Culture
A classic AI training tool just got a serious privacy upgrade
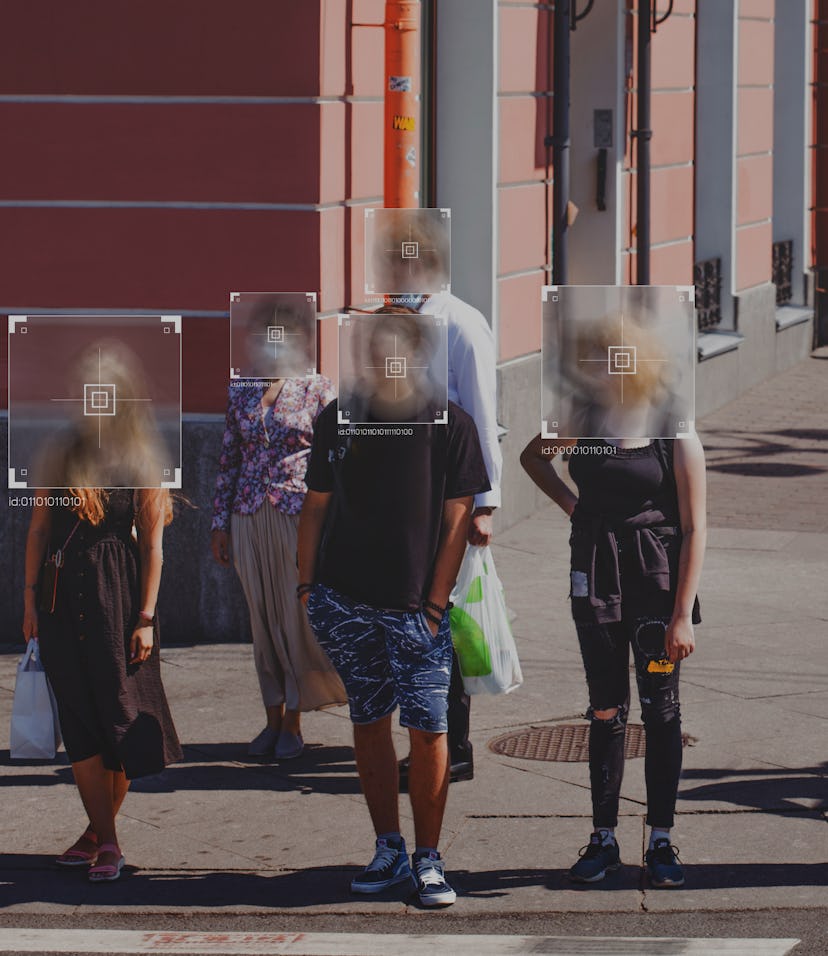
243K
How many of the almost 1.5 million images on ImageNet were affected.
ImageNet, a repository of 1.5 million images designed to train neural networks at object-recognition, originated in 2007. After several years of training different models, ImageNet’s artificial intelligence researchers managed to scrap millions of photos from the internet to create a comprehensive data set with remarkable improvement in 2012. ImageNet includes images of inanimate things as well as of people. When ImageNet was created, privacy was less of a concern than it is today. To that end, Wired reports the team that manages ImageNet has opted to blur the faces of the hundreds of thousands of people the repository includes.
Of the 1.5 million images, 243,198 images had people's faces in them. The decision to obscure these faces is a “privacy-aware” approach to object-recognition, Olga Russakovsky says. Russakovsky is an assistant professor at Princeton University and one of the people who administers ImageNet.
This isn't the first time that researchers critically evaluated visual data sets in ImageNet. Machine learning scientists Vinay Prabhu and Abeba Birhane published their findings in 2020, noting that people could be readily identified in the images. Additionally, Birhane and Prabhu have criticized the ImageNet team for “erasing” their scholarly contribution from the paper.
Why the obscuring now? — Initially, it was difficult to train algorithms to gauge and identify different objects, or people, in photos. Today, however, researchers have made gigantic leaps in the field, and algorithms are now able to accurately identify and categorize items in millions of photos with negligible error rates.
Of course, there’s controversy about privacy, too. Machine learning is now used in facial recognition technology and has elicited its fair share of criticism and even bans in certain American cities over the use of such programs because of biases, racism, and invasion of unsuspecting individuals’ privacy.
The issue of privacy is at the core of ImageNet's decision to blur faces, despite the potential unintended consequences. The team says the obscuring should encourage other researchers to create more privacy-aware practices in the field of computational image collection. They also say that blurring human faces doesn’t affect AI’s ability to classify inanimate objects.
Still, skeptics worry that this approach, no matter how well-intentioned, may eventually fail. They say that algorithms trained on data sets of blurred faces may struggle to classify clear faces in other collections. This concern, however, sounds less urgent than securing people’s privacy, which is rapidly being eroded both on and offline.