Tech
Researchers trained AI to quickly determine disaster-related damage
All the useful first responder tool needs is an aerial image.
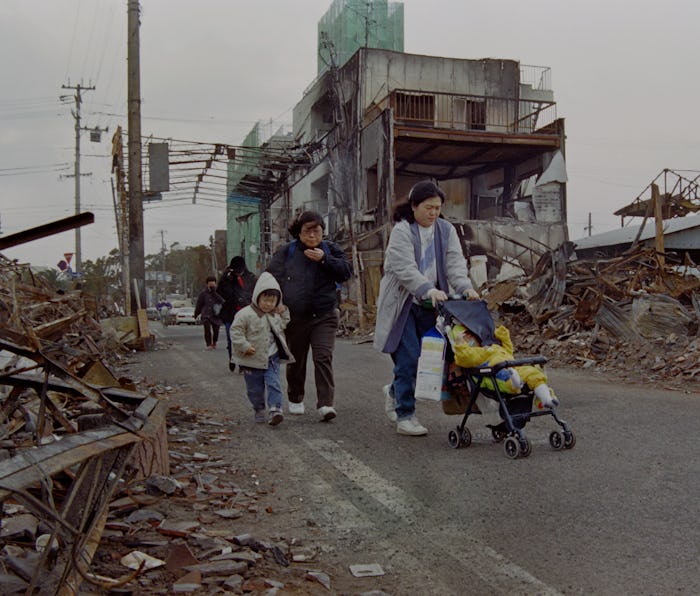
On Wednesday, researchers at Hiroshima University announced that they have taught an AI to analyze aerial images of post-disaster sights and determine where the most damage is. The ability to map the fallout of natural disasters and even acts of war is a vital tool first responders can leverage to target the places that need assistance the most. This is the latest in a line of research hoping to help them do just that.
The algorithm — A team from Hiroshima University's Graduate School of Advanced Science and Engineering used a convolutional neural network (CNN) to instantly evaluate photos. CNNs are modeled on how our own brains process images.
Led by Associate Professor Hiroyuki Miura, the team created a model that doesn’t need pre-disaster images to work. This CNN takes post-disaster images and classifies building damage as collapsed, non-collapsed, or blue-tarp-covered. The system draws from the seven damage scales the Architectural Institute of Japan used in the 2016 Kumamoto earthquakes.
The team believes that using ground data from structural engineers to train the algorithm has created a more reliable model. The CNN was tested on images taken after the September 2019 typhoon in Chiba, Japan. The damage classifications for about 94 percent of the buildings were accurate.
"We would like to develop a more robust damage identification method by learning more training data obtained from various disasters such as landslides, tsunami, and etcetera," Miura said in a statement.
AI you can get excited about — AI has become more of a specter than a helpful, futuristic tool lately, especially considering image recognition advancements have largely been involved surveillance-related facial recognition. Deep learning algorithms on this level, however, are an increasingly hot topic.
In August, Carnegie Mellon researchers unveiled ways to use drone imagery to get more detailed views of buildings post-disaster, filling in gaps aerial photos can’t. Their AI system will soon incorporate geolocation by leveraging Google Streetview. Google’s own researchers had already announced their own CNN in June, which uses high-resolution satellite images from before and after the event.
Following any disaster, but particularly very unpredictable ones like earthquakes, performing these kinds of assessments can take hours and divert personnel and other resources. The ability to achieve this in an instant could help save countless lives.