Tech
Siri may not be 'racist,' but tech companies still must do better to address AI biases
A Stanford study shows, yet again, that speech recognition favors white Americans.
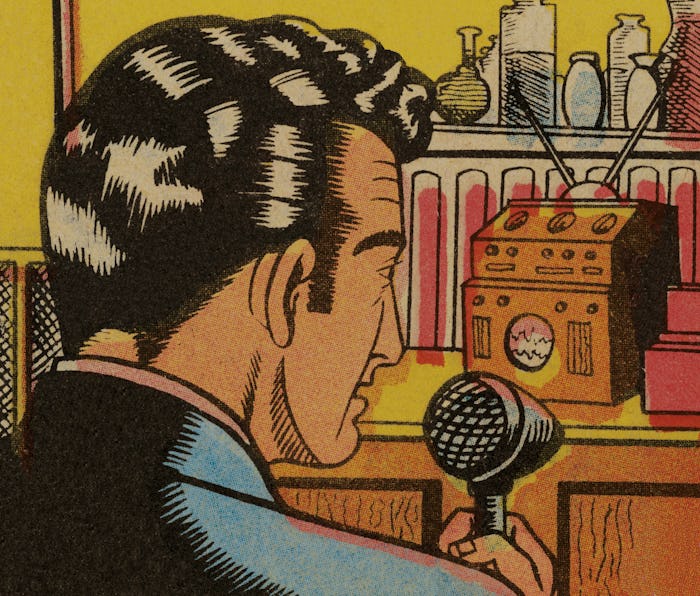
Speech recognition systems offered by the top names in tech still have a long way to go in addressing unconscious biases woven into their design. A new study conducted by a team at Stanford Engineering is the latest to highlight exactly that; according to the researchers, these systems disproportionately fail to understand black speakers.
It’s a problem we are, by now, well aware of — artificial intelligence is being developed and trained primarily with white people in mind, giving rise to systems that struggle to account for the rest of us. And that could have some pretty scary consequences.
In this case, researchers put technology from Apple, Google, Amazon, IBM, and Microsoft to the test, revealing staggering differences in error rates when comparing the results based on black versus white speakers. These systems misunderstood black speakers an average of 35 percent of the time, while the figure sits at just 19 percent for white speakers.
Yes yes, nuances, we know — In the study published this week to the journal Proceedings of the National Academy of Sciences, the Stanford team examined paid online speech recognition systems from Amazon, IBM, Google, and Microsoft and Apple’s free offering, for which they built a custom iOS app to run. These systems were fed roughly 2,000 speech samples from interviews recorded in the Corpus of Regional African American Language and Voices of California, providing examples of natural speech across black and white communities, respectively.
This kicked up some pretty blatant discrepancies in the systems’ ability to understand different groups of people. Among the takeaways: Black male speakers were the most misunderstood, particularly those who used what’s known as African American Vernacular English (a very clinical way of describing speech common in mostly-urban black communities).
It goes further than that, too, per the press release:
The researchers also ran additional tests to ascertain how often the five speech recognition technologies misinterpreted words so drastically that the transcriptions were practically useless. They tested thousands of speech samples, averaging 15 seconds in length, to count how often the technologies passed a threshold of botching at least half the words in each sample. This unacceptably high error rate occurred in over 20 percent of samples spoken by blacks, versus fewer than 2 percent of samples spoken by whites.
Shh, your racism is showing — While some outlets have chosen to highlight the above points as an opportunity to ‘debunk’ the findings with a shaky argument centered around slang, doing so misses — or intentionally dismisses — the whole point of the research. Which, in itself, just goes to show why these conversations are so necessary.
African American Vernacular English can’t just be brushed off as "slang." It’s not hyper-local, little-used colloquialisms unknown to the world beyond the confines of a few tucked-away regions; it’s the way people speak in many parts of this country… a lot of people, at that. So if a company is touting, or at least trying to develop, a system capable of understanding people in the broad sense, then it has to take these dialects into account. That also goes for other speech patterns common in non-white populations and even lower-income white communities that also get forgotten by the data.
"One should expect that U.S.-based companies would build products that serve all Americans," said lead author Allison Koenecke, a doctoral candidate in computational and mathematical engineering. "Right now, it seems that they’re not doing that for a whole segment of the population."
There’s more work to be done — The study, if anything, shows how important it is that tech companies diversify their datasets. If speech and facial recognition systems are trained using data that’s heavily comprised of white voices and white faces, their behavior in the real world will reflect that, to the detriment of minority groups.
While it’s worth noting that we don’t know if the studied systems are the same used in popular voice assistants (i.e. Siri, Alexa), as companies generally remain tight-lipped about special ingredients like that, it’s a comprehensive look into the ways biases arise in common tech. And if the companies themselves can’t do it right, they may need to enlist independent help.
"We can’t count on companies to regulate themselves," said Stanford computational engineering professor, Sharad Goel, who oversaw the work.
"That’s not what they’re set up to do. I can imagine that some might voluntarily commit to independent audits if there’s enough public pressure. But it may also be necessary for government agencies to impose more oversight. People have a right to know how well the technology that affects their lives really works."