verbal com- munication such as pointing and guiding when language communication is unavailable.